(Invited) A Framework for in-Line Analysis and Quantification of Battery Aging Mechanisms Using Electrochemical Signatures Under Fast-Cycling Conditions
Meeting abstracts(2023)
摘要
Rapid, in-situ Li-ion battery state-of-health quantification is challenging. Li-ion battery aging modes can vary significantly with chemistry, operating conditions, cycling demands, electrode design, and operation history. In literature, Li-ion battery aging modes are typically lumped into categories such as loss-of-lithium-inventory (LLI), loss-of-active-material (LAM) in either electrode, and/or impedance rise. Importantly, as a cell ages, optimal and safe operating conditions need to be adapted to account for battery degradation. The present research describes a framework for identifying battery aging modes in-operando using fast-rate voltage charge/discharge responses. Once developed, the model can be used to quickly identify the battery aged state, without relying on slow reference performance tests. The framework first develops a physically based Li-ion battery model that captures aging effects (i.e., LLI and LAM). Once the aging model is calibrated to known battery performance at aged states, the model is used to generate synthetic voltage responses under a variety of aged conditions. These synthetic responses are then used to train a machine-learning model. In the end, the machine-learning model is used, in conjunction with experimentally measured voltage profiles, to identify cycle-by-cycle battery state-of-health variables of real cells in operando. The framework is used to identify battery state-of-health for two different electrode loadings and for cells charged a variety of fast-rate conditions (i.e., 1C, 4C, 6C, and 9C). Figure 1
更多查看译文
关键词
battery aging mechanisms,electrochemical signatures,in-line,fast-cycling
AI 理解论文
溯源树
样例
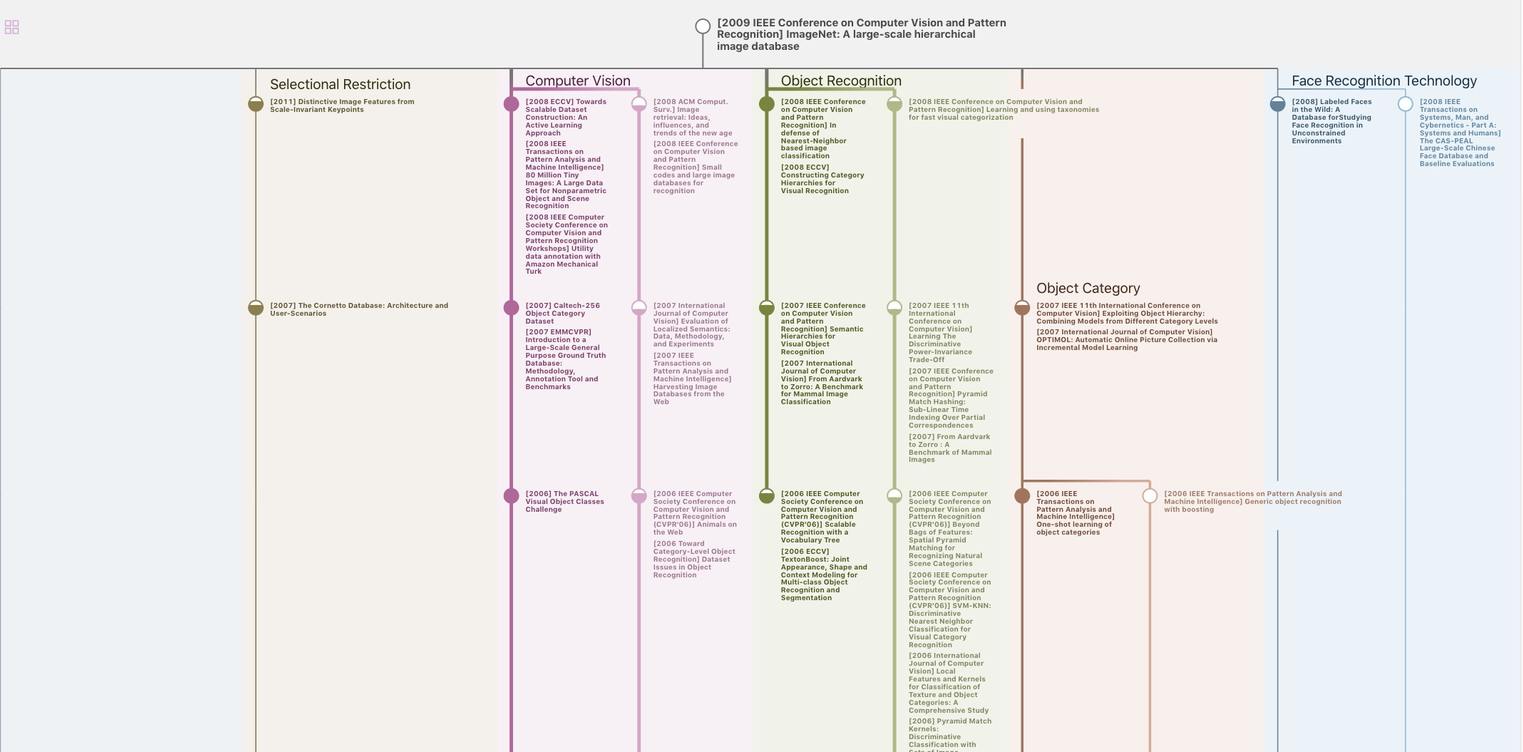
生成溯源树,研究论文发展脉络
Chat Paper
正在生成论文摘要