Incremental Reinforcement Learning via Performance Evaluation and Policy Perturbation.
IEEE Trans. Artif. Intell.(2024)
摘要
Rapid adaptation to the environment is the long-term task of reinforcement learning. However, reinforcement learning faces great challenges in dynamic environments, especially with continuous state-action spaces. In this paper, we propose a systematic Incremental Reinforcement Learning method via Performance Evaluation and Policy Perturbation (IRL-PEPP) to improve the adaptability of reinforcement learning algorithms in dynamic environments with continuous state-action spaces, which mainly includes three parts, i.e., performance evaluation, policy perturbation and importance weighting. Firstly, in performance evaluation, we apply the learned optimal policy to sample a few episodes in the original environment and use these samples to evaluate the policy applicability in the new environment. Then, in policy perturbation, the policy is perturbed according to the policy applicability to balance the trade-off between exploration and exploitation in the new environment. Finally, importance weighting is applied to weight the information to speed up the adjustment process of the policy. Experimental results demonstrate the feasibility and efficiency of the proposed IRL-PEPP method for continuous control tasks in comparison to the existing state-of-the-art methods.
更多查看译文
关键词
Continuous state-action spaces,dynamic environments,incremental reinforcement learning,performance evaluation,policy perturbation
AI 理解论文
溯源树
样例
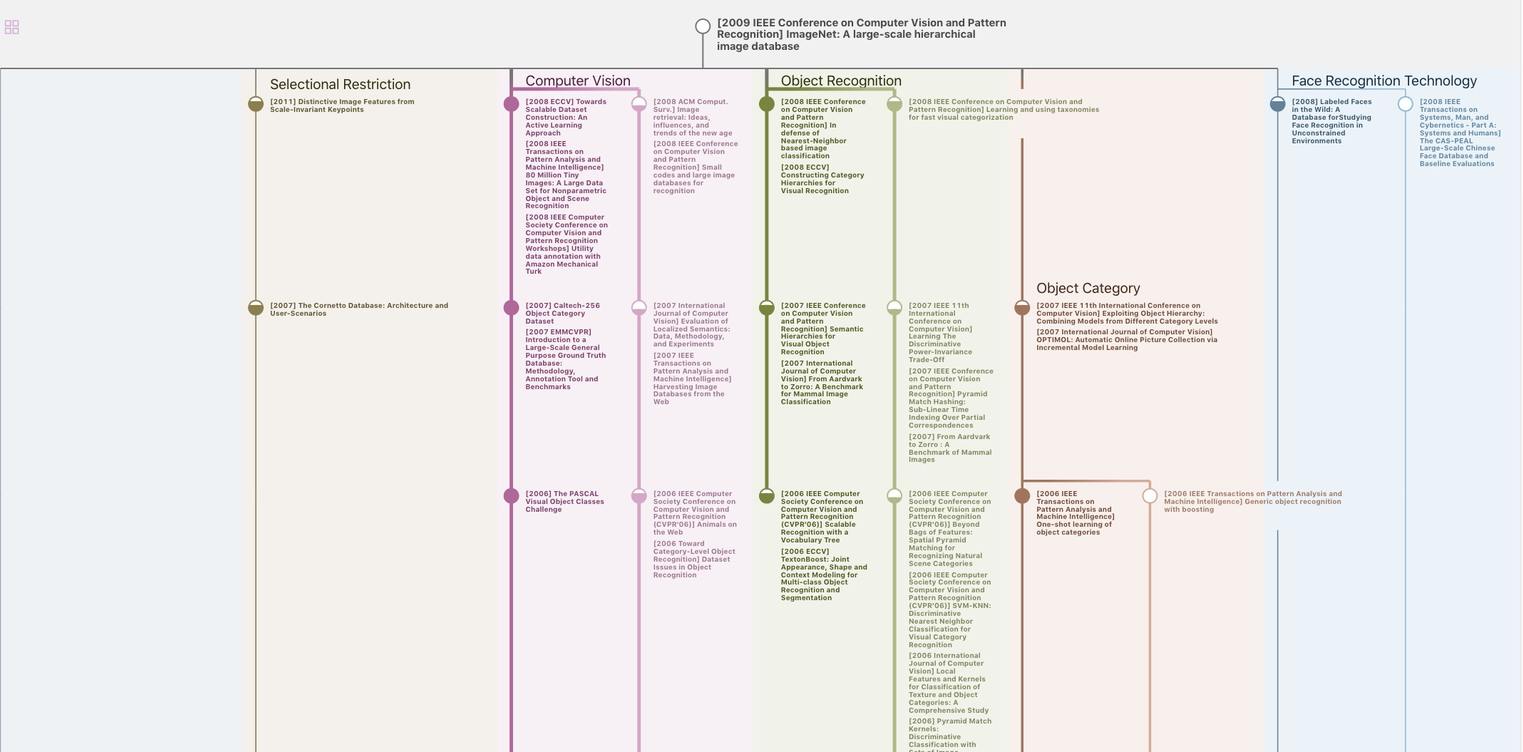
生成溯源树,研究论文发展脉络
Chat Paper
正在生成论文摘要