Predicting Vegetation Stratum Occupancy from Airborne LiDAR Data with Deep Learning
arXiv (Cornell University)(2022)
摘要
We propose a new deep learning-based method for estimating the occupancy of vegetation strata from airborne 3D LiDAR point clouds. Our model predicts rasterized occupancy maps for three vegetation strata corresponding to lower, medium, and higher cover. Our weakly-supervised training scheme allows our network to only be supervised with vegetation occupancy values aggregated over cylindrical plots containing thousands of points. Such ground truth is easier to produce than pixel-wise or point-wise annotations. Our method outperforms handcrafted and deep learning baselines in terms of precision by up to 30%, while simultaneously providing visual and interpretable predictions. We provide an open-source implementation along with a dataset of 199 agricultural plots to train and evaluate weakly supervised occupancy regression algorithms.
更多查看译文
关键词
airborne lidar data,vegetation stratum occupancy,deep learning
AI 理解论文
溯源树
样例
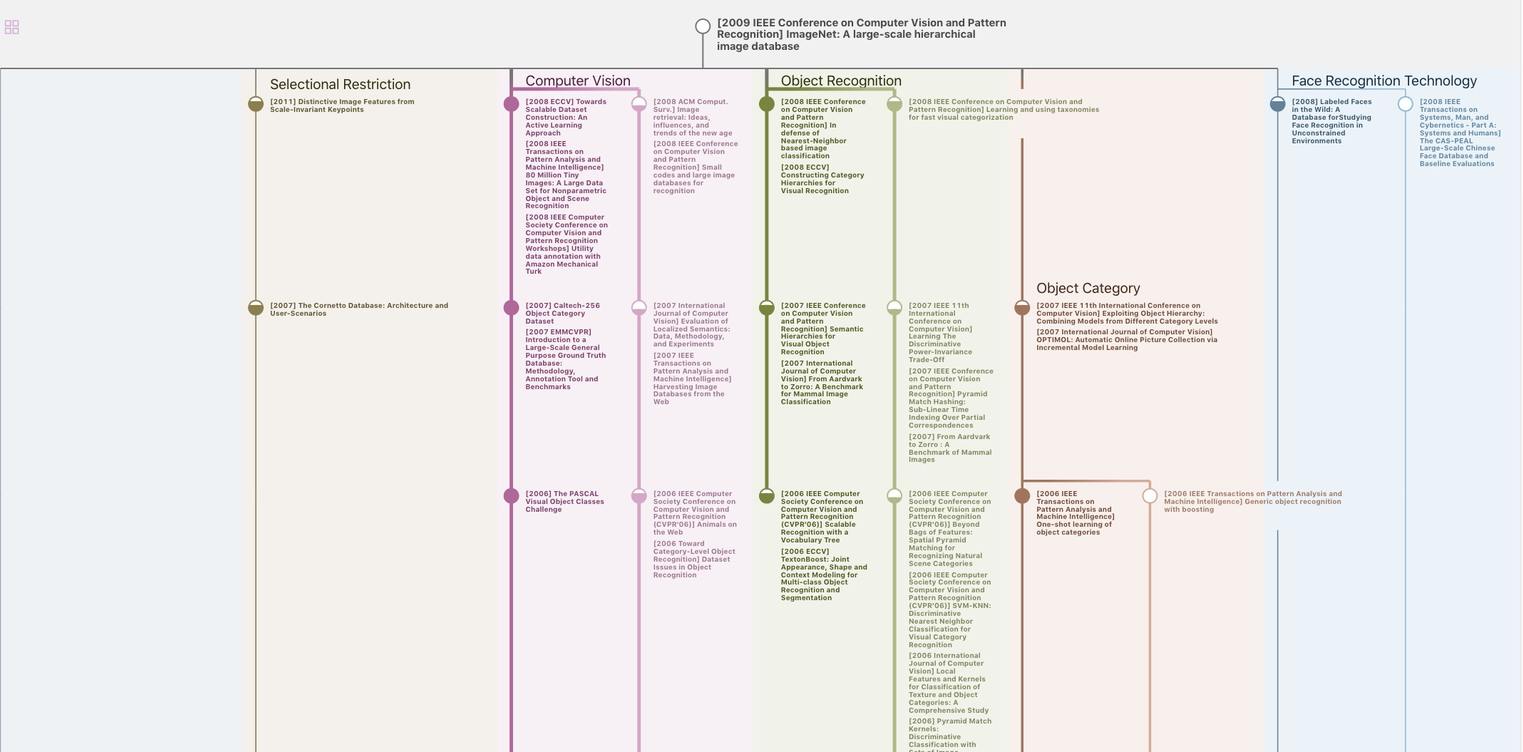
生成溯源树,研究论文发展脉络
Chat Paper
正在生成论文摘要