A Simple and Efficient Pipeline to Build an End-to-End Spatial-Temporal Action Detector
arXiv (Cornell University)(2022)
摘要
Spatial-temporal action detection is a vital part of video understanding. Current spatial-temporal action detection methods mostly use an object detector to obtain person candidates and classify these person candidates into different action categories. So-called two-stage methods are heavy and hard to apply in real-world applications. Some existing methods build one-stage pipelines, But a large performance drop exists with the vanilla one-stage pipeline and extra classification modules are needed to achieve comparable performance. In this paper, we explore a simple and effective pipeline to build a strong one-stage spatial-temporal action detector. The pipeline is composed by two parts: one is a simple end-to-end spatial-temporal action detector. The proposed end-to-end detector has minor architecture changes to current proposal-based detectors and does not add extra action classification modules. The other part is a novel labeling strategy to utilize unlabeled frames in sparse annotated data. We named our model as SE-STAD. The proposed SE-STAD achieves around 2% mAP boost and around 80% FLOPs reduction. Our code will be released at https://github.com/4paradigm-CV/SE-STAD.
更多查看译文
关键词
action,end-to-end,spatial-temporal
AI 理解论文
溯源树
样例
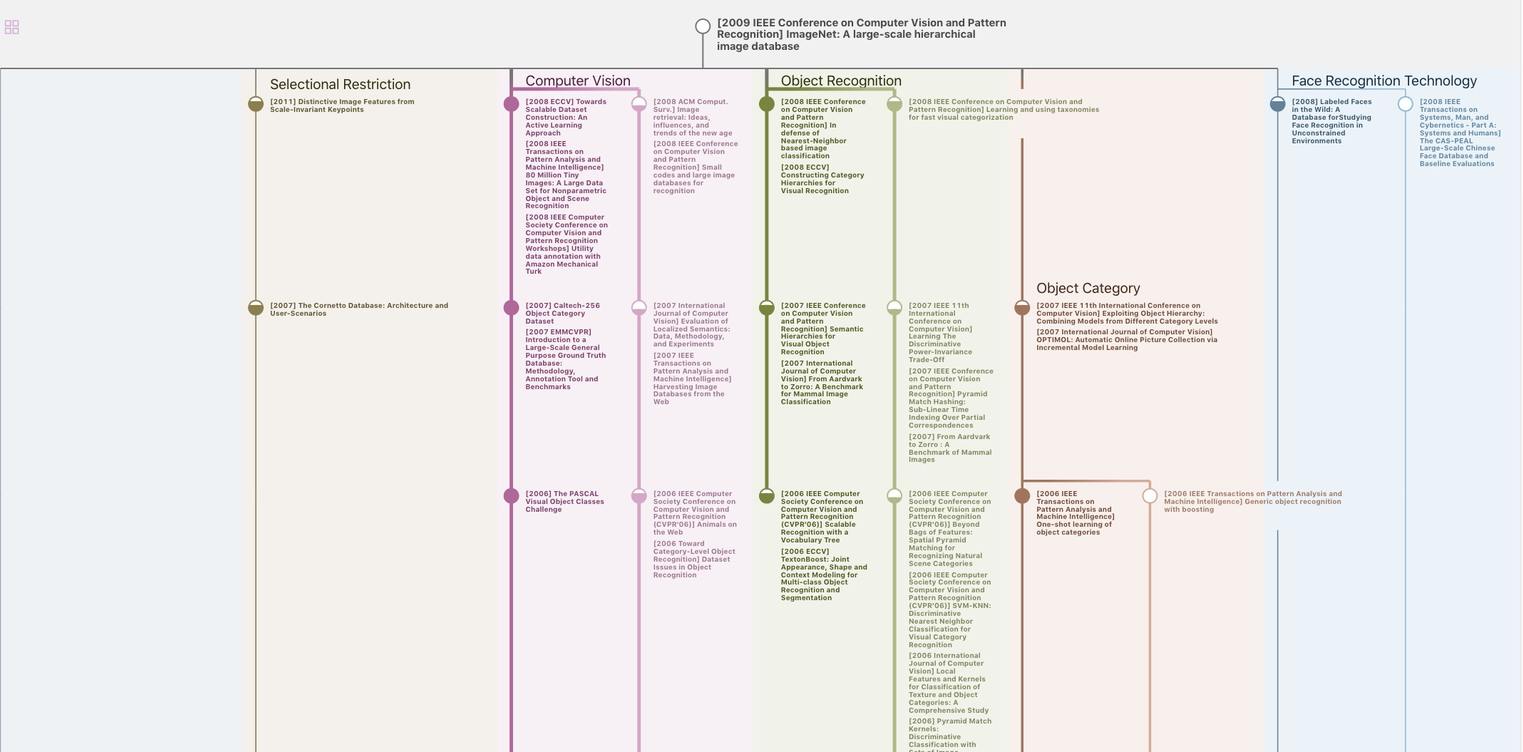
生成溯源树,研究论文发展脉络
Chat Paper
正在生成论文摘要