Minimum Efforts to Build an End-to-End Spatial-Temporal Action Detector
arXiv (Cornell University)(2022)
摘要
Spatial-temporal action detection is a vital part of video understanding. Current spatial-temporal action detection methods will first use an object detector to obtain person candidate proposals. Then, the model will classify the person candidates into different action categories. So-called two-stage methods are heavy and hard to apply in real-world applications. Some existing methods use a unified model structure, But they perform badly with the vanilla model and often need extra modules to boost the performance. In this paper, we explore the strategy to build an end-to-end spatial-temporal action detector with minimal modifications. To this end, we propose a new method named ME-STAD, which solves the spatial-temporal action detection problem in an end-to-end manner. Besides the model design, we propose a novel labeling strategy to deal with sparse annotations in spatial-temporal datasets. The proposed ME-STAD achieves better results (2.2% mAP boost) than original two-stage detectors and around 80% FLOPs reduction. Moreover, our proposed ME-STAD only has minimum modifications with previous methods and does not require extra components. Our code will be made public.
更多查看译文
关键词
action,detector,end-to-end,spatial-temporal
AI 理解论文
溯源树
样例
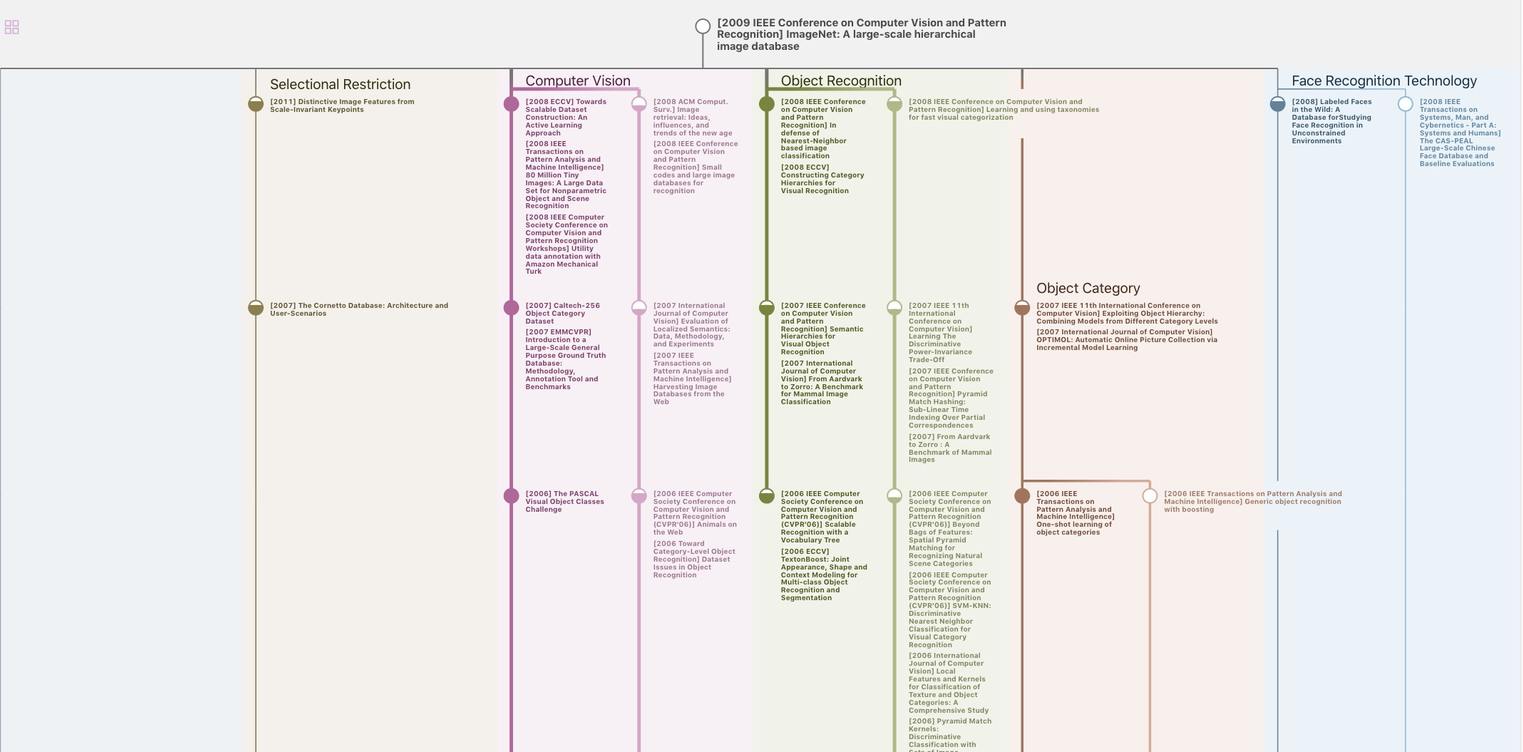
生成溯源树,研究论文发展脉络
Chat Paper
正在生成论文摘要