Simultaneous estimation and group identification for network vector autoregressive model with heterogeneous nodes
Journal of Econometrics(2023)
摘要
Individuals or companies in a large social or financial network often display rather heterogeneous behaviors for various reasons. In this work, we propose a network vector autoregressive model with a latent group structure to model heterogeneous dynamic patterns observed from network nodes, for which group-wise network effects and time-invariant fixed-effects can be naturally incorporated. In our framework, the model parameters and network node memberships can be simultaneously estimated by minimizing a least-squares type objective function. In particular, our theoretical investigation allows the number of latent groups G to be over-specified when achieving the estimation consistency of the model parameters and group memberships, which significantly improves the robustness of the proposed approach. When G is correctly specified, valid statistical inference can be made for model parameters based on the asymptotic normality of the estimators. A data-driven criterion is developed to consistently identify the true group number for practical use. Extensive simulation studies and two real data examples are used to demonstrate the effectiveness of the proposed methodology.
更多查看译文
关键词
Heterogeneity,Latent group structure,Network autoregressive model,Network time series
AI 理解论文
溯源树
样例
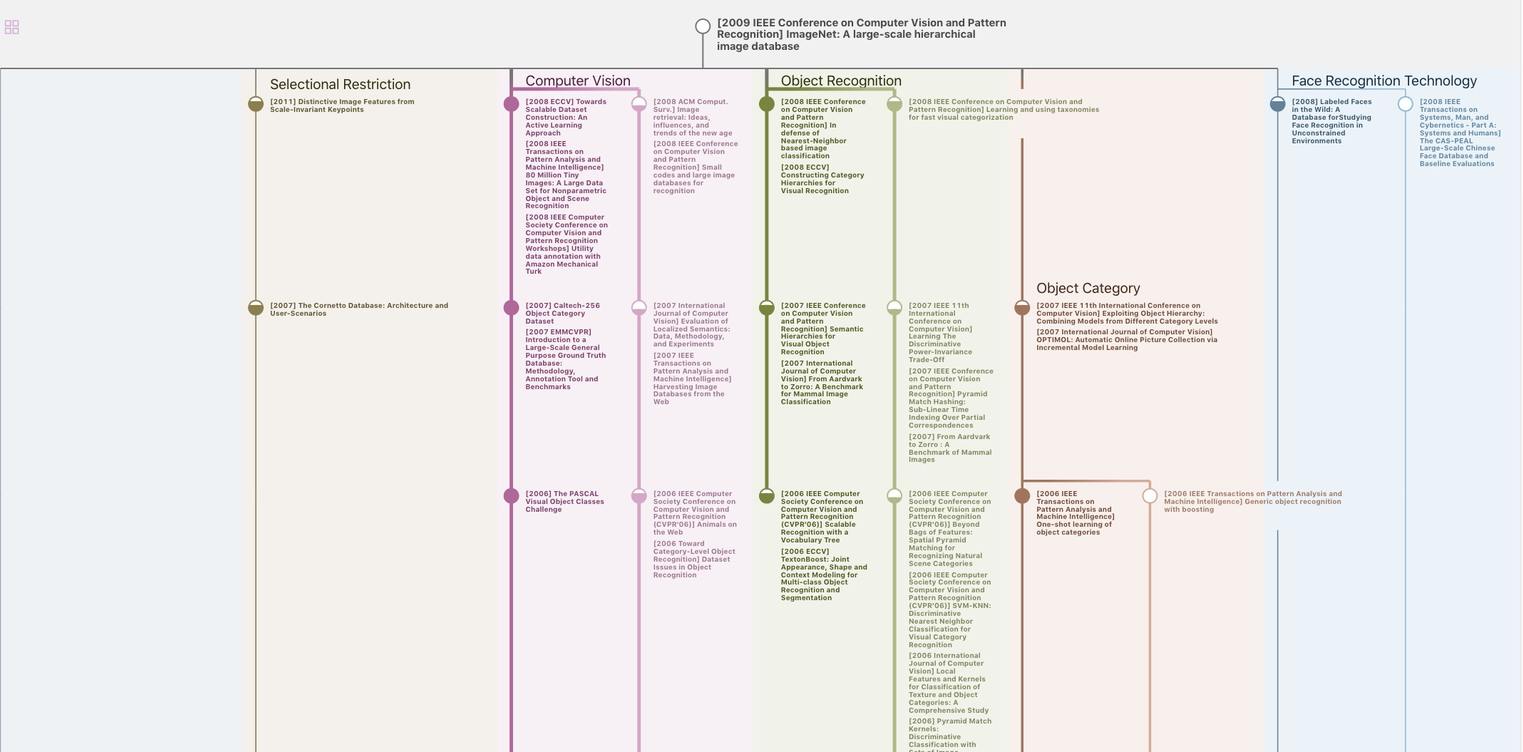
生成溯源树,研究论文发展脉络
Chat Paper
正在生成论文摘要