Deep Direct Discriminative Decoders for High-dimensional Time-series Data Analysis
arXiv (Cornell University)(2023)
摘要
The state-space models (SSMs) are widely utilized in the analysis of time-series data. SSMs rely on an explicit definition of the state and observation processes. Characterizing these processes is not always easy and becomes a modeling challenge when the dimension of observed data grows or the observed data distribution deviates from the normal distribution. Here, we propose a new formulation of SSM for high-dimensional observation processes with a heavy-tailed distribution. We call this solution the deep direct discriminative process (D4). The D4 brings deep neural networks’ expressiveness and scalability to the SSM formulation letting us build a novel solution that efficiently estimates the underlying state processes through high-dimensional observation signal.We demonstrate the D4 solutions in simulated and real data such as Lorenz attractors, Langevin dynamics, random walk dynamics, and rat hippocampus spiking neural data and show that the D4’s performance precedes traditional SSMs and RNNs. The D4 can be applied to a broader class of time-series data where the connection between high-dimensional observation and the underlying latent process is hard to characterize.
更多查看译文
关键词
time-series time-series,high-dimensional
AI 理解论文
溯源树
样例
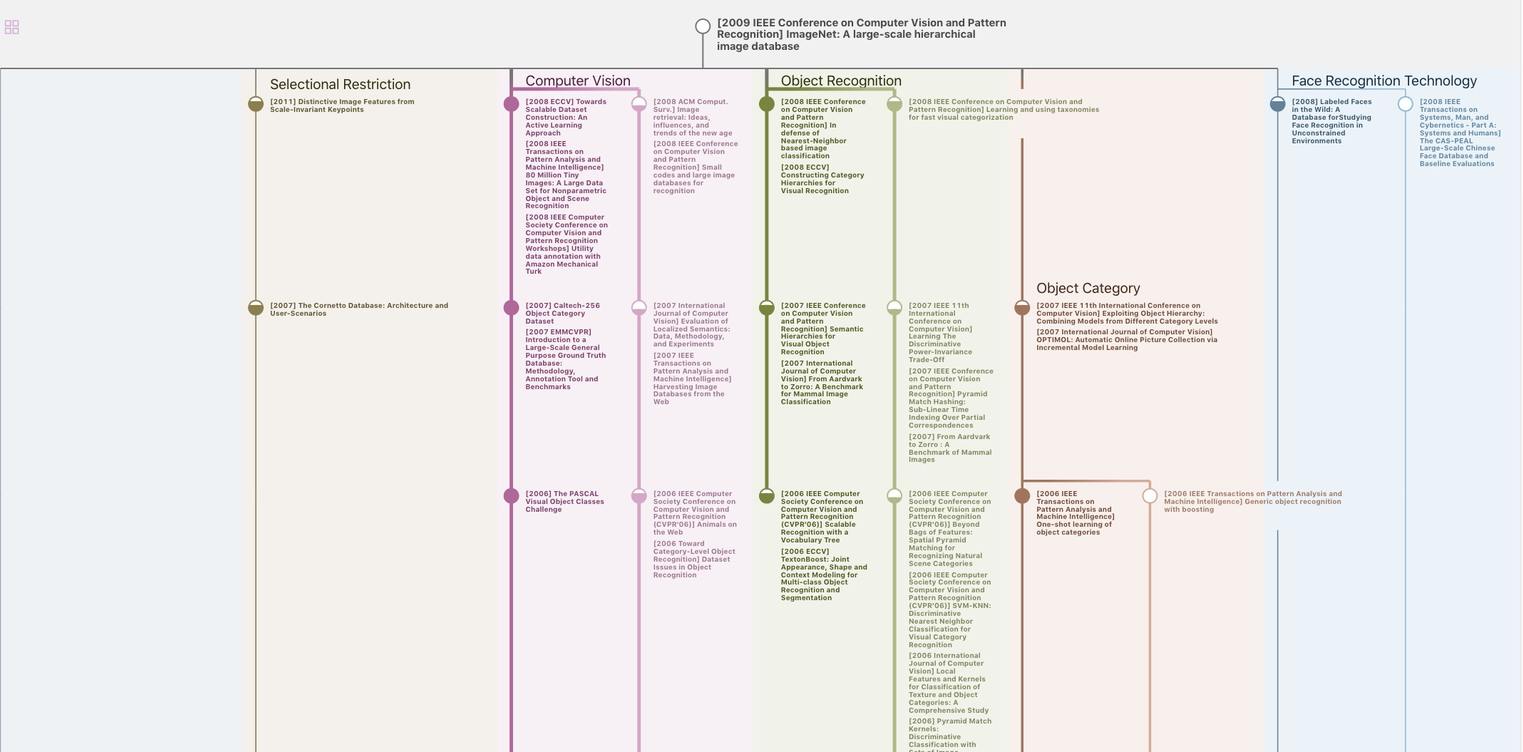
生成溯源树,研究论文发展脉络
Chat Paper
正在生成论文摘要