1287 Radiomics and delta-radiomics signatures to predict response and survival in patient with non-small cell lung cancer treated with immune checkpoint inhibitors
Regular and Young Investigator Award Abstracts(2022)
摘要
Background
Accurate and early selection of patients with advanced non-small cell lung cancer (NSCLC) who would benefit from immunotherapy is of the utmost clinical importance. The aim of our study was to determine the potential role of CT-based radiomics and delta-radiomics signatures in predicting treatment response and survival in patients with advanced NSCLC treated with programmed death-1 (PD-1) or programmed death ligand-1 (PD-L1) inhibitors. Moreover, we compared the results obtained with both signatures to early RECIST v1.1 assessment (first follow up visit).
Methods
In this retrospective multi-centric study, we included 188 patients with NSCLC treated with PD-1/PD-L1 inhibitors who underwent a pre-treatment and at least one follow-up CT scans. The training dataset was composed of 146 patients and the external validation dataset of 42 patients. All the lesions, both target and non-target, according to RECIST v1.1 criteria, were manually segmented by experienced reader. Radiomics analysis was performed on both single and multiple target lesions per patient. A delta-radiomics analysis was also conducted on a subset of 160 patients who underwent a follow-up CT after 2 to 4 treatment cycles. Radiomic features were selected by the Minimum Redundancy Maximum Relevance method. Linear and Random Forest (RF) models were tested to predict response at 6 months and overall survival (OS). Performances of the models were expressed in term of Area Under the Curve (AUC) and survival prediction with Cox concordance index.
Results
The baseline CT radiomics signatures did not show any significant results for prediction of treatment response or survival. The RF delta-radiomics model showed the best performance for treatment response prediction with an AUC of 0.81 (95% CI: 0.66-0.95). The GLM delta-radiomics model was the most accurate at predicting survival with a concordance index of 0.68 (95% CI: 0.56-0.80) (p=0.02). The comparison between the delta radiomics signatures and early RECIST v1.1 assessment showed a clear improvement in survival prediction with an AUC of 0.66 (95% CI:0.54-0.78) for early RECIST against an AUC of 0.77 (95% CI: 0.61-0.93) and 0.81 (GLM and RF signatures respectively) for delta radiomics signature and a concordance index of 0.56 (95% CI: 0.47-0.65) on the validation dataset.
Conclusions
Our delta radiomics models were able to early identify patients with advanced NSCLC who were more likely to benefit from immunotherapy.
Ethics Approval
This study received approval from institutional review board of University Hospital of Liège, and the need for informed consent was waived based on its retrospective design.
更多查看译文
关键词
lung cancer,delta-radiomics,non-small
AI 理解论文
溯源树
样例
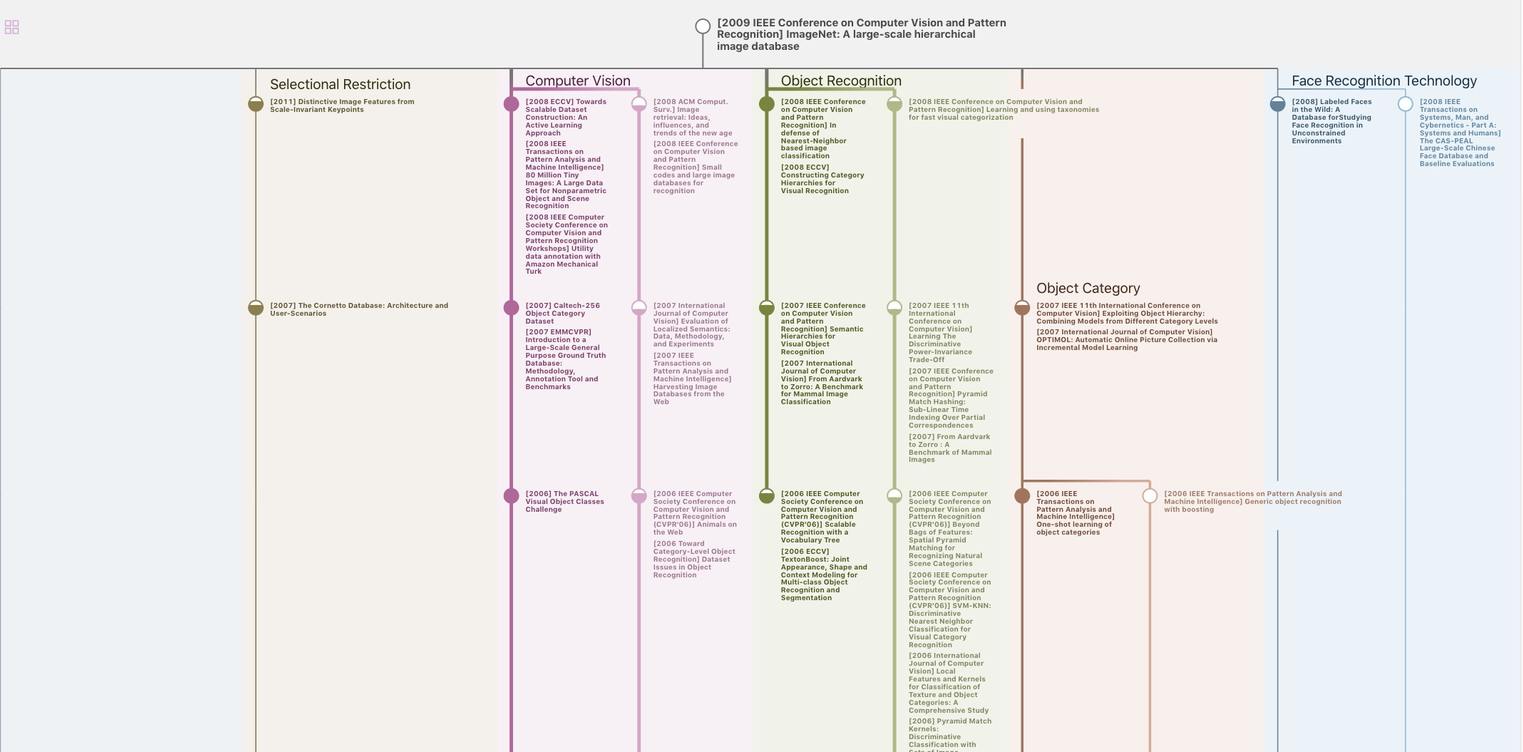
生成溯源树,研究论文发展脉络
Chat Paper
正在生成论文摘要