Credit Card Fraud Detection Using Logistic Regression and Synthetic Minority Oversampling Technique (SMOTE) Approach.
International journal of computer and communication technology(2022)
Abstract
Financial fraud is a serious threat that is expanding effects on the financial sector. The use of credit cards is growing as digitization and internet transactions advance daily. The most common issues in today's culture are credit card scams. This kind of fraud typically happens when someone uses someone else's credit card details. Credit card fraud detection uses transaction data attributes to identify credit card fraud, which can save significant financial losses and affluence the burden on the police. The detection of credit card fraud has three difficulties: uneven data, an abundance of unseen variables, and the selection of an appropriate threshold to improve the models' reliability. This study employs a modified Logistic Regression (LR) model to detect credit card fraud in order to get over the preceding difficulties. The dataset sampling strategy, variable choice, and detection methods employed all have a significant impact on the effectiveness of fraud detection in credit card transactions. The effectiveness of naive bayes, k-nearest neighbour, and logistic regression on highly skewed credit card fraud data is examined in this research. The accuracy of the logistic regression technique will be closer to 0.98%; with this accuracy, frauds may be easily detected. The fact that LR receives the highest classifier score illustrates how well LR predicts credit card theft.
MoreTranslated text
Key words
synthetic minority oversampling technique,smote,logistic regression
AI Read Science
Must-Reading Tree
Example
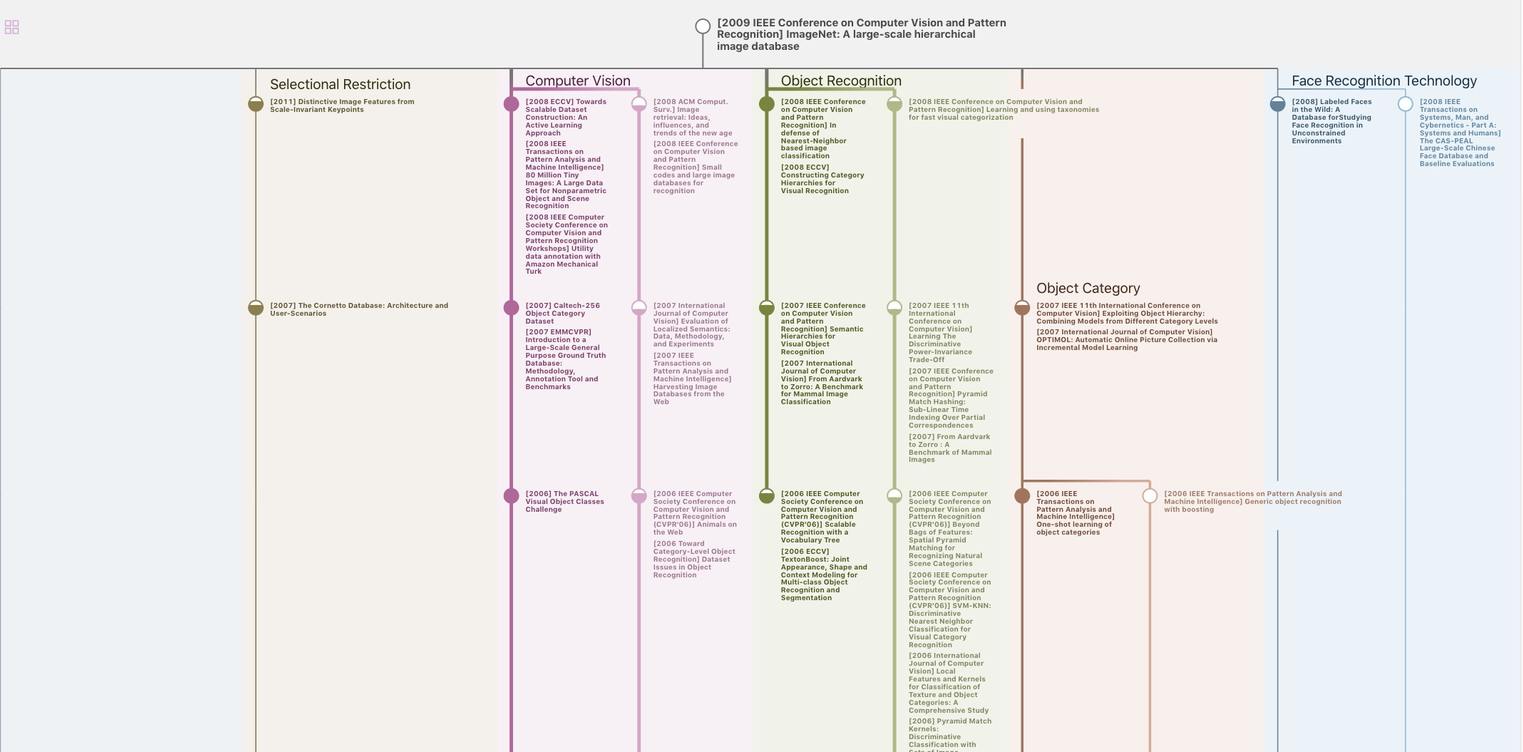
Generate MRT to find the research sequence of this paper
Chat Paper
Summary is being generated by the instructions you defined