Stacking Designs: Designing Multifidelity Computer Experiments with Target Predictive Accuracy
arXiv (Cornell University)(2024)
摘要
In an era where scientific experiments can be very costly, multifidelity emulators provide a useful tool for cost-efficient predictive scientific computing. For scientific applications, the experimenter is often limited by a tight computational budget, and thus wishes to (i) maximize predictive power of the multifidelity emulator via a careful design of experiments, and (ii) ensure this model achieves a desired error tolerance with some notion of confidence. Existing design methods, however, do not jointly tackle objectives (i) and (ii). We propose a novel stacking design approach that addresses both goals. A multilevel reproducing kernel Hilbert space (RKHS) interpolator is first introduced to build the emulator, under which our stacking design provides a sequential approach for designing multifidelity runs such that a desired prediction error of epsilon > 0 is met under regularity assumptions. We then prove a novel cost complexity theorem that, under this multilevel interpolator, establishes a bound on the computation cost (for training data simulation) needed to achieve a prediction bound of epsilon . This result provides novel insights on conditions under which the proposed multifidelity approach improves upon a conventional RKHS interpolator which relies on a single fidelity level. Finally, we demonstrate the effectiveness of stacking designs in a suite of simulation experiments and an application to finite element analysis.
更多查看译文
关键词
computer experiments,experimental design,finite element analysis,Gaussian process modeling,multilevel modeling,uncertainty quantification
AI 理解论文
溯源树
样例
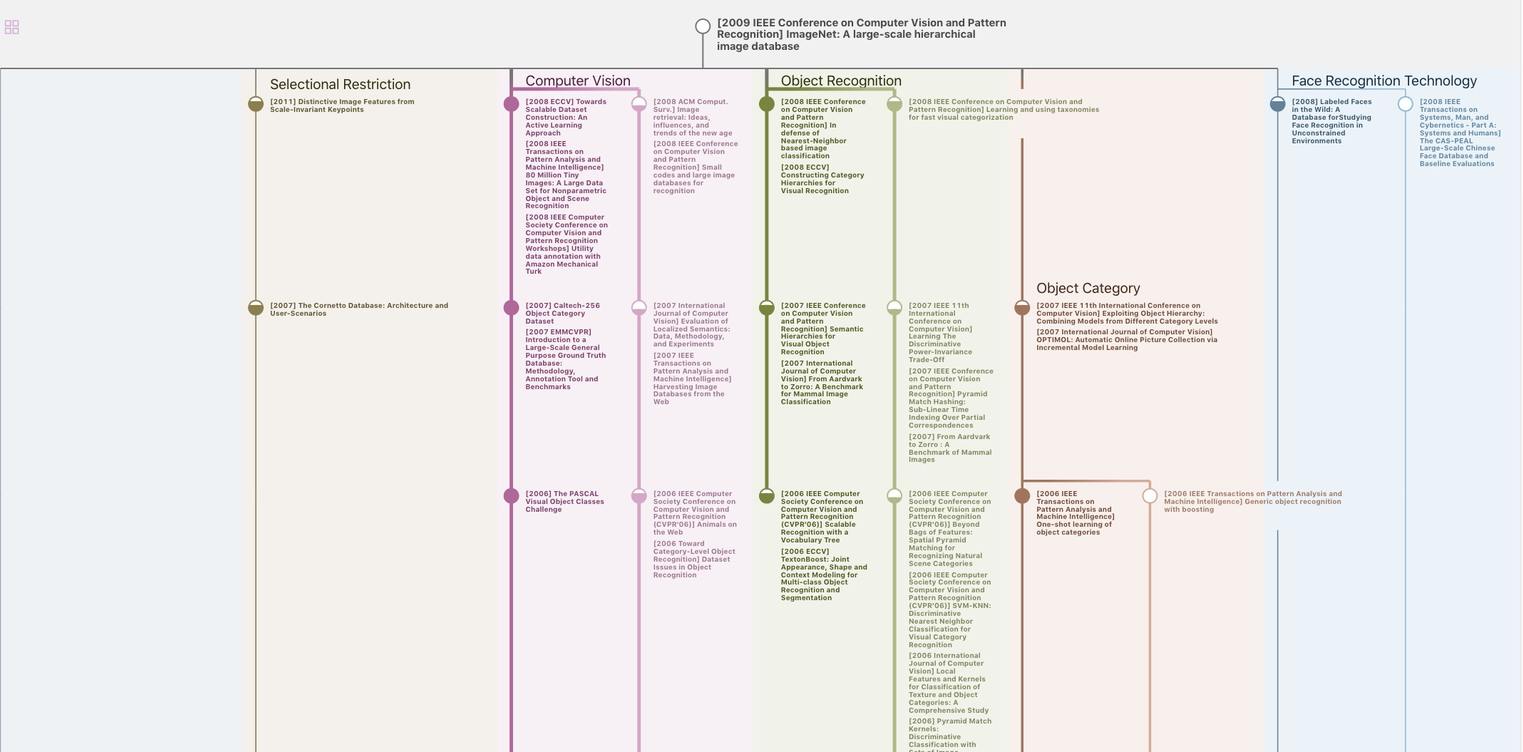
生成溯源树,研究论文发展脉络
Chat Paper
正在生成论文摘要