Deep Learning-Based Dose Prediction for Automated, Individualized Quality Assurance of Head and Neck Radiotherapy Plans
arXiv (Cornell University)(2022)
摘要
Purpose: This study aimed to use deep learning-based dose prediction to assess head and neck (HN) plan quality and identify sub-optimal plans. Methods: A total of 245 VMAT HN plans were created using RapidPlan knowledge-based planning (KBP). A subset of 112 high-quality plans was selected under the supervision of an HN radiation oncologist. We trained a 3D Dense Dilated U-Net architecture to predict 3D dose distributions using three-fold cross-validation on 90 plans. Model inputs included CT images, target prescriptions, and contours for targets and organs at risk (OARs). The model's performance was assessed on the remaining 22 test plans. We then tested the application of the dose prediction model for automated review of plan quality. Dose distributions were predicted on 14 clinical plans. The predicted versus clinical OAR dose metrics were compared to flag OARs with sub-optimal normal tissue sparing using a 2Gy dose difference or 3% dose-volume threshold. OAR flags were compared to manual flags by three HN radiation oncologists. Results: The predicted dose distributions were of comparable quality to the KBP plans. The differences between the predicted and KBP-planned D1%, D95%, and D99% across the targets were within -2.53%(SD=1.34%), -0.42%(SD=1.27%), and -0.12%(SD=1.97%), respectively, while the OAR mean and maximum doses were within -0.33Gy(SD=1.40Gy) and -0.96Gy(SD=2.08Gy). For the plan quality assessment study, radiation oncologists flagged 47 OARs for possible plan improvement. There was high inter-physician variability; 83% of physician-flagged OARs were flagged by only one of three physicians. The comparative dose prediction model flagged 63 OARs, including 30/47 physician-flagged OARs. Conclusion: Deep learning can predict high-quality dose distributions, which can be used as comparative dose distributions for automated, individualized assessment of HN plan quality.
更多查看译文
关键词
radiotherapy,learning-based
AI 理解论文
溯源树
样例
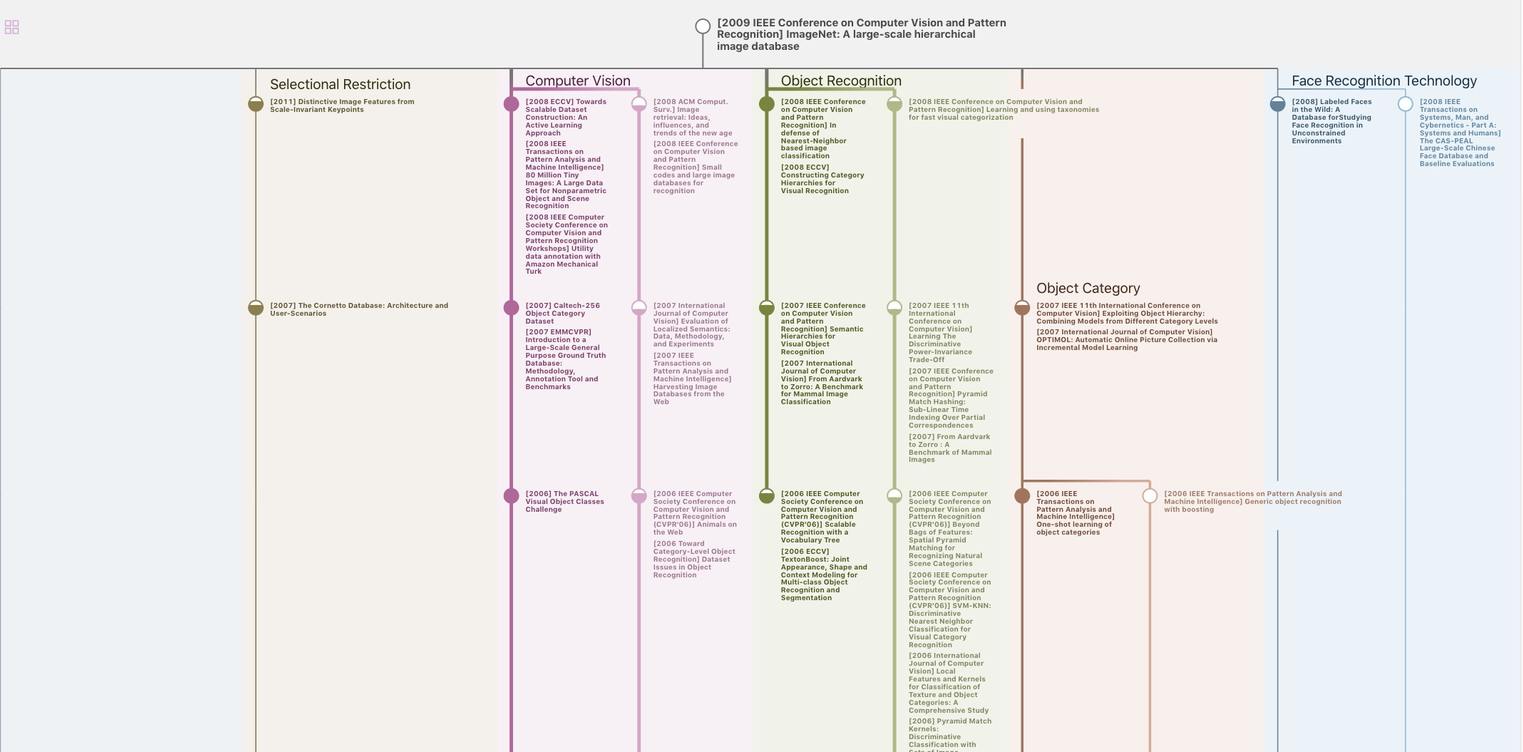
生成溯源树,研究论文发展脉络
Chat Paper
正在生成论文摘要