Untrained, physics-informed neural networks for structured illumination microscopy
arXiv (Cornell University)(2022)
Abstract
In recent years there has been great interest in using deep neural networks (DNN) for super-resolution image reconstruction including for structured illumination microscopy (SIM). While these methods have shown very promising results, they all rely on data-driven, supervised training strategies that need a large number of ground truth images, which is experimentally difficult to realize. For SIM imaging, there exists a need for a flexible, general, and open-source reconstruction method that can be readily adapted to different forms of structured illumination. We demonstrate that we can combine a deep neural network with the forward model of the structured illumination process to reconstruct sub-diffraction images without training data. The resulting physics-informed neural network (PINN) can be optimized on a single set of diffraction limited sub-images and thus doesn't require any training set. We show with simulated and experimental data that this PINN can be applied to a wide variety of SIM methods by simply changing the known illumination patterns used in the loss function and can achieve resolution improvements that match well with theoretical expectations.
MoreTranslated text
Key words
structured illumination
AI Read Science
Must-Reading Tree
Example
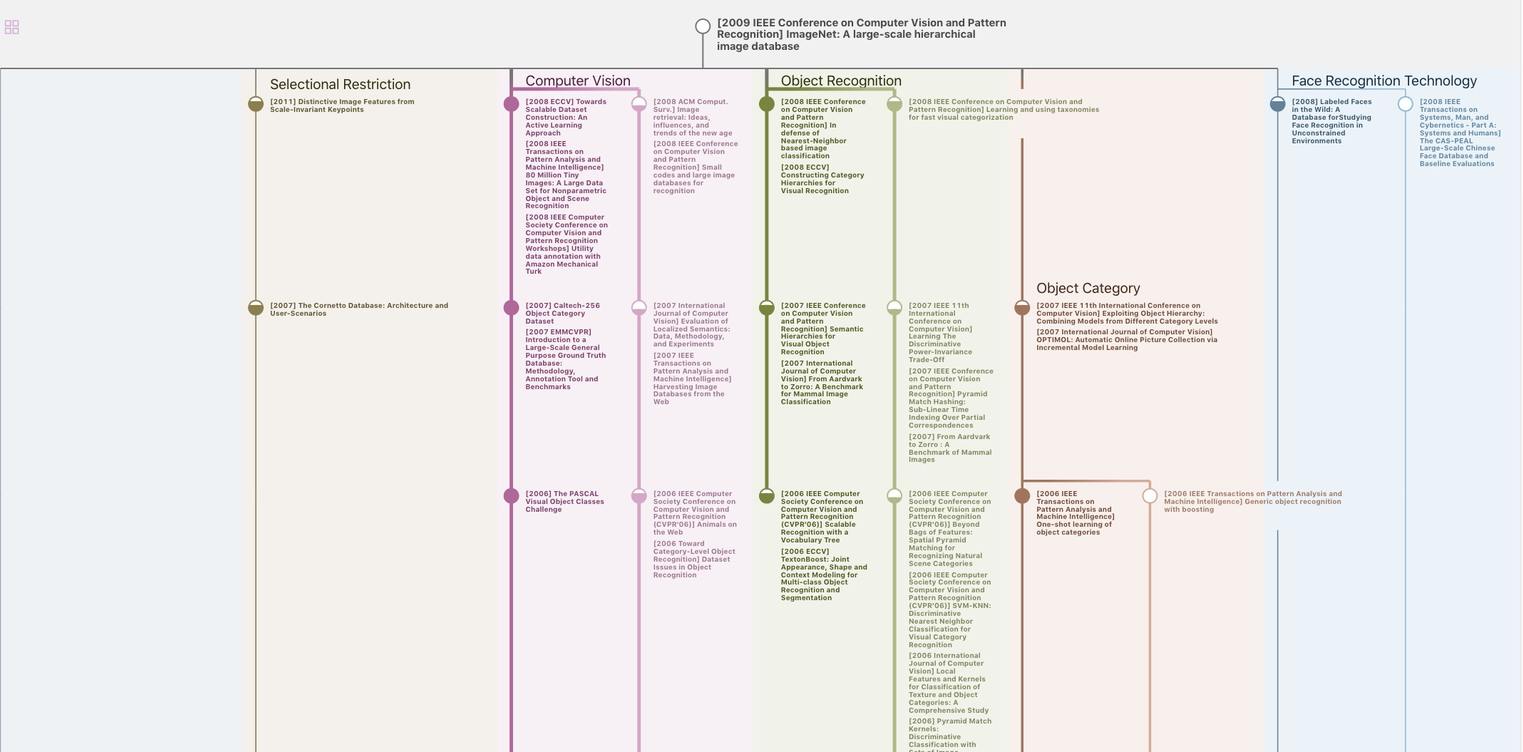
Generate MRT to find the research sequence of this paper
Chat Paper
Summary is being generated by the instructions you defined