A pruning algorithm with relaxed conditions for high-order neural networks based on smoothing group L 1 / 2 regularization and adaptive momentum
Knowledge Based Systems(2022)
摘要
To enhance the sparseness of the network, improve its generalization ability and accelerate its training, we propose a novel pruning approach for sigma-pi-sigma neural network (SPSNN) under the relaxed condition by adding smoothing group L 1 / 2 regularization and adaptive momentum. The main strength of this method is that it can prune both the redundant nodes between groups in the network, and also the redundant weights of the non-redundant nodes within the group, so as to achieve the sparseness of the network. Another strength is that the non-smooth absolute value function in the traditional L 1 / 2 regularization method is replaced by a smooth function. This reduces the oscillations of learning and enables us to more effectively prove the convergence of the proposed algorithm. Finally, the numerical simulation results demonstrate the effectiveness of the proposed algorithm.
更多查看译文
关键词
pruning algorithm,smoothing group,regularization,neural networks,high-order
AI 理解论文
溯源树
样例
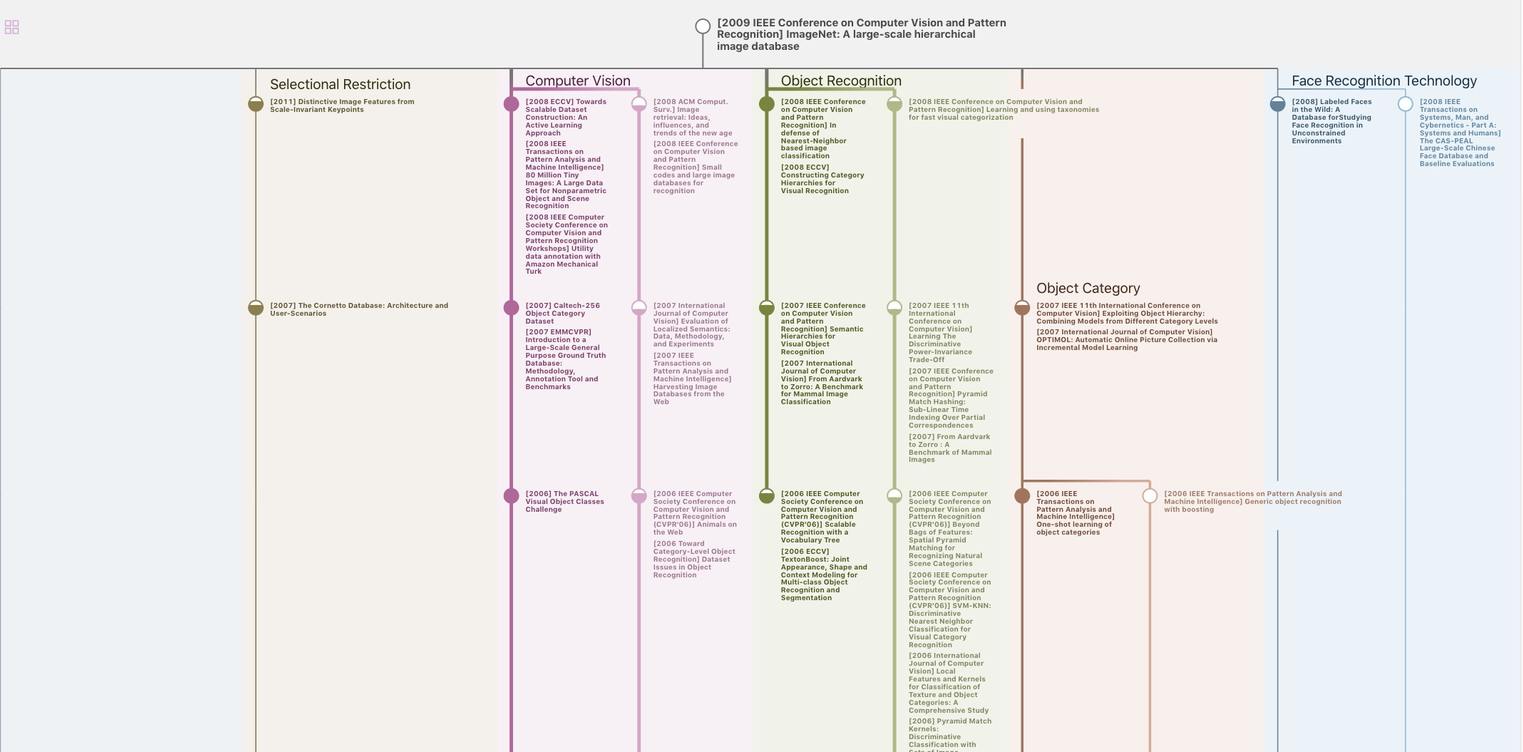
生成溯源树,研究论文发展脉络
Chat Paper
正在生成论文摘要