Masked Contrastive Representation Learning
arXiv (Cornell University)(2022)
摘要
Masked image modelling (e.g., Masked AutoEncoder) and contrastive learning (e.g., Momentum Contrast) have shown impressive performance on unsupervised visual representation learning. This work presents Masked Contrastive Representation Learning (MACRL) for self-supervised visual pre-training. In particular, MACRL leverages the effectiveness of both masked image modelling and contrastive learning. We adopt an asymmetric setting for the siamese network (i.e., encoder-decoder structure in both branches), where one branch with higher mask ratio and stronger data augmentation, while the other adopts weaker data corruptions. We optimize a contrastive learning objective based on the learned features from the encoder in both branches. Furthermore, we minimize the $L_1$ reconstruction loss according to the decoders' outputs. In our experiments, MACRL presents superior results on various vision benchmarks, including CIFAR-10, CIFAR-100, Tiny-ImageNet, and two other ImageNet subsets. Our framework provides unified insights on self-supervised visual pre-training and future research.
更多查看译文
关键词
representation,learning
AI 理解论文
溯源树
样例
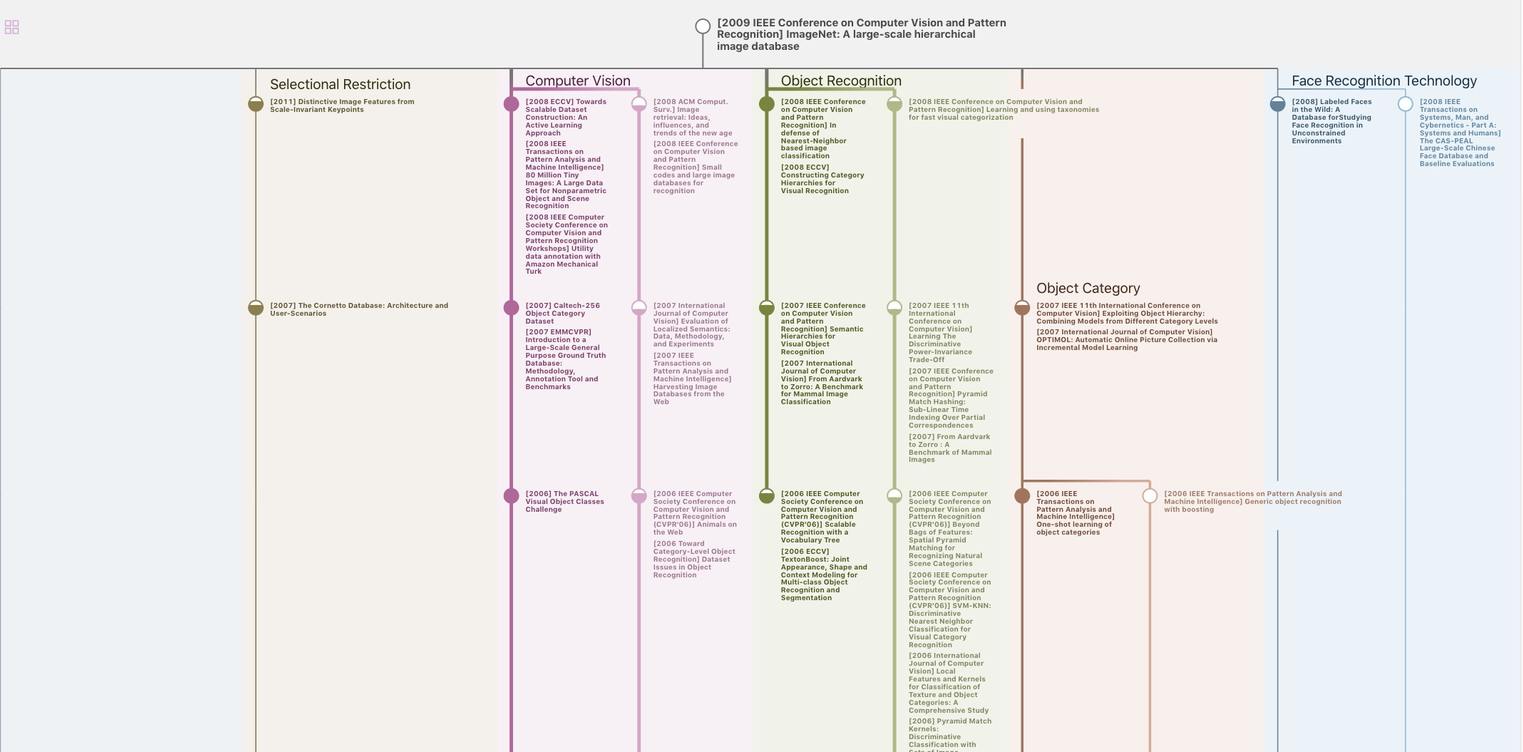
生成溯源树,研究论文发展脉络
Chat Paper
正在生成论文摘要