Increasing Visual Awareness in Multimodal Neural Machine Translation from an Information Theoretic Perspective
arXiv (Cornell University)(2022)
摘要
Multimodal machine translation (MMT) aims to improve translation quality by equipping the source sentence with its corresponding image. Despite the promising performance, MMT models still suffer the problem of input degradation: models focus more on textual information while visual information is generally overlooked. In this paper, we endeavor to improve MMT performance by increasing visual awareness from an information theoretic perspective. In detail, we decompose the informative visual signals into two parts: source-specific information and target-specific information. We use mutual information to quantify them and propose two methods for objective optimization to better leverage visual signals. Experiments on two datasets demonstrate that our approach can effectively enhance the visual awareness of MMT model and achieve superior results against strong baselines.
更多查看译文
关键词
multimodal neural machine translation
AI 理解论文
溯源树
样例
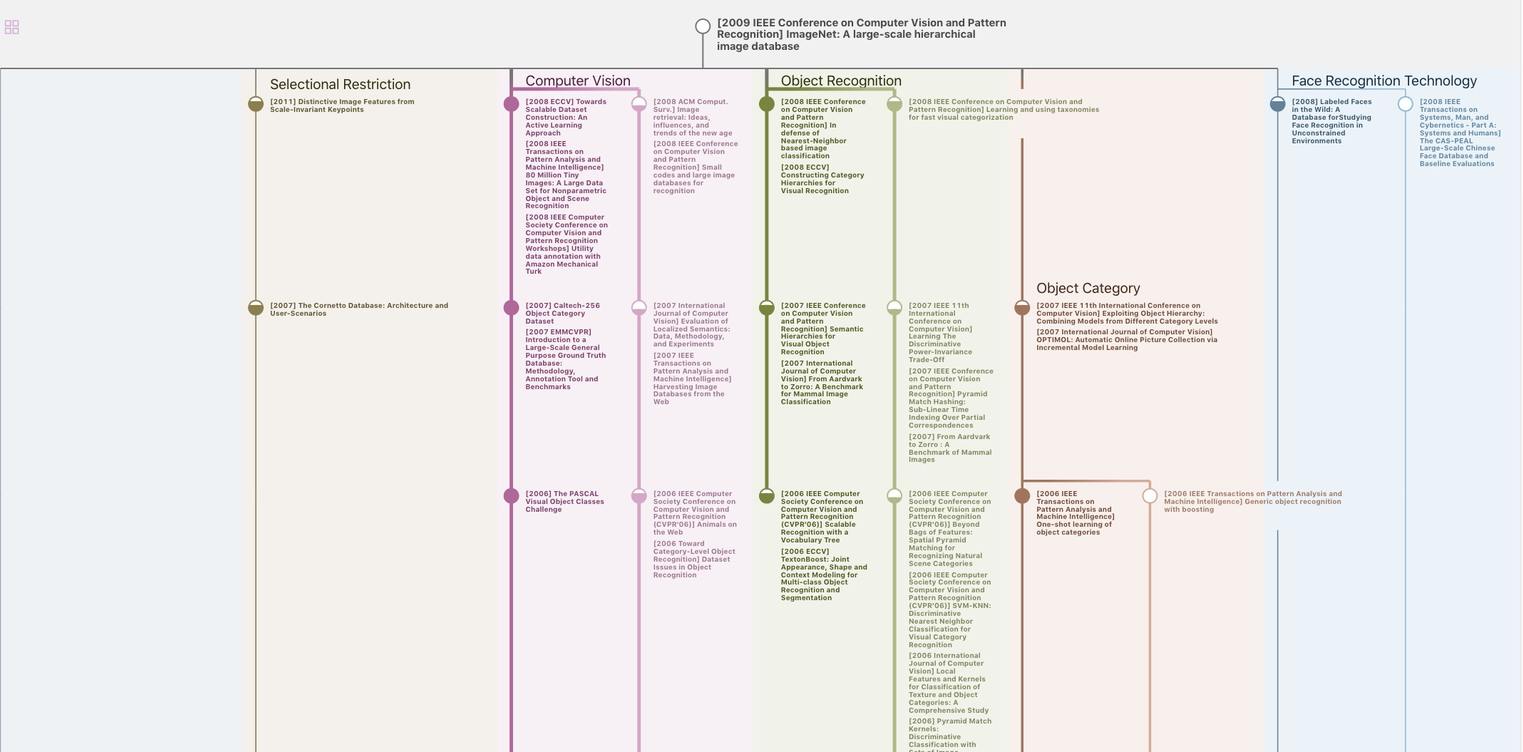
生成溯源树,研究论文发展脉络
Chat Paper
正在生成论文摘要