Self-supervised Video Representation Learning with Motion-Aware Masked Autoencoders
arXiv (Cornell University)(2022)
摘要
Masked autoencoders (MAEs) have emerged recently as art self-supervised spatiotemporal representation learners. Inheriting from the image counterparts, however, existing video MAEs still focus largely on static appearance learning whilst are limited in learning dynamic temporal information hence less effective for video downstream tasks. To resolve this drawback, in this work we present a motion-aware variant -- MotionMAE. Apart from learning to reconstruct individual masked patches of video frames, our model is designed to additionally predict the corresponding motion structure information over time. This motion information is available at the temporal difference of nearby frames. As a result, our model can extract effectively both static appearance and dynamic motion spontaneously, leading to superior spatiotemporal representation learning capability. Extensive experiments show that our MotionMAE outperforms significantly both supervised learning baseline and state-of-the-art MAE alternatives, under both domain-specific and domain-generic pretraining-then-finetuning settings. In particular, when using ViT-B as the backbone our MotionMAE surpasses the prior art model by a margin of 1.2% on Something-Something V2 and 3.2% on UCF101 in domain-specific pretraining setting. Encouragingly, it also surpasses the competing MAEs by a large margin of over 3% on the challenging video object segmentation task. The code is available at https://github.com/happy-hsy/MotionMAE.
更多查看译文
关键词
video representation learning,self-supervised,motion-aware
AI 理解论文
溯源树
样例
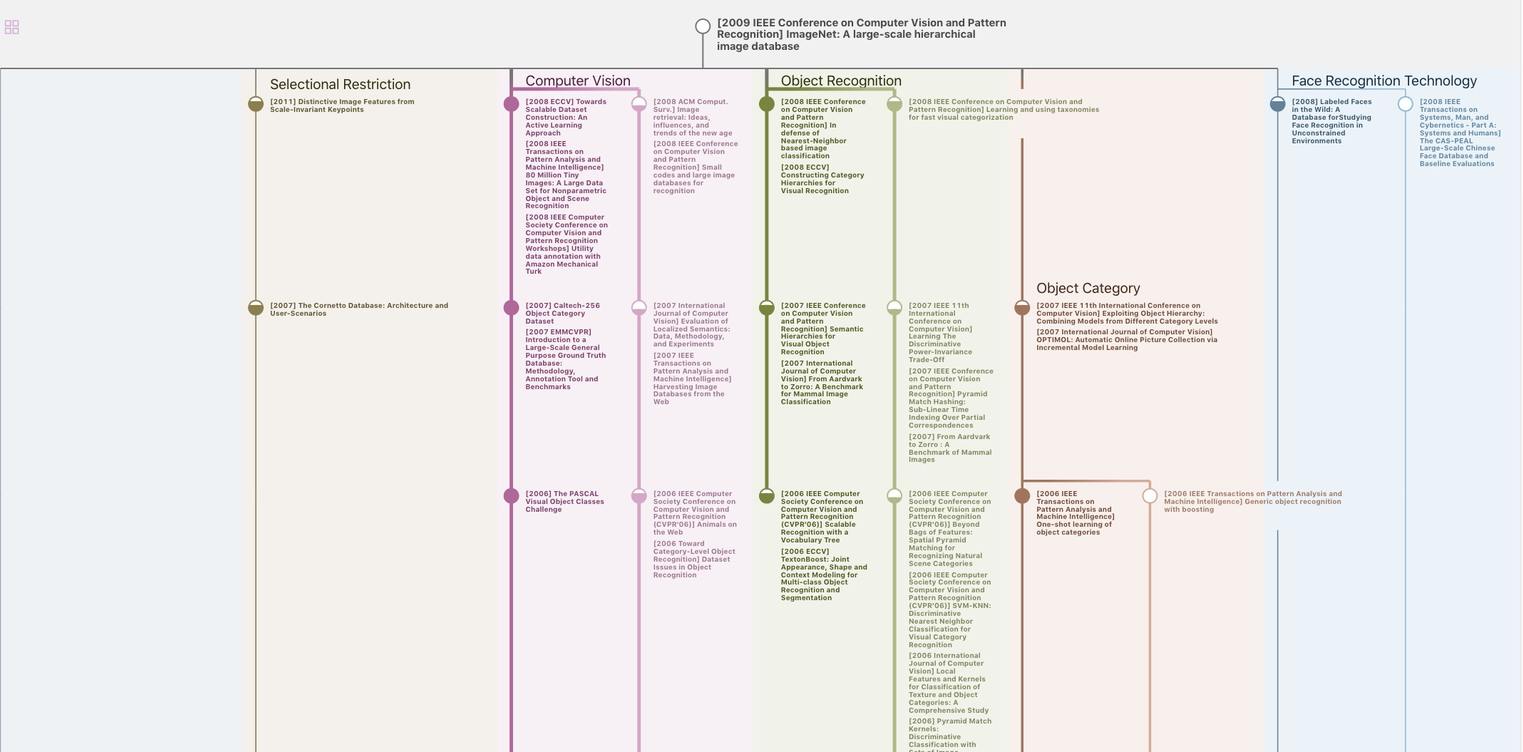
生成溯源树,研究论文发展脉络
Chat Paper
正在生成论文摘要