Coarse-to-fine Task-driven Inpainting for Geoscience Images
arXiv (Cornell University)(2022)
摘要
The processing and recognition of geoscience images have wide applications. Most of existing researches focus on understanding the high-quality geoscience images by assuming that all the images are clear. However, in many real-world cases, the geoscience images might contain occlusions during the image acquisition. This problem actually implies the image inpainting problem in computer vision and multimedia. To the best of our knowledge, all the existing image inpainting algorithms learn to repair the occluded regions for a better visualization quality, they are excellent for natural images but not good enough for geoscience images by ignoring the geoscience related tasks. This paper aims to repair the occluded regions for a better geoscience task performance with the advanced visualization quality simultaneously, without changing the current deployed deep learning based geoscience models. Because of the complex context of geoscience images, we propose a coarse-to-fine encoder-decoder network with coarse-to-fine adversarial context discriminators to reconstruct the occluded image regions. Due to the limited data of geoscience images, we use a MaskMix based data augmentation method to exploit more information from limited geoscience image data. The experimental results on three public geoscience datasets for remote sensing scene recognition, cross-view geolocation and semantic segmentation tasks respectively show the effectiveness and accuracy of the proposed method.
更多查看译文
关键词
inpainting,images,coarse-to-fine,task-driven
AI 理解论文
溯源树
样例
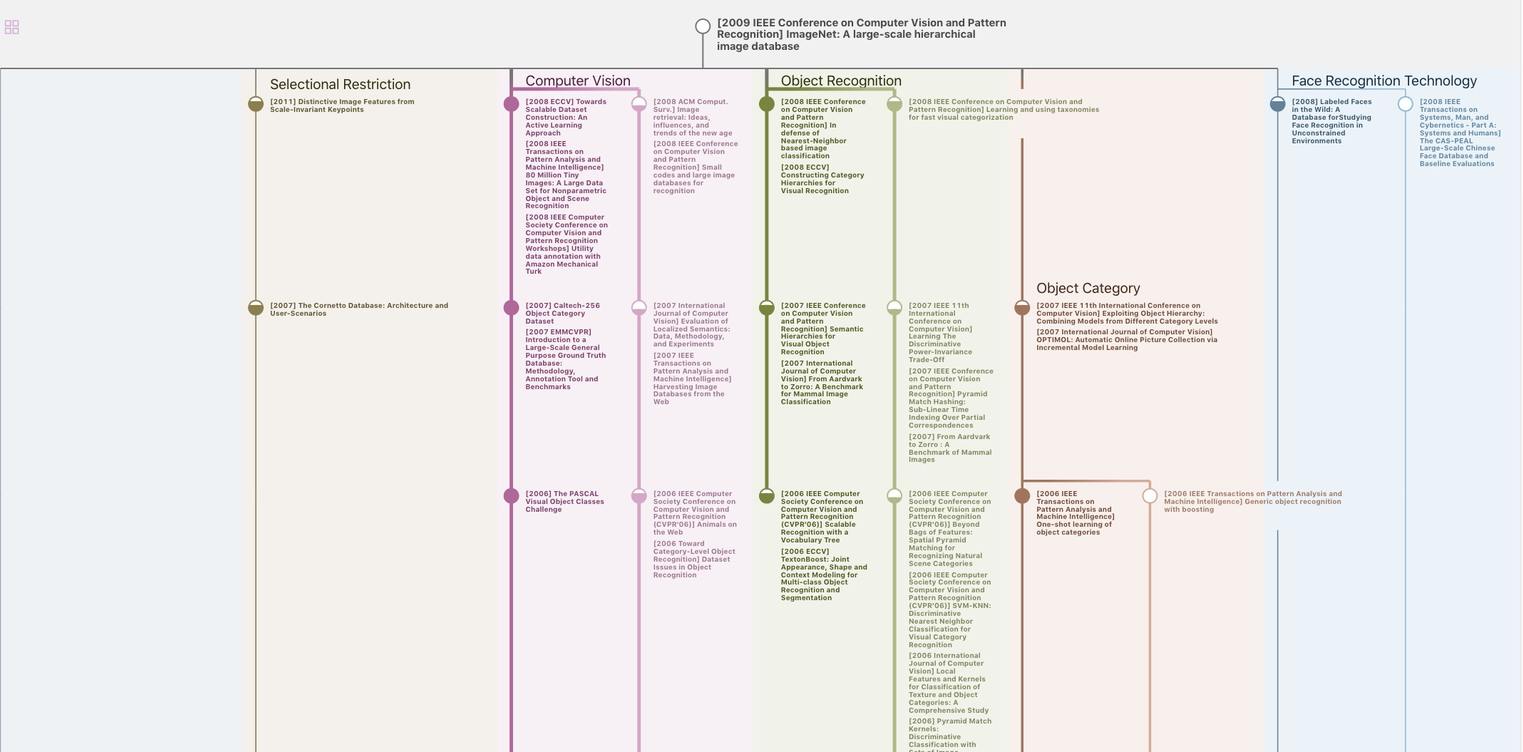
生成溯源树,研究论文发展脉络
Chat Paper
正在生成论文摘要