Self-supervised Learning with Multi-view Rendering for 3D Point Cloud Analysis
Lecture Notes in Computer Science(2023)
摘要
Recently, great progress has been made in 3D deep learning with the emergence of deep neural networks specifically designed for 3D point clouds. These networks are often trained from scratch or from pre-trained models learned purely from point cloud data. Inspired by the success of deep learning in the image domain, we devise a novel pre-training technique for better model initialization by utilizing the multi-view rendering of the 3D data. Our pre-training is self-supervised by a local pixel/point level correspondence loss computed from perspective projection and a global image/point cloud level loss based on knowledge distillation, thus effectively improving upon popular point cloud networks, including PointNet, DGCNN and SR-UNet. These improved models outperform existing state-of-the-art methods on various datasets and downstream tasks. We also analyze the benefits of synthetic and real data for pre-training, and observe that pre-training on synthetic data is also useful for high-level downstream tasks. Code and pre-trained models are available at https://github.com/VinAIResearch/selfsup_pcd.git .
更多查看译文
关键词
learning,self-supervised,multi-view
AI 理解论文
溯源树
样例
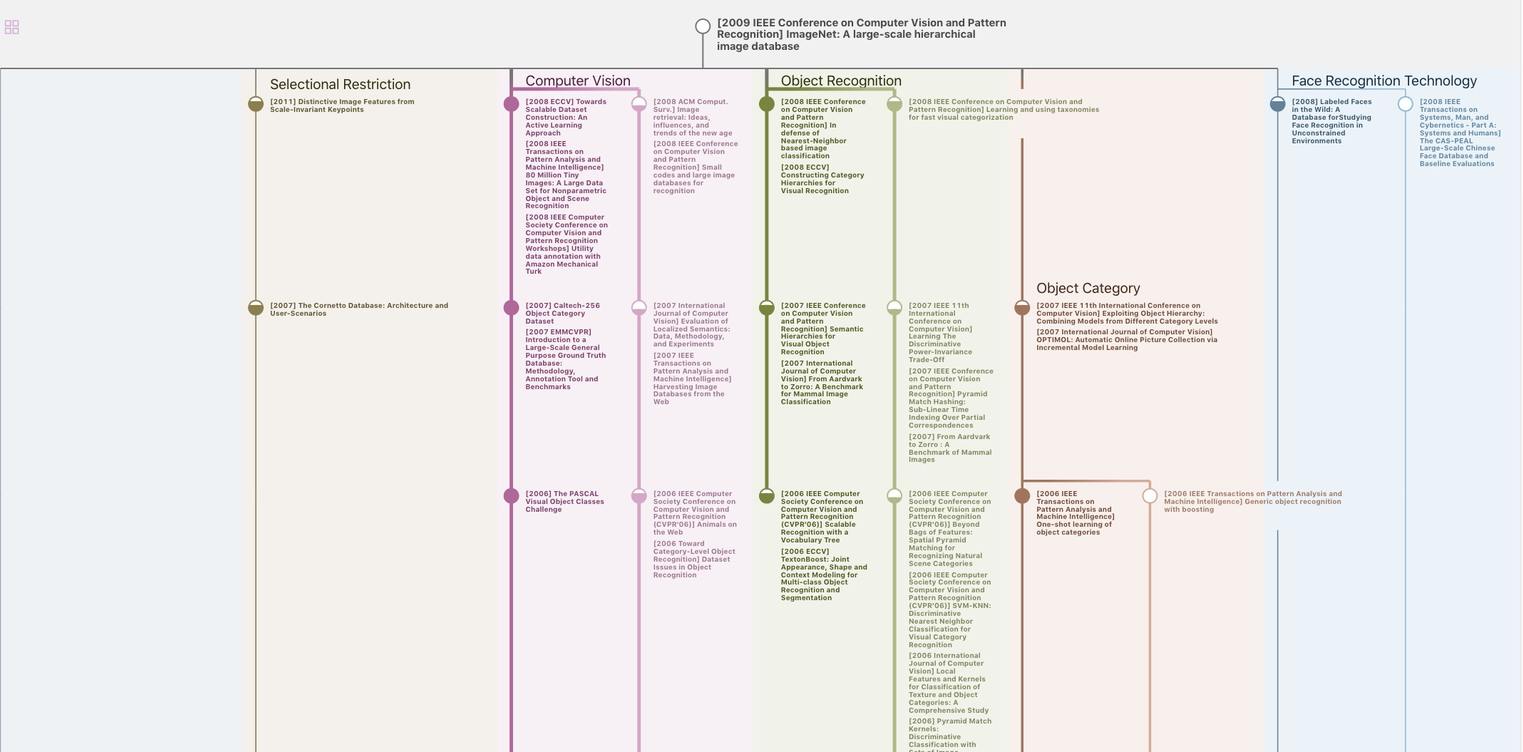
生成溯源树,研究论文发展脉络
Chat Paper
正在生成论文摘要