Functional Bayesian Networks for Discovering Causality from Multivariate Functional Data
arXiv (Cornell University)(2022)
摘要
Multivariate functional data arise in a wide range of applications. One fundamental task is to understand the causal relationships among these functional objects of interest, which has not yet been fully explored. In this article, we develop a novel Bayesian network model for multivariate functional data where the conditional independence and causal structure are both encoded by a directed acyclic graph. Specifically, we allow the functional objects to deviate from Gaussian process, which is adopted by most existing functional data analysis models. The more reasonable non-Gaussian assumption is the key for unique causal structure identification even when the functions are measured with noises. A fully Bayesian framework is designed to infer the functional Bayesian network model with natural uncertainty quantification through posterior summaries. Simulation studies and real data examples are used to demonstrate the practical utility of the proposed model.
更多查看译文
关键词
functional bayesian networks,discovering causality,multivariate
AI 理解论文
溯源树
样例
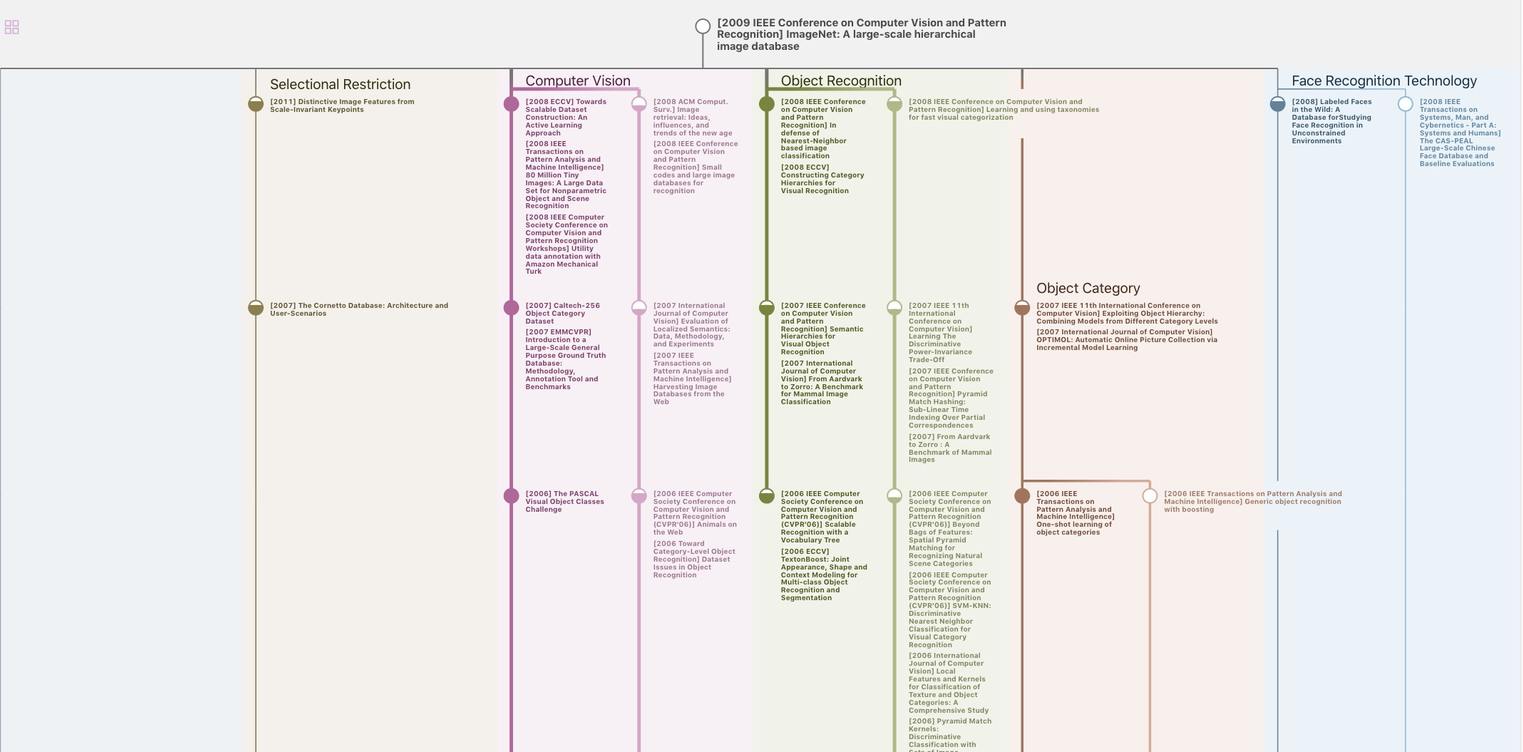
生成溯源树,研究论文发展脉络
Chat Paper
正在生成论文摘要