VQ-T: RNN Transducers using Vector-Quantized Prediction Network States
Interspeech 2022(2022)
摘要
Beam search, which is the dominant ASR decoding algorithm for end-to-end models, generates tree-structured hypotheses. However, recent studies have shown that decoding with hypothesis merging can achieve a more efficient search with comparable or better performance. But, the full context in recurrent networks is not compatible with hypothesis merging. We propose to use vector-quantized long short-term memory units (VQ-LSTM) in the prediction network of RNN transducers. By training the discrete representation jointly with the ASR network, hypotheses can be actively merged for lattice generation. Our experiments on the Switchboard corpus show that the proposed VQ RNN transducers improve ASR performance over transducers with regular prediction networks while also producing denser lattices with a very low oracle word error rate (WER) for the same beam size. Additional language model rescoring experiments also demonstrate the effectiveness of the proposed lattice generation scheme.
更多查看译文
关键词
rnn transducers,prediction,vector-quantized
AI 理解论文
溯源树
样例
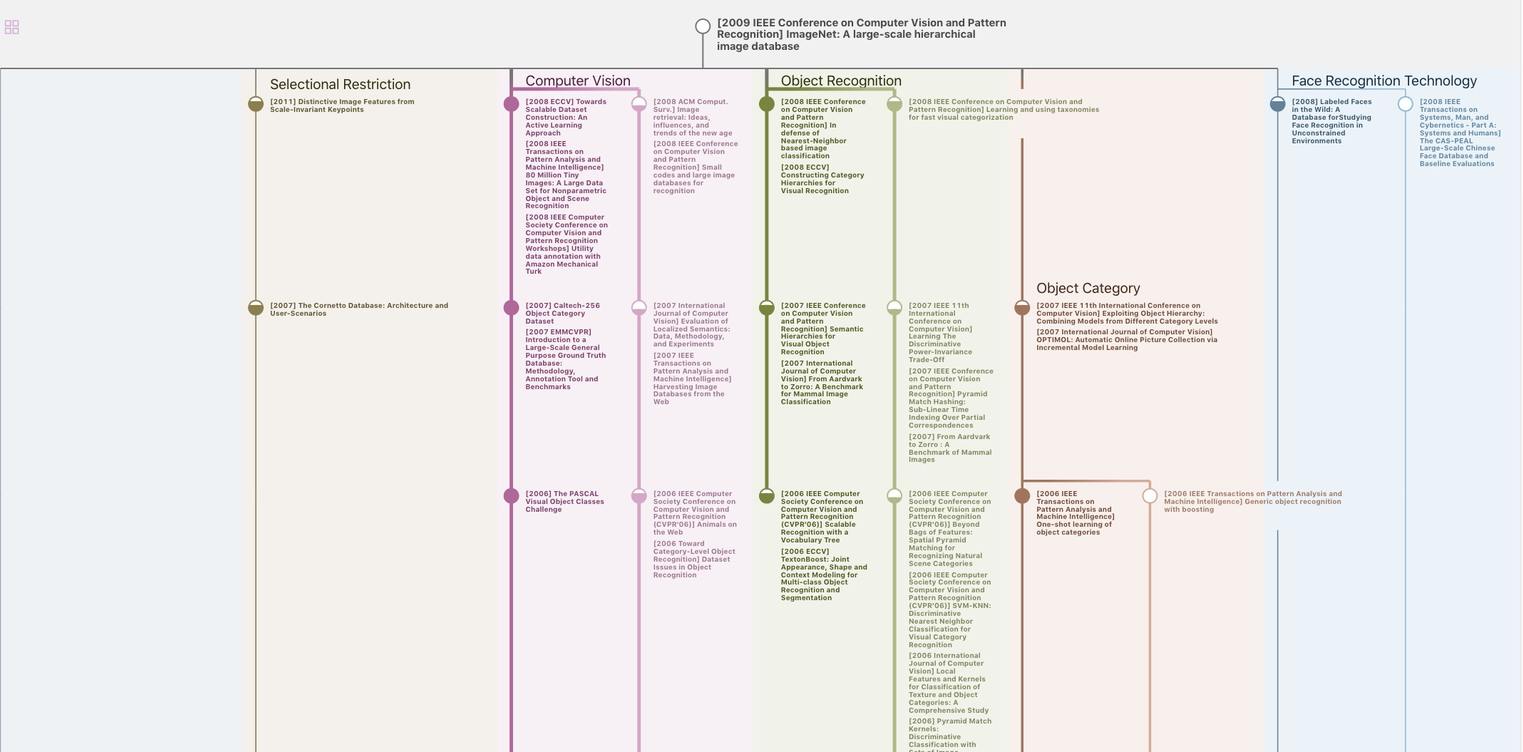
生成溯源树,研究论文发展脉络
Chat Paper
正在生成论文摘要