Spatial-temporal associations representation and application for process monitoring using graph convolution neural network
arXiv (Cornell University)(2022)
摘要
Thank you very much for the attention and concern of colleagues and scholars in this work. With the comments and guidance of experts, editors, and reviewers, this work has been accepted for publishing in the journal "Process Safety and Environmental Protection". The theme of this paper relies on the Spatial-temporal associations of numerous variables in the same industrial processes, which refers to numerous variables obtained in dynamic industrial processes with Spatial-temporal correlation characteristics, i.e., these variables are not only highly correlated in time but also interrelated in space. To handle this problem, three key issues need to be well addressed: variable characteristics modeling and representation, graph network construction (temporal information), and graph characteristics perception. The first issue is implemented by assuming the data follows one improved Gaussian distribution, while the graph network can be defined by the monitoring variables and their edges which are calculated by their characteristics in time. Finally, these networks corresponding to process states at different times are fed into a graph convolutional neural network to implement graph classification to achieve process monitoring. A benchmark experiment (Tennessee Eastman chemical process) and one application study (cobalt purification from zinc solution) are employed to demonstrate the feasibility and applicability of this paper.
更多查看译文
关键词
graph convolution,monitoring,process
AI 理解论文
溯源树
样例
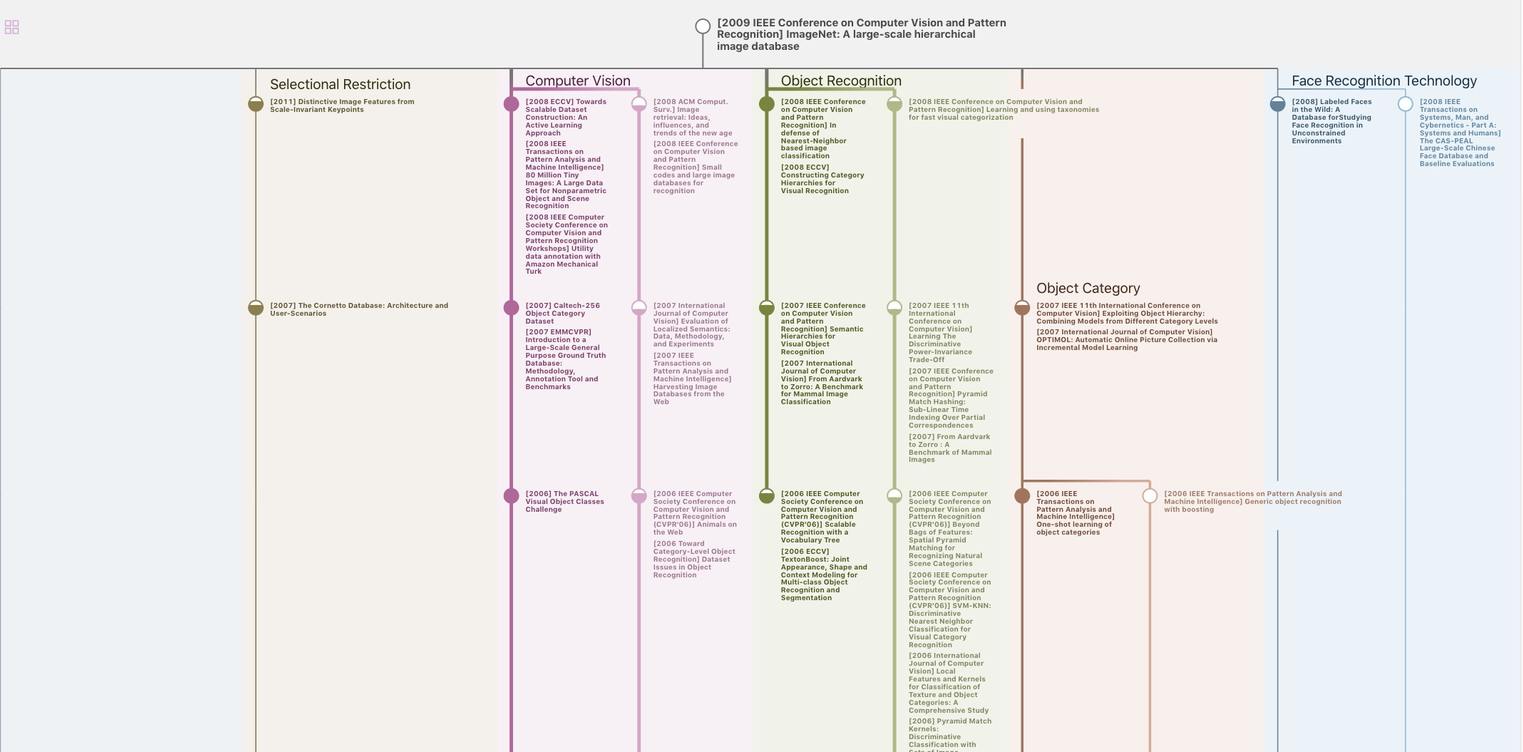
生成溯源树,研究论文发展脉络
Chat Paper
正在生成论文摘要