How to (virtually) train your speaker localizer
arXiv (Cornell University)(2022)
摘要
Learning-based methods have become ubiquitous in speaker localization. Existing systems rely on simulated training sets for the lack of sufficiently large, diverse and annotated real datasets. Most room acoustics simulators used for this purpose rely on the image source method (ISM) because of its computational efficiency. This paper argues that carefully extending the ISM to incorporate more realistic surface, source and microphone responses into training sets can significantly boost the real-world performance of speaker localization systems. It is shown that increasing the training-set realism of a state-of-the-art direction-of-arrival estimator yields consistent improvements across three different real test sets featuring human speakers in a variety of rooms and various microphone arrays. An ablation study further reveals that every added layer of realism contributes positively to these improvements.
更多查看译文
关键词
speaker
AI 理解论文
溯源树
样例
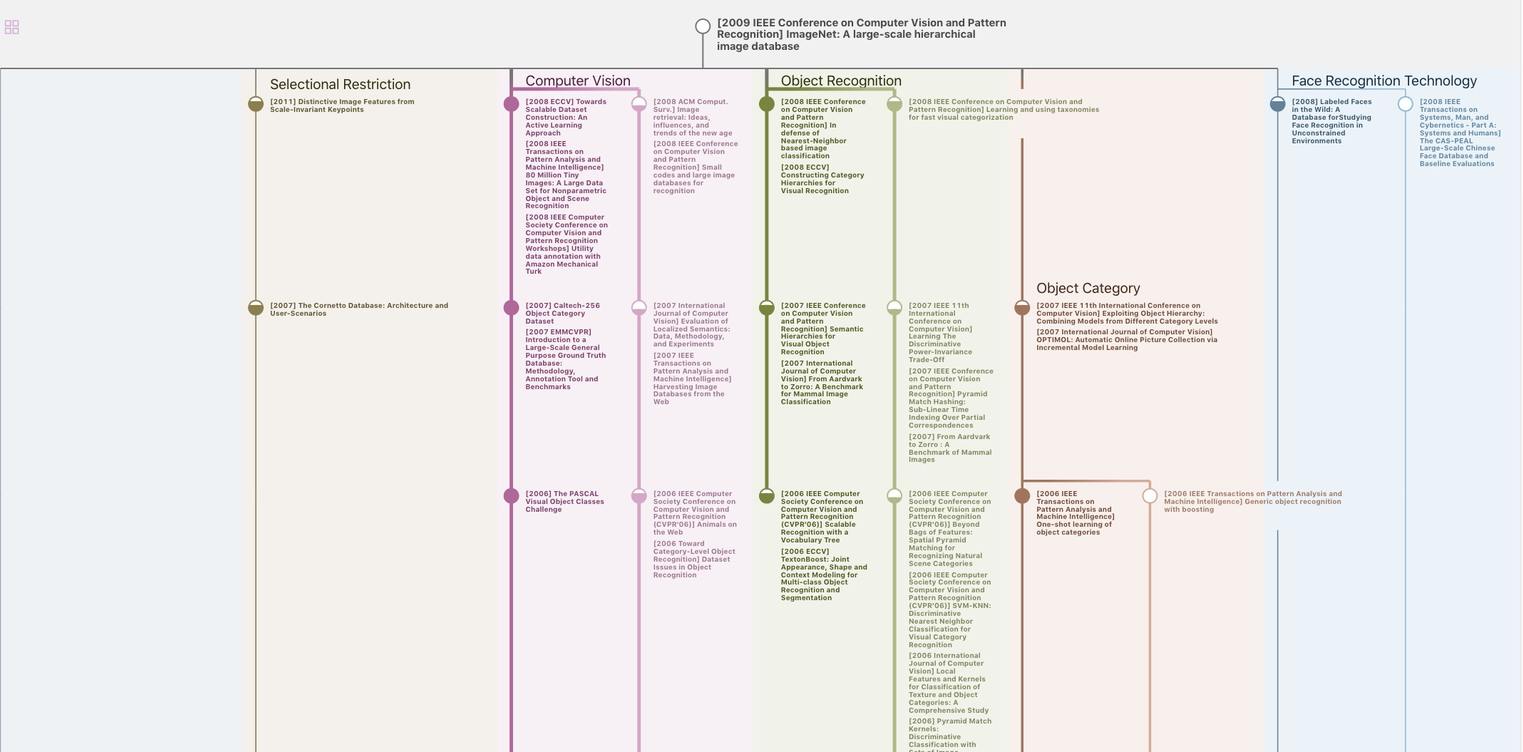
生成溯源树,研究论文发展脉络
Chat Paper
正在生成论文摘要