Harmonizer: Learning to Perform White-Box Image and Video Harmonization
arXiv (Cornell University)(2022)
Abstract
Recent works on image harmonization solve the problem as a pixel-wise image translation task via large autoencoders. They have unsatisfactory performances and slow inference speeds when dealing with high-resolution images. In this work, we observe that adjusting the input arguments of basic image filters, e.g., brightness and contrast, is sufficient for humans to produce realistic images from the composite ones. Hence, we frame image harmonization as an image-level regression problem to learn the arguments of the filters that humans use for the task. We present a Harmonizer framework for image harmonization. Unlike prior methods that are based on black-box autoencoders, Harmonizer contains a neural network for filter argument prediction and several white-box filters (based on the predicted arguments) for image harmonization. We also introduce a cascade regressor and a dynamic loss strategy for Harmonizer to learn filter arguments more stably and precisely. Since our network only outputs image-level arguments and the filters we used are efficient, Harmonizer is much lighter and faster than existing methods. Comprehensive experiments demonstrate that Harmonizer surpasses existing methods notably, especially with high-resolution inputs. Finally, we apply Harmonizer to video harmonization, which achieves consistent results across frames and 56 fps at 1080P resolution. Code and models are available at: https://github.com/ZHKKKe/Harmonizer.
MoreTranslated text
Key words
video harmonization,learning,image,white-box
AI Read Science
Must-Reading Tree
Example
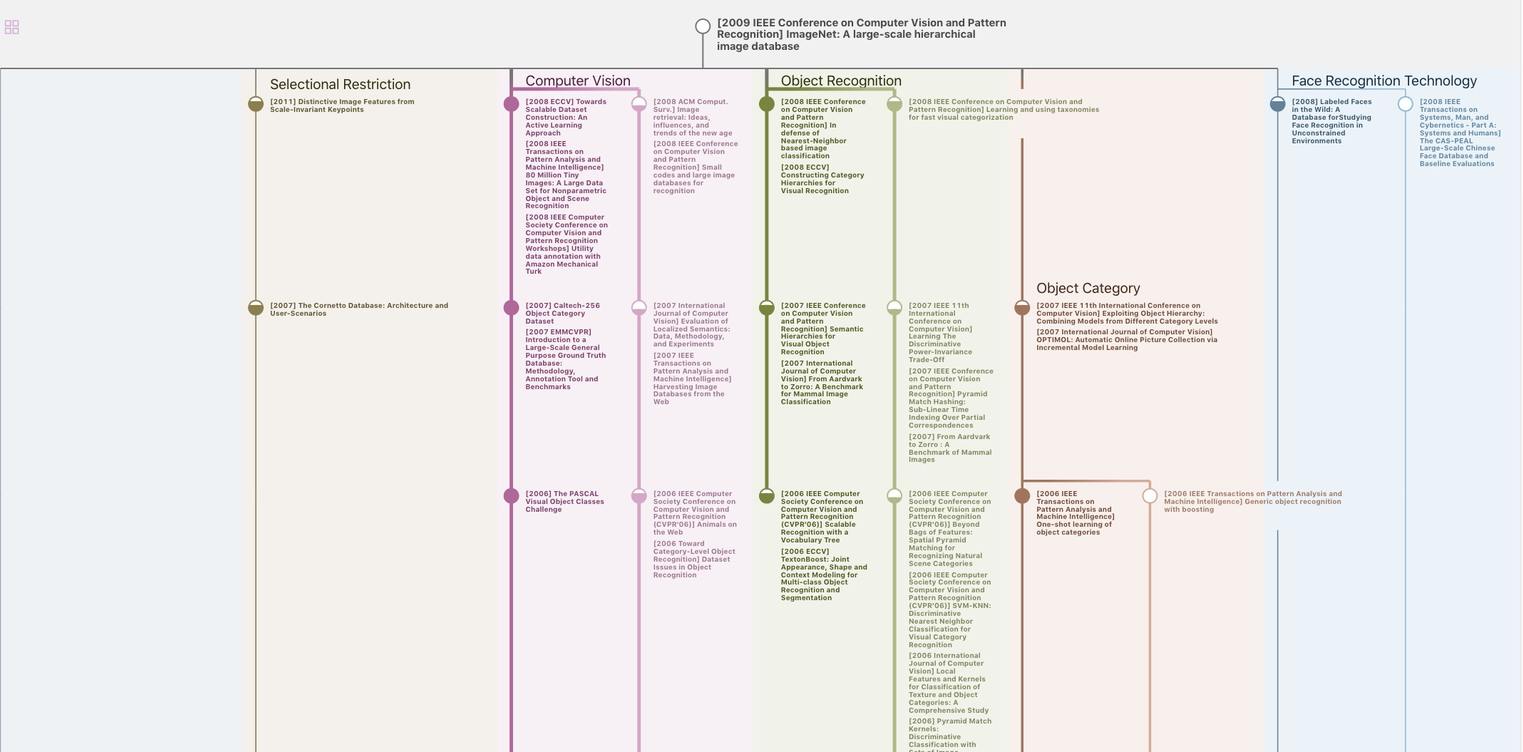
Generate MRT to find the research sequence of this paper
Chat Paper
Summary is being generated by the instructions you defined