COOP-DHGNN: a Framework for Joint Classification and Prediction of Brain Functional Connectivity Using Sparse Trajectory Dataset with Application to Early Dementia
2022 IEEE International Conference on Big Data (Big Data)(2022)
摘要
Graph neural networks for the classification and prediction of brain functional connectivity, are of great value for the early diagnosis and prognosis of progressive neurodegenerative diseases such as Alzheimer’s Disease. However, current graph neural network-based models are suboptimal in three aspects: 1) limited to a single task, 2) require a complete longitudinal dataset and 3) neglect of edge representation learning. To bridge these gaps, we proposed Co-opetition Hypergraph Graph Neural Network (COOPDHGNN), the first general interpretable framework for joint classification (patients with mild cognitive impairment versus cognitively normal individuals) and prediction of brain functional connectivity trajectory, which is compatible with a sparse trajectory dataset. To boost the performance of our graph neural networks, we further proposed Dual Hypergraph Module (DHM) to combine node and edge feature embeddings. Experimental results showed that our model achieved outstanding performance compared to baselines on both classification and prediction with built-in interpretability. Category: Novel research paper.
更多查看译文
关键词
brain functional connectivity,dementia,joint classification,sparse trajectory dataset,coop-dhgnn
AI 理解论文
溯源树
样例
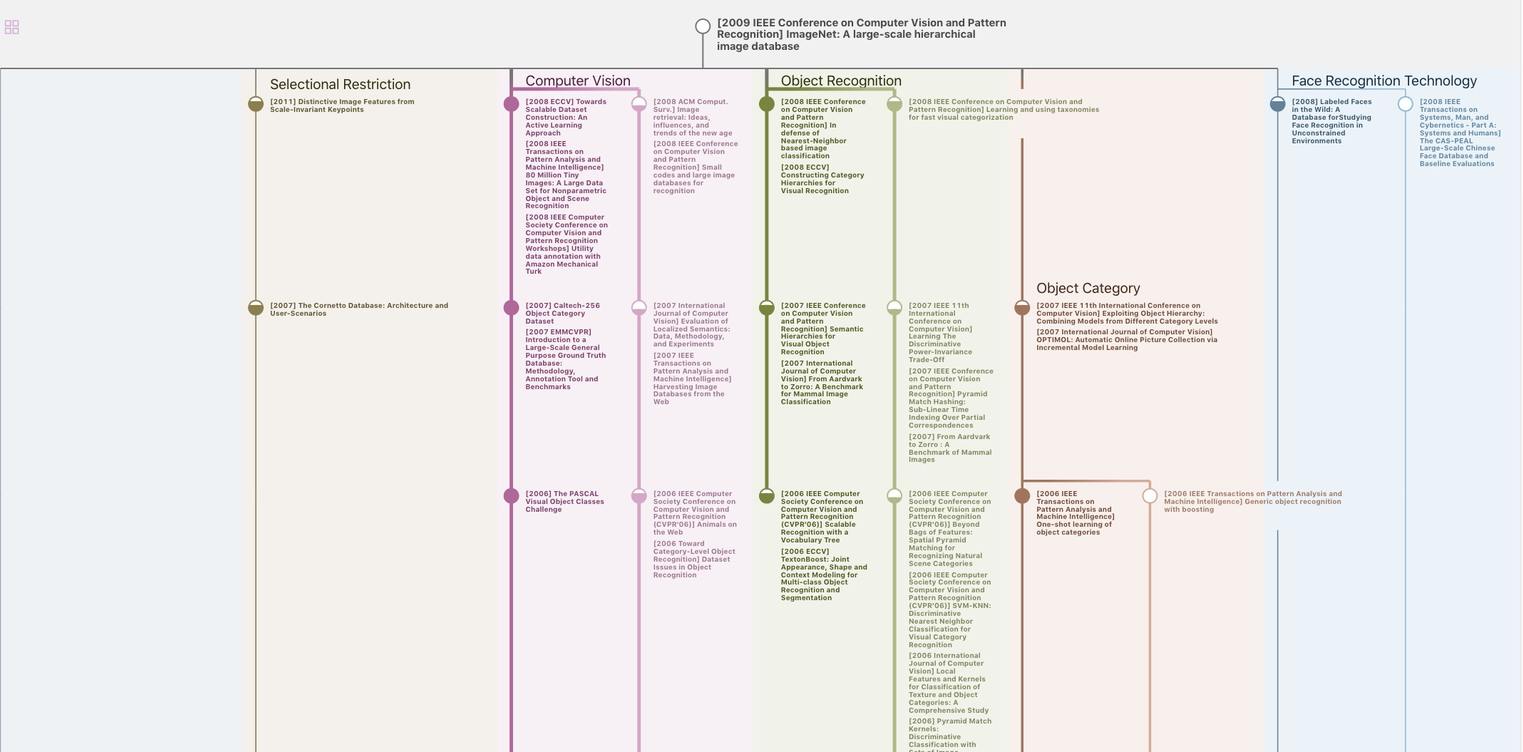
生成溯源树,研究论文发展脉络
Chat Paper
正在生成论文摘要