A Compressed Gradient Tracking Method for Decentralized Optimization with Linear Convergence
arXiv (Cornell University)(2022)
Abstract
Communication compression techniques are of growing interests for solving the decentralized optimization problem under limited communication, where the global objective is to minimize the average of local cost functions over a multi-agent network using only local computation and peer-to-peer communication. In this paper, we propose a novel compressed gradient tracking algorithm (C-GT) that combines gradient tracking technique with communication compression. In particular, C-GT is compatible with a general class of compression operators that unifies both unbiased and biased compressors. We show that C-GT inherits the advantages of gradient tracking-based algorithms and achieves linear convergence rate for strongly convex and smooth objective functions. Numerical examples complement the theoretical findings and demonstrate the efficiency and flexibility of the proposed algorithm.
MoreTranslated text
Key words
compressed gradient tracking method,decentralized optimization
AI Read Science
Must-Reading Tree
Example
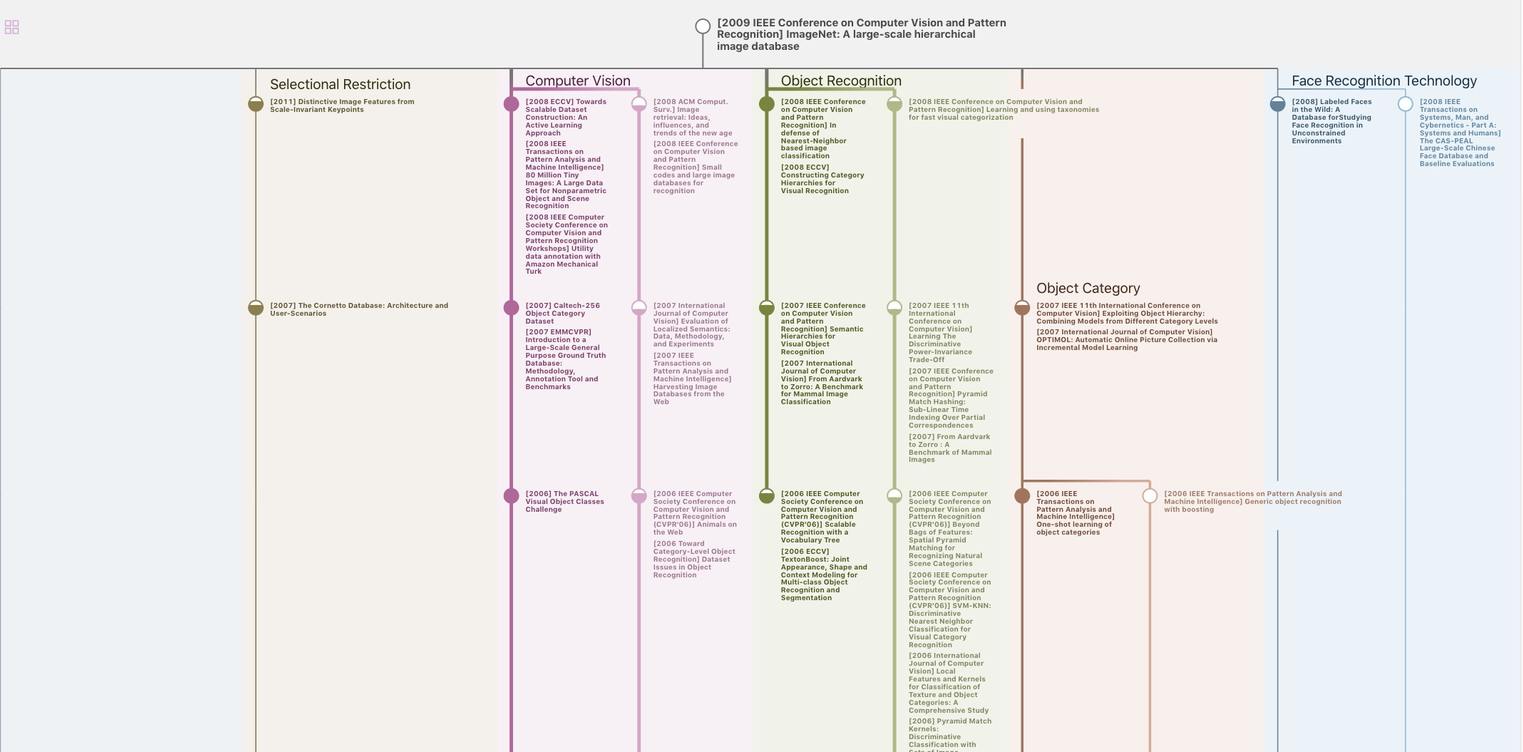
Generate MRT to find the research sequence of this paper
Chat Paper
Summary is being generated by the instructions you defined