Hyper-differential sensitivity analysis with respect to model discrepancy: Calibration and optimal solution updating
arXiv (Cornell University)(2022)
摘要
Optimization constrained by computational models is common across science and engineering. However, in many cases a high-fidelity numerical emulation of systems cannot be optimized due to complexity and computational costs. Rather, low-fidelity models are constructed to enable intrusive algorithms for large-scale optimization. As a result of the discrepancy between high and low-fidelity models, optimal solutions determined using low-fidelity models are frequently far from true optimality. In this article we introduce a novel approach that uses post-optimality sensitivities with respect to model discrepancy to enable solutions representative of the true system. Limited high-fidelity data is used to calibrate the model discrepancy in a Bayesian framework which in turn is propagated through the optimization problem. The result provides significant improvement in optimal solutions with uncertainty characterizations. Our formulation exploits structure in the post-optimality sensitivity operator to achieve computational scalability. Numerical results demonstrate how an optimal solution computed using a low-fidelity model may be significantly improved with limited evaluations of a high-fidelity model.
更多查看译文
关键词
sensitivity,calibration,hyper-differential
AI 理解论文
溯源树
样例
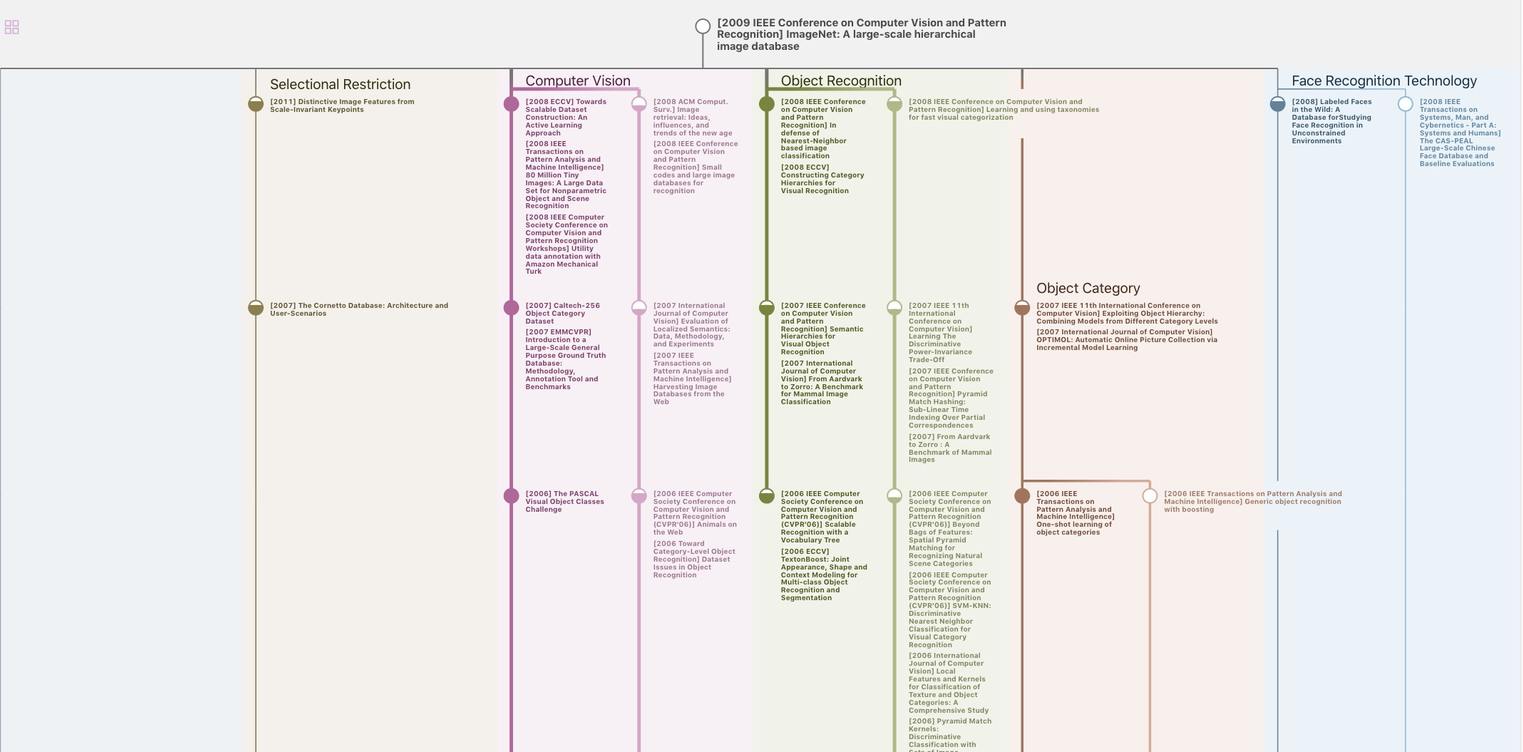
生成溯源树,研究论文发展脉络
Chat Paper
正在生成论文摘要