Hierarchical Dependency Constrained Tree Augmented Naive Bayes Classifiers for Hierarchical Feature Spaces
arXiv (Cornell University)(2022)
摘要
The Tree Augmented Naive Bayes (TAN) classifier is a type of probabilistic graphical model that constructs a single-parent dependency tree to estimate the distribution of the data. In this work, we propose two novel Hierarchical dependency-based Tree Augmented Naive Bayes algorithms, i.e. Hie-TAN and Hie-TAN-Lite. Both methods exploit the pre-defined parent-child (generalisation-specialisation) relationships between features as a type of constraint to learn the tree representation of dependencies among features, whilst the latter further eliminates the hierarchical redundancy during the classifier learning stage. The experimental results showed that Hie-TAN successfully obtained better predictive performance than several other hierarchical dependency constrained classification algorithms, and its predictive performance was further improved by eliminating the hierarchical redundancy, as suggested by the higher accuracy obtained by Hie-TAN-Lite.
更多查看译文
关键词
classifiers
AI 理解论文
溯源树
样例
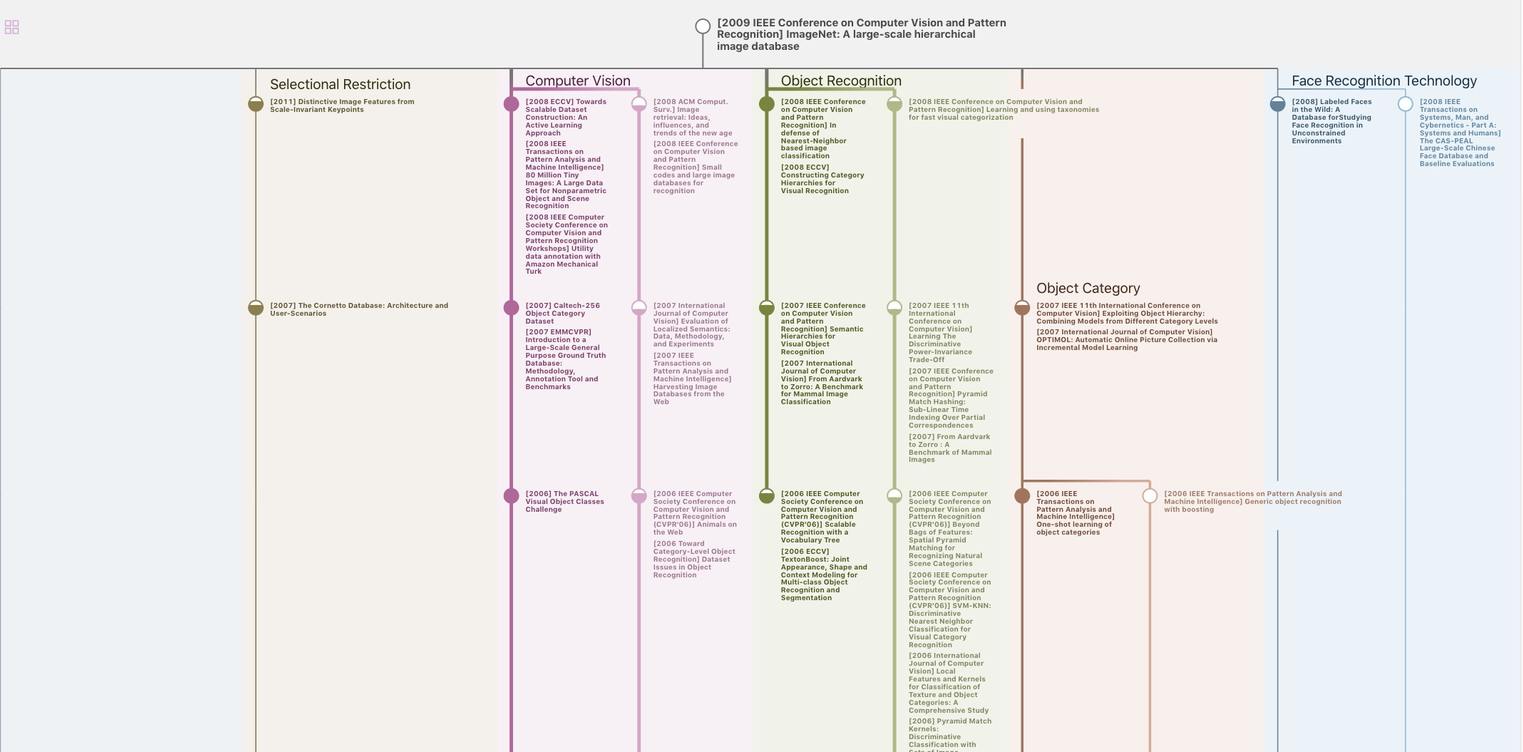
生成溯源树,研究论文发展脉络
Chat Paper
正在生成论文摘要