Frame Mining: a Free Lunch for Learning Robotic Manipulation from 3D Point Clouds
arXiv (Cornell University)(2022)
摘要
We study how choices of input point cloud coordinate frames impact learning of manipulation skills from 3D point clouds. There exist a variety of coordinate frame choices to normalize captured robot-object-interaction point clouds. We find that different frames have a profound effect on agent learning performance, and the trend is similar across 3D backbone networks. In particular, the end-effector frame and the target-part frame achieve higher training efficiency than the commonly used world frame and robot-base frame in many tasks, intuitively because they provide helpful alignments among point clouds across time steps and thus can simplify visual module learning. Moreover, the well-performing frames vary across tasks, and some tasks may benefit from multiple frame candidates. We thus propose FrameMiners to adaptively select candidate frames and fuse their merits in a task-agnostic manner. Experimentally, FrameMiners achieves on-par or significantly higher performance than the best single-frame version on five fully physical manipulation tasks adapted from ManiSkill and OCRTOC. Without changing existing camera placements or adding extra cameras, point cloud frame mining can serve as a free lunch to improve 3D manipulation learning.
更多查看译文
关键词
learning robotic manipulation,3d
AI 理解论文
溯源树
样例
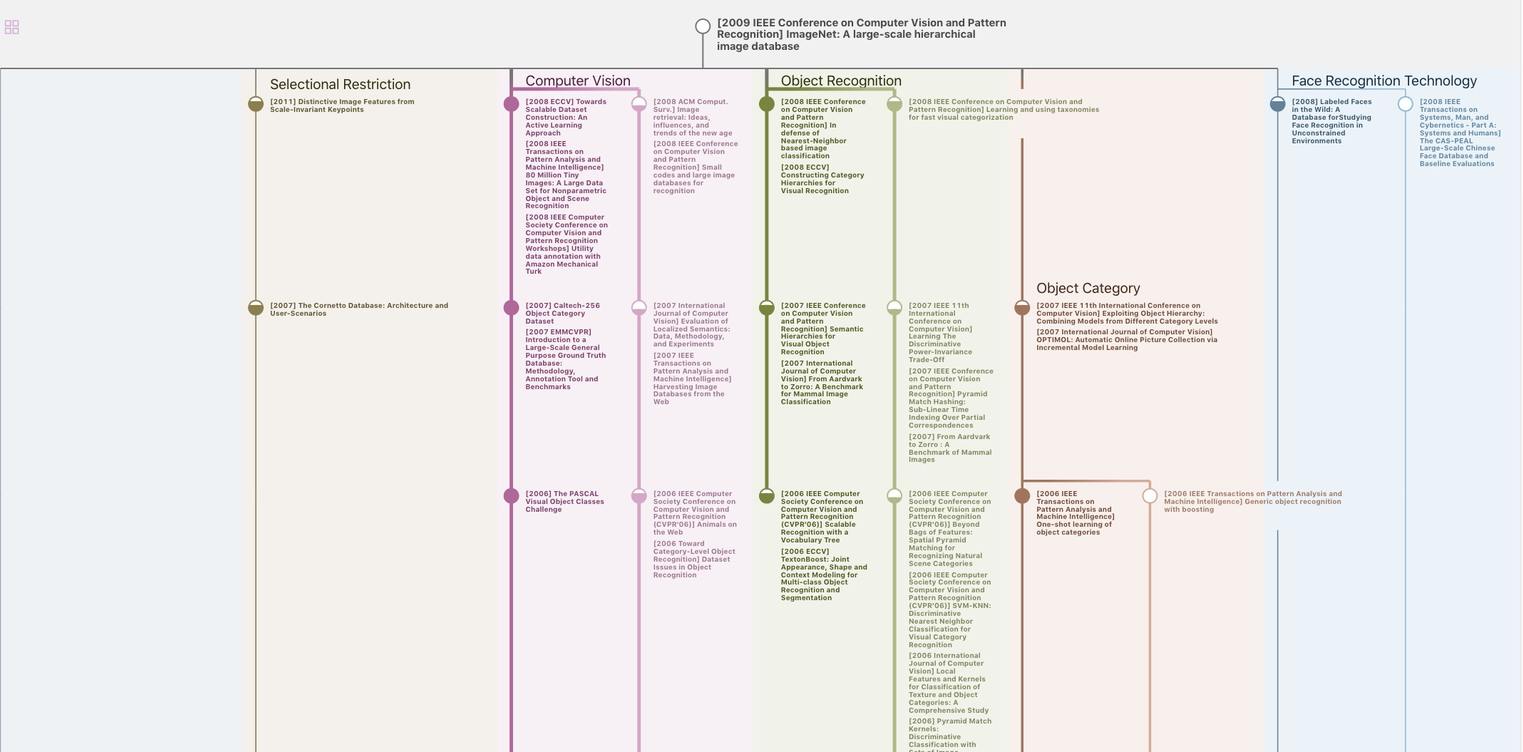
生成溯源树,研究论文发展脉络
Chat Paper
正在生成论文摘要