Solutions for Fine-grained and Long-tailed Snake Species Recognition in SnakeCLEF 2022
arXiv (Cornell University)(2022)
摘要
Automatic snake species recognition is important because it has vast potential to help lower deaths and disabilities caused by snakebites. We introduce our solution in SnakeCLEF 2022 for fine-grained snake species recognition on a heavy long-tailed class distribution. First, a network architecture is designed to extract and fuse features from multiple modalities, i.e. photograph from visual modality and geographic locality information from language modality. Then, logit adjustment based methods are studied to relieve the impact caused by the severe class imbalance. Next, a combination of supervised and self-supervised learning method is proposed to make full use of the dataset, including both labeled training data and unlabeled testing data. Finally, post processing strategies, such as multi-scale and multi-crop test-time-augmentation, location filtering and model ensemble, are employed for better performance. With an ensemble of several different models, a private score 82.65%, ranking the 3rd, is achieved on the final leaderboard.
更多查看译文
关键词
snake species recognition,snakeclef,fine-grained,long-tailed
AI 理解论文
溯源树
样例
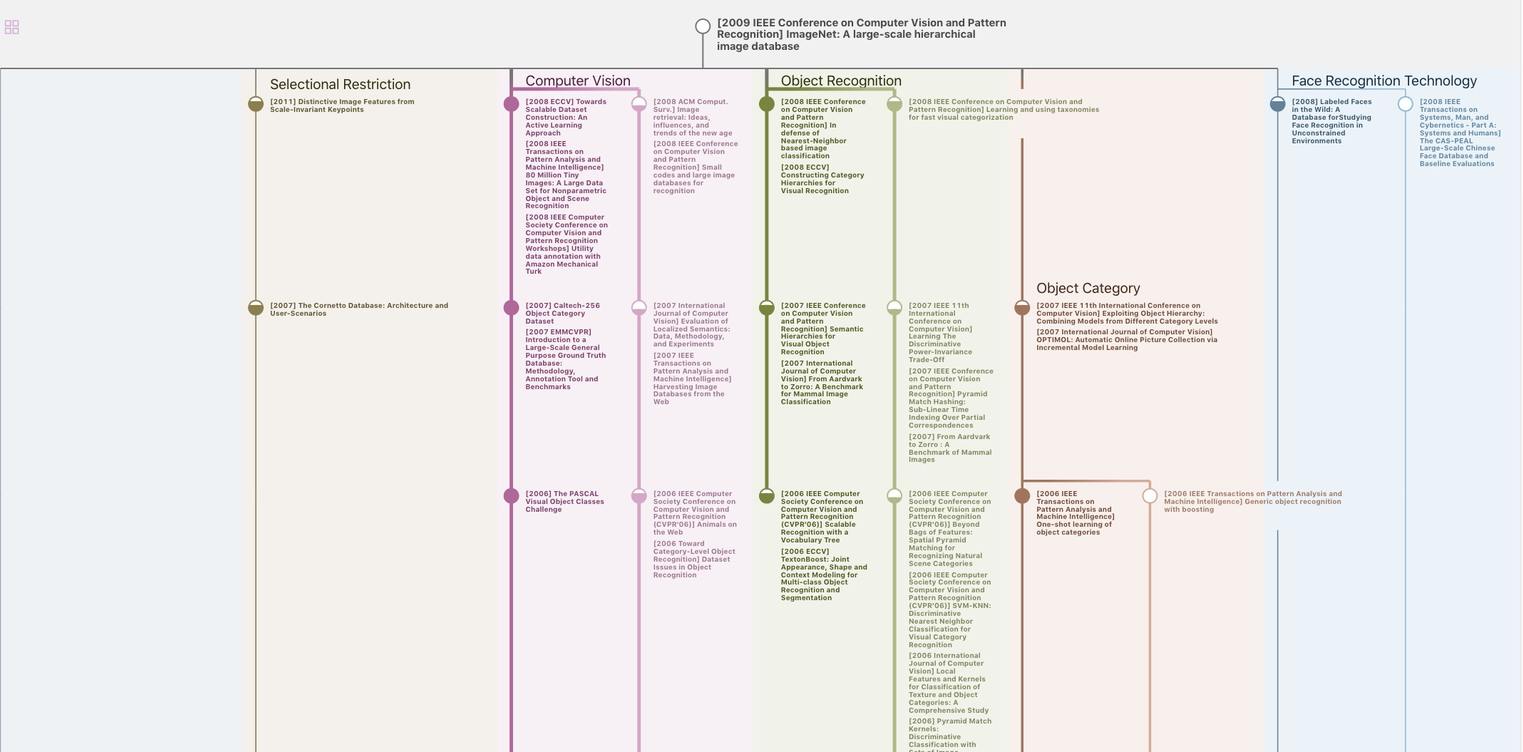
生成溯源树,研究论文发展脉络
Chat Paper
正在生成论文摘要