Enabling Malware Detection with Machine Learning on Programmable Switch
NOMS 2022-2022 IEEE/IFIP Network Operations and Management Symposium(2022)
摘要
Malware detection is an important issue for network security, especially for the Internet of Things (IoT) network. Traditional network intrusion detection system (NIDS), running on external host servers, are not scalable for ever-increasing IoT traffic and waste time on transmitting data back and forth. Here, we propose a novel architecture called on-switch malware detector that utilizes the programmable switch and the machine-learning technique to achieve better performance on detecting malicious flows in the network. The on-switch malware detector mainly consists of four components: (1) packet forwarder, (2) feature extractor, (3) flow director, and (4) neural-network detector. According to the experimental results, the on-switch malware detection has a 99.57% shorter response time than a conventional signature-based NIDS; meanwhile its processing capacity increases by 800 times. As a result, the on-switch malware detector efficiently overcomes the shortcomings of conventional NIDSs, making it a better fit for the IoT network.
更多查看译文
关键词
malware detection,programmable switch,machine learning
AI 理解论文
溯源树
样例
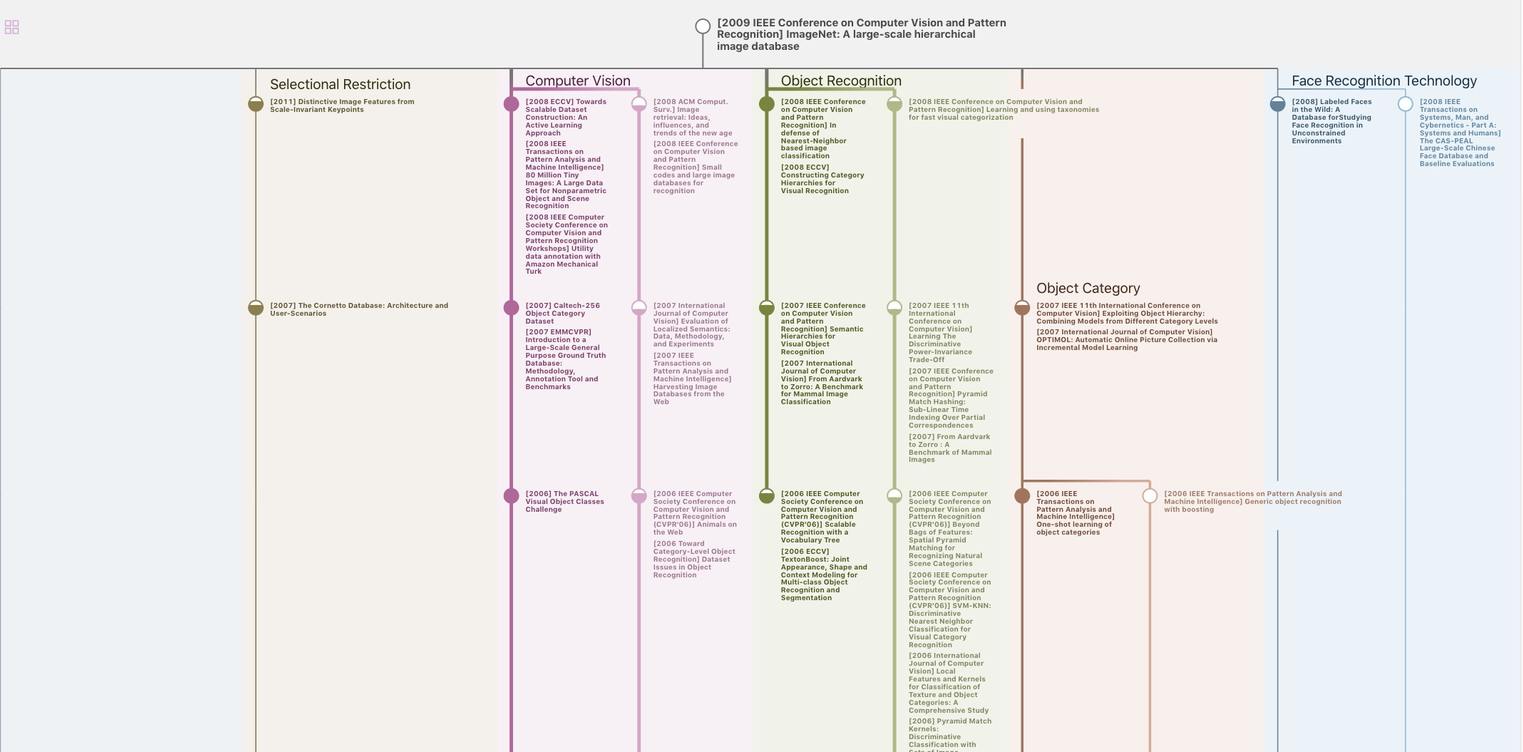
生成溯源树,研究论文发展脉络
Chat Paper
正在生成论文摘要