Abstract 11242: Spatial-Temporal Deep Learning for Automatic Detection of Echocardiographic Predictors of Mortality in Patients Hospitalized With Covid-19 - Data From the PROVAR-COVID Study
Circulation(2022)
摘要
Background: Cardiac involvement seems to impact the prognosis of COVID-19. Bedside echocardiography (echo) holds promise for the early outcome prediction, and artificial intelligence has shown to be an additional tool to overcome personnel limitations. We propose a spatial-temporal deep learning-based approach for automatic prediction of mortality of inpatients with COVID-19 with echo images. Methods: Patients admitted to two hospitals in Brazil in 90 days with confirmed moderate and severe COVID-19 underwent clinical and laboratory evaluation, and focused bedside echo (GE Vivid IQ), following admission, with remote interpretation. Independent echo predictors of all-cause mortality were assessed, after adjustment for clinical variables. Our image dataset consists of 752 videos (Mpeg) acquired in three different probe positions: apical 4-chamber and parasternal long and short axis. We predicted the patients’ outcome (discharge or death) in a 10-fold cross-validation procedure manner, where we applied a 2-stream deep neural network composed of 2 identical ResNet-18 Convolutional Neural Networks (CNN) - pre-trained and developed for Rheumatic Heart Disease - followed by attention units to extract relevant spatial and temporal features from RGB and Optical Flow frames. A final softmax layer is used for classification. Results: A total of 163 patients were enrolled, the mean age was 64±16 years, 107 (66%) were admitted to intensive care and in-hospital mortality was 34% (N=56). Independent predictors of mortality were age≥63 years (OR=5.53, 95%CI 1.52-20.17), LVEF<64% (OR=7.37, 95%CI 2.10-25.94), and TAPSE<18.5 mm (OR=9.43, 95% CI 2.57-35.03), C-statistic=0.83. During training, utilizing a balanced set of 106 patients, we rescaled videos to 224x224 pixels and used temporal jittering to select a final clip with 32 frames per video in random batches of size 6. Our proposed method achieved an exam-wise accuracy of 62.22% (95%CI 52.40-72,04), sensitivity of 67.52% (95%CI 51.62-83.42), and specificity of 60.45% (95%CI 45.15-75.75) for mortality. Conclusion: Automatic detection of high-risk echo findings in COVID-19 inpatients at the bedside seems feasible and, with more research, can improve mortality prediction at the point of care.
更多查看译文
关键词
echocardiographic predictors,mortality,spatial-temporal,provar-covid
AI 理解论文
溯源树
样例
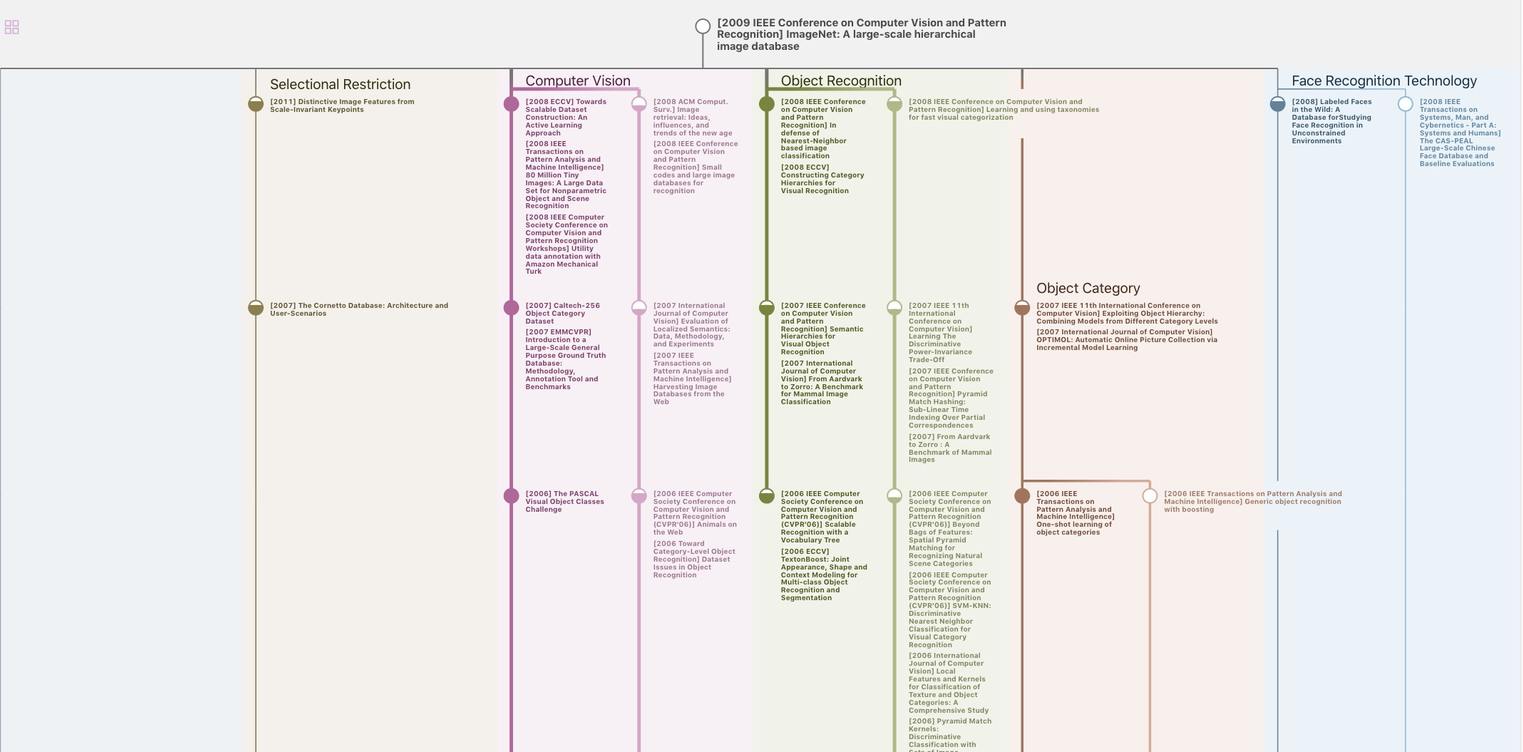
生成溯源树,研究论文发展脉络
Chat Paper
正在生成论文摘要