Learning to Detect Noisy Labels Using Model-Based Features
arXiv (Cornell University)(2022)
摘要
Label noise is ubiquitous in various machine learning scenarios such as self-labeling with model predictions and erroneous data annotation. Many existing approaches are based on heuristics such as sample losses, which might not be flexible enough to achieve optimal solutions. Meta learning based methods address this issue by learning a data selection function, but can be hard to optimize. In light of these pros and cons, we propose Selection-Enhanced Noisy label Training (SENT) that does not rely on meta learning while having the flexibility of being data-driven. SENT transfers the noise distribution to a clean set and trains a model to distinguish noisy labels from clean ones using model-based features. Empirically, on a wide range of tasks including text classification and speech recognition, SENT improves performance over strong baselines under the settings of self-training and label corruption.
更多查看译文
关键词
noisy labels,learning,features,model-based
AI 理解论文
溯源树
样例
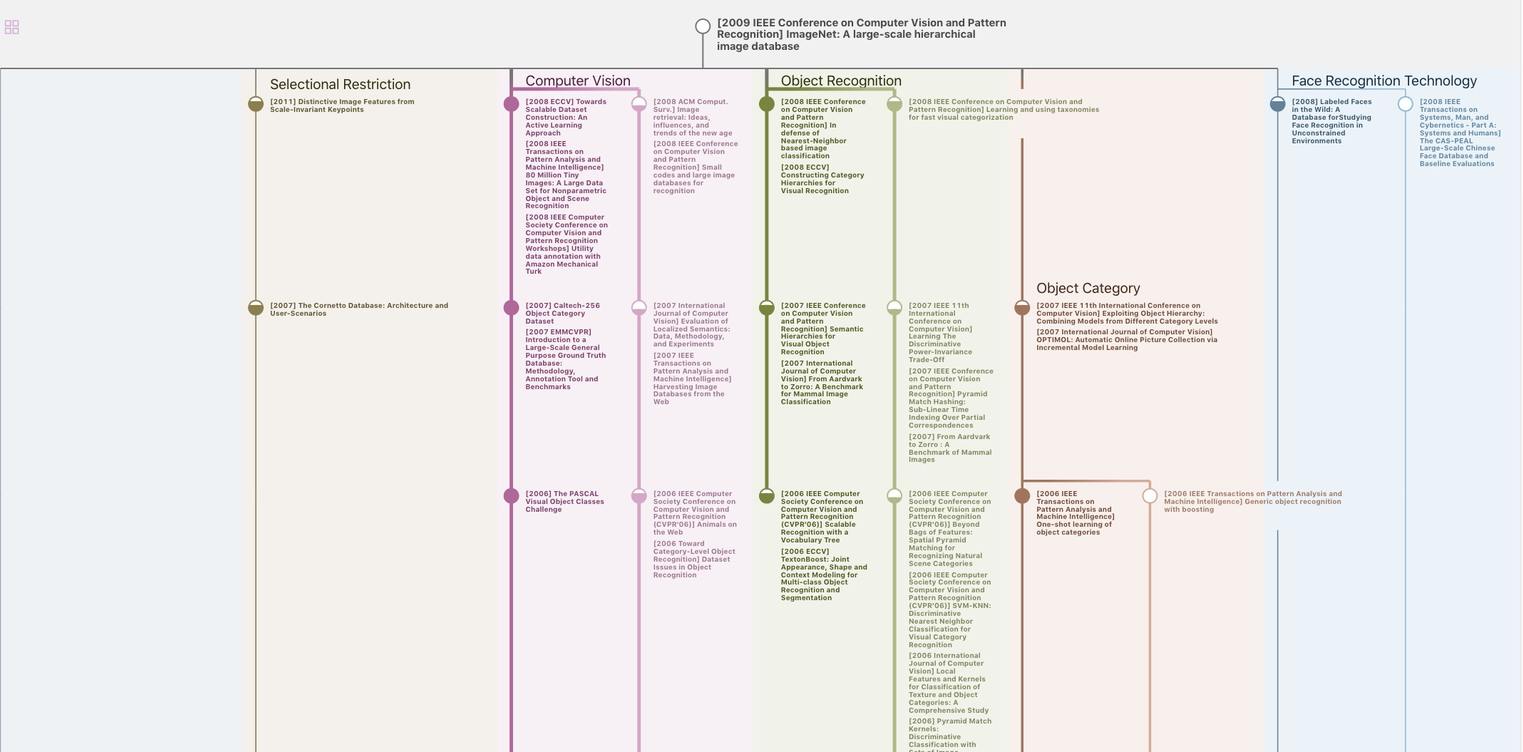
生成溯源树,研究论文发展脉络
Chat Paper
正在生成论文摘要