Knowledge Graph Quality Evaluation under Incomplete Information
arXiv (Cornell University)(2022)
Abstract
Knowledge graphs (KGs) have attracted more and more attentions because of their fundamental roles in many tasks. Quality evaluation for KGs is thus crucial and indispensable. Existing methods in this field evaluate KGs by either proposing new quality metrics from different dimensions or measuring performances at KG construction stages. However, there are two major issues with those methods. First, they highly rely on raw data in KGs, which makes KGs' internal information exposed during quality evaluation. Second, they consider more about the quality at data level instead of ability level, where the latter one is more important for downstream applications. To address these issues, we propose a knowledge graph quality evaluation framework under incomplete information (QEII). The quality evaluation task is transformed into an adversarial Q&A game between two KGs. Winner of the game is thus considered to have better qualities. During the evaluation process, no raw data is exposed, which ensures information protection. Experimental results on four pairs of KGs demonstrate that, compared with baselines, the QEII implements a reasonable quality evaluation at ability level under incomplete information.
MoreTranslated text
Key words
incomplete information,knowledge,quality,graph
AI Read Science
Must-Reading Tree
Example
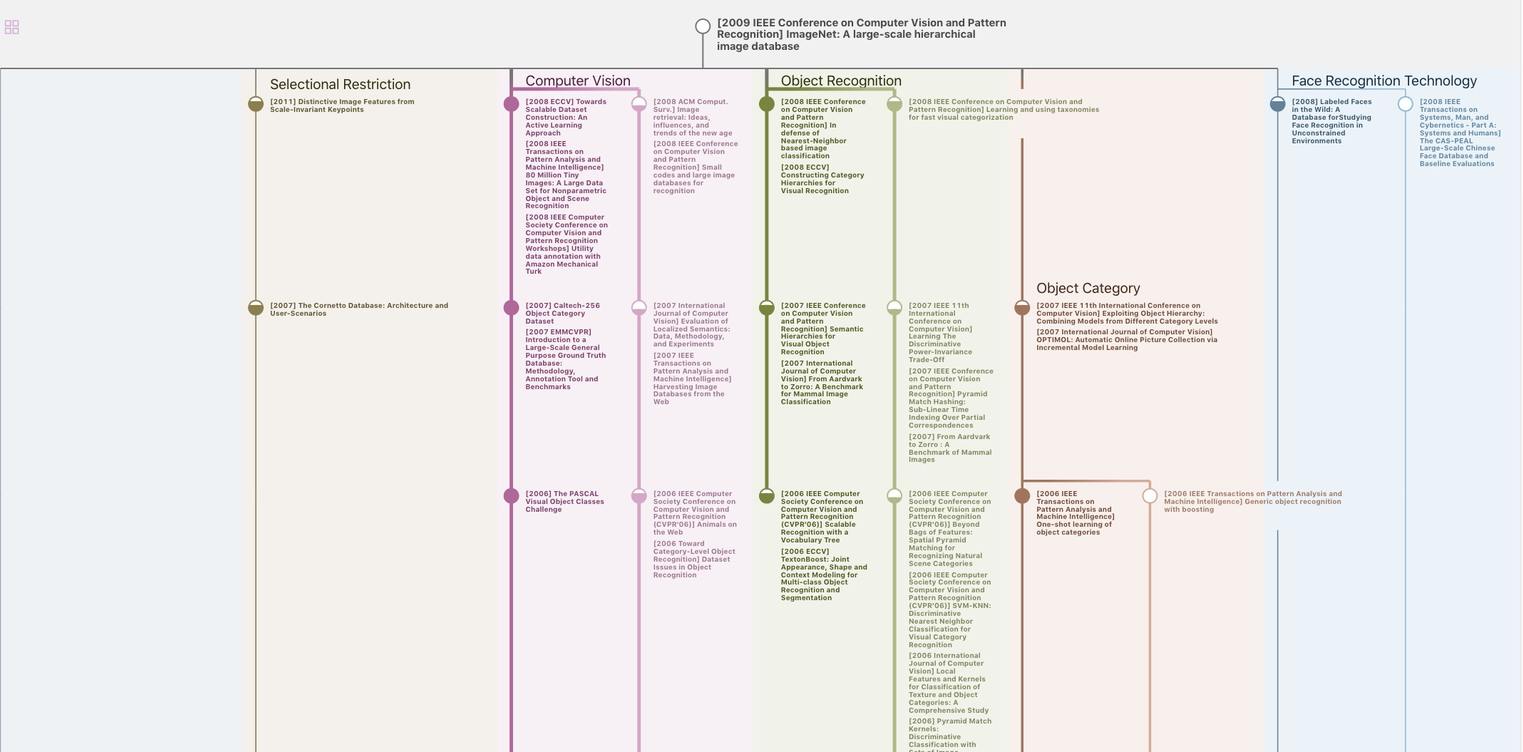
Generate MRT to find the research sequence of this paper
Chat Paper
Summary is being generated by the instructions you defined