Plausible Adversarial Attacks on Direct Parameter Inference Models in Astrophysics
arXiv (Cornell University)(2022)
摘要
In this abstract we explore the possibility of introducing biases in physical parameter inference models from adversarial-type attacks. In particular, we inject small amplitude systematics into inputs to a mixture density networks tasked with inferring cosmological parameters from observed data. The systematics are constructed analogously to white-box adversarial attacks. We find that the analysis network can be tricked into spurious detection of new physics in cases where standard cosmological estimators would be insensitive. This calls into question the robustness of such networks and their utility for reliably detecting new physics.
更多查看译文
关键词
direct parameter inference models
AI 理解论文
溯源树
样例
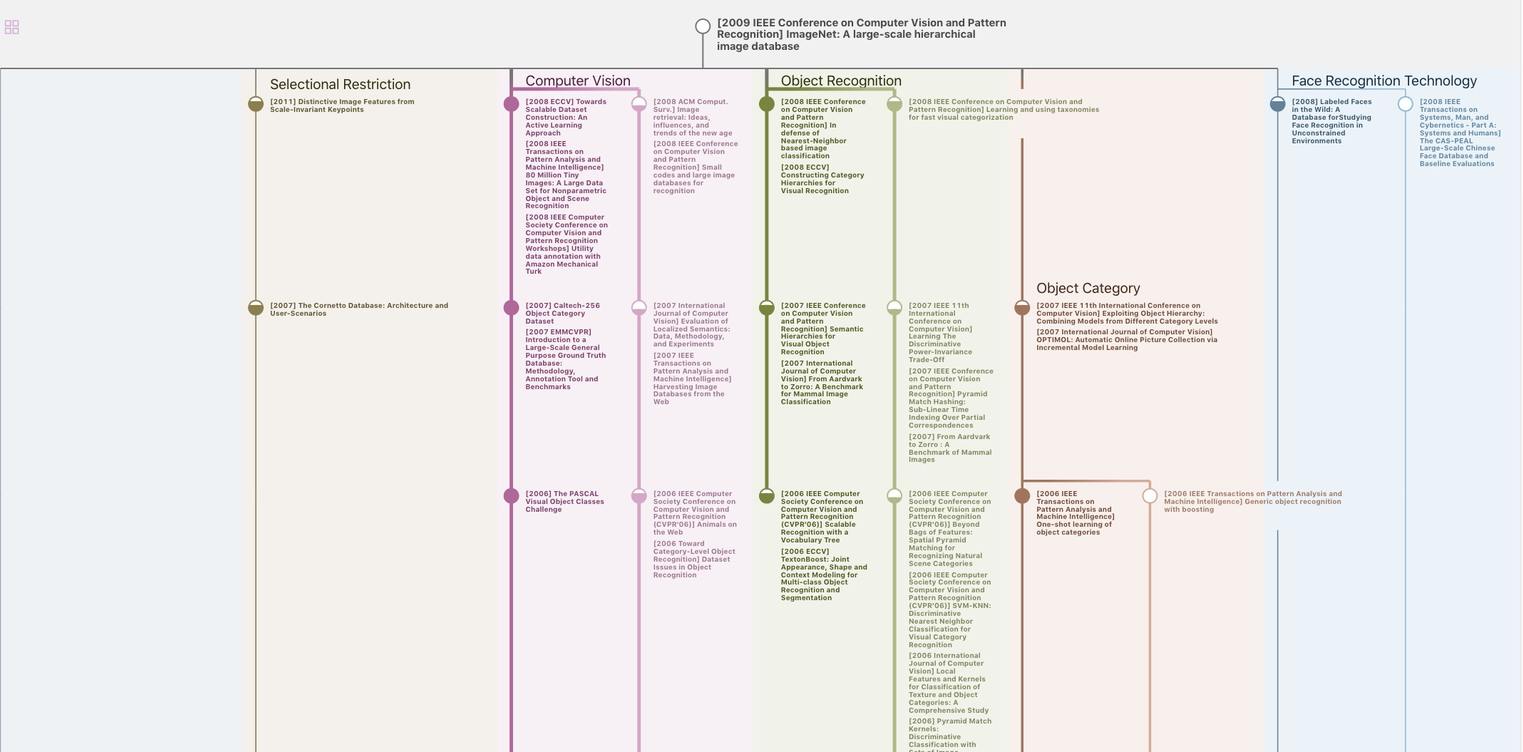
生成溯源树,研究论文发展脉络
Chat Paper
正在生成论文摘要