Using Sum-Product Networks to Assess Uncertainty in Deep Active Learning
arXiv (Cornell University)(2022)
摘要
The success of deep active learning hinges on the choice of an effective acquisition function, which ranks not yet labeled data points according to their expected informativeness. Many acquisition functions are (partly) based on the uncertainty that the current model has about the class label of a point, yet there is no generally agreed upon strategy for computing such uncertainty. This paper proposes a new and very simple approach to computing uncertainty in deep active learning with a Convolutional Neural Network (CNN). The main idea is to use the feature representation extracted by the CNN as data for training a Sum-Product Network (SPN). Since SPNs are typically used for estimating the distribution of a dataset, they are well suited to the task of estimating class probabilities that can be used directly by standard acquisition functions such as max entropy and variational ratio. The effectiveness of our method is demonstrated in an experimental study on several standard benchmark datasets for image classification, where we compare it to various state-of-the-art methods for assessing uncertainty in deep active learning.
更多查看译文
关键词
uncertainty sampling,deep neural networks,neural networks,sum-product
AI 理解论文
溯源树
样例
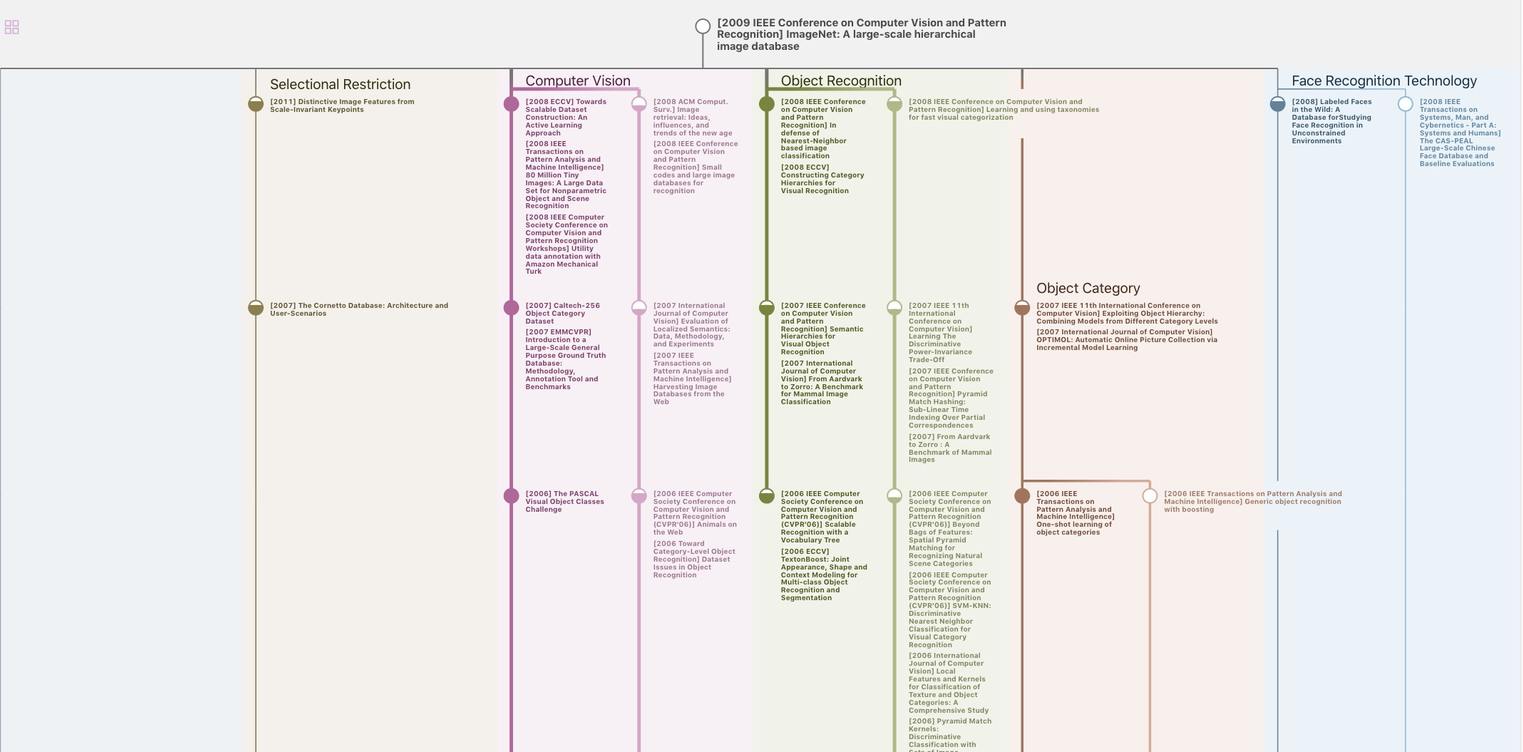
生成溯源树,研究论文发展脉络
Chat Paper
正在生成论文摘要