Self-Prompting Large Language Models for Zero-Shot Open-Domain QA
arXiv (Cornell University)(2022)
摘要
Open-Domain Question Answering (ODQA) aims at answering factoid questions without explicitly providing specific background documents. In a zero-shot setting, this task is more challenging since no data is available to train customized models like Retriever-Readers. Recently, Large Language Models (LLMs) like GPT-3 have shown their power in zero-shot ODQA with direct prompting methods, but these methods are still far from releasing the full powerfulness of LLMs only in an implicitly invoking way. In this paper, we propose a Self-Prompting framework to explicitly utilize the massive knowledge stored in the parameters of LLMs and their strong instruction understanding abilities. Concretely, we prompt LLMs step by step to generate multiple pseudo QA pairs with background passages and explanations from scratch and then use those generated elements for in-context learning. Experimental results show our method surpasses previous SOTA methods significantly on three widely-used ODQA datasets, and even achieves comparable performance with some Retriever-Reader models fine-tuned on full training data.
更多查看译文
关键词
models,language,self-prompting,zero-shot,open-domain
AI 理解论文
溯源树
样例
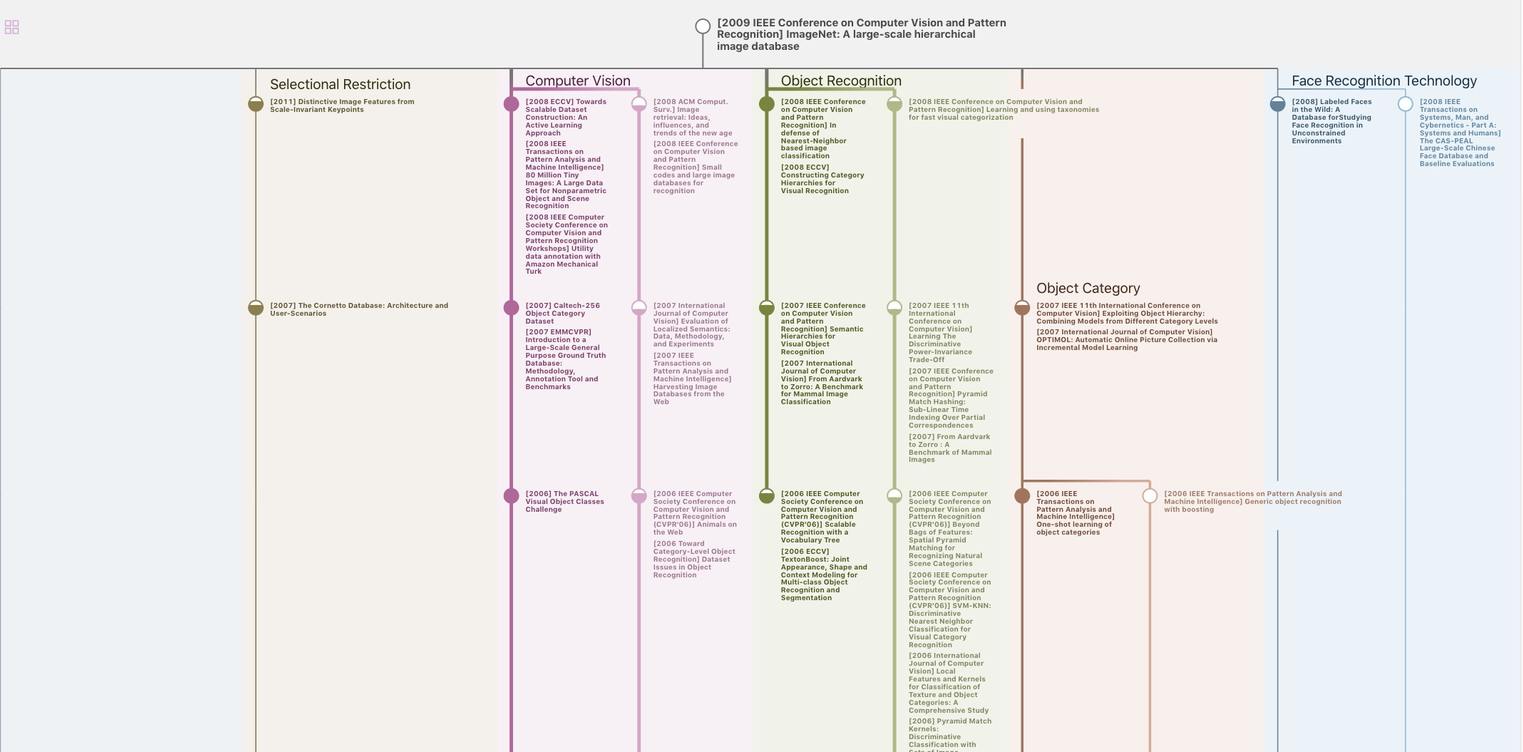
生成溯源树,研究论文发展脉络
Chat Paper
正在生成论文摘要