Early Diagnosis of Mild Cognitive Impairment Using Deep Neural Network with Single and Multi-input Analysis.
2023 IEEE 11th International Conference on Healthcare Informatics (ICHI)(2023)
摘要
Convolutional Neural Networks (CNNs) have made significant advancements in the diagnosis of hard-to-detect illnesses, such as Mild Cognitive Impairment (MCI) and its prodromal stage Alzheimer’s Disease (AD). However, identifying early MCI (EMCI) remains a challenge since the brain patterns of EMCI and Normal Cognitive (NC) individuals are quite similar. Recent studies have shown that CNNs are effective in analyzing multiple neuroimaging datasets. However, more work remains to be done on multi-input (imaging and non-imaging) CNN models. By leveraging models based on a shallow CNN and hybrid neural network, this paper investigates if the accuracy of diagnosing EMCI could be enhanced using multi-input data (e.g., structural Magnetic Resonance Imaging (sMRI), demographic data). These models have fewer parameters while retaining competitive performance on EMCI vs. NC classification problems, requiring less computational effort. Experiments were performed on a publicly available Alzheimer’s Disease Neuroimaging Initiative (ADNI) dataset with 546 subjects, including 164 EMCI and 282 NC from ADNI3 and 50 EMCI and 50 NC from ADNI2. The hybrid model achieved 87% accuracy in distinguishing between NC and EMCI groups using a coronal view of the brain.
更多查看译文
关键词
Alzheimer’s disease,early mild cognitive impairment,structural magnetic resonance imaging,convolutional neural network,multi-layer perceptron
AI 理解论文
溯源树
样例
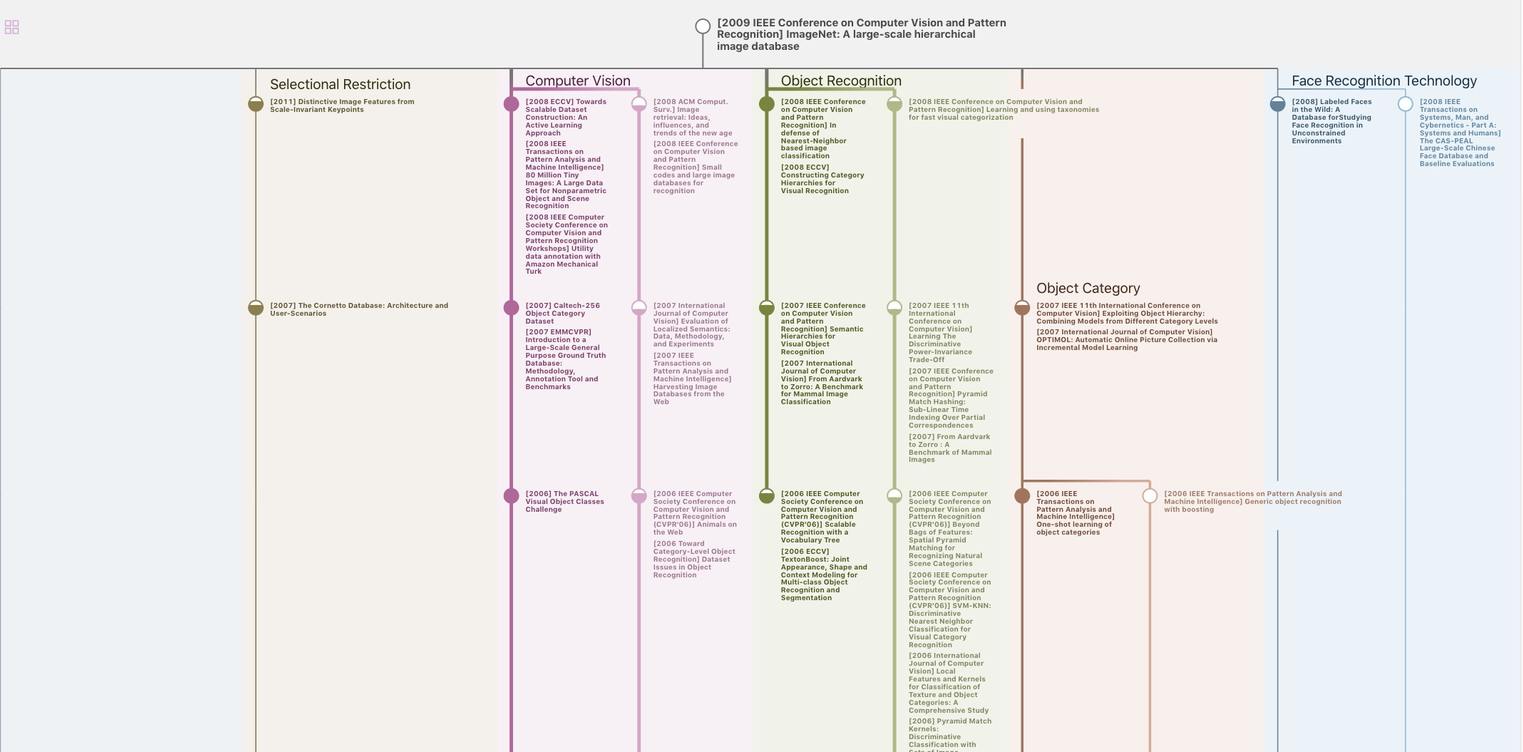
生成溯源树,研究论文发展脉络
Chat Paper
正在生成论文摘要