A Process for Evaluating Explanations for Transparent and Trustworthy AI Prediction Models.
2023 IEEE 11th International Conference on Healthcare Informatics (ICHI)(2023)
摘要
This study proposes a process to generate and validate algorithmic explanations for the reasoning of an AI prediction model, implemented using a Bayesian network (BN). The intention of the generated explanations is to increase the transparency and trustworthiness of a decision-support system that uses a BN prediction model. To achieve this, explanations should be presented in an easy-to-understand, clear, and concise natural language narrative. We have developed an algorithm for explaining the reasoning of a prediction made using a BN. For the narrative part of the explanation, we use a template which presents the ‘content’ part of the explanation; this content is a word-less information structure that applies to all BNs. The template, on the other hand, needs to be designed specifically for each BN model. In this paper, we use a BN for the risk of trauma-induced coagulopathy, a critical bleeding problem. We outline a process for using experts’ explanations as the basis for designing the explanation template. We do not believe that an algorithmic explanation needs to be indistinguishable from expert explanations; instead we aim to imitate the narrative structure of explanations given by experts, although we find that there is considerable variation in these. We then consider how the generated explanations can be evaluated, since a direct comparison (in the style of a Turing test) would likely fail. We describe a study using questionnaires and interviews to evaluate the effect of an algorithmic explanation on the transparency and also on the trustworthiness of the predictions made by the system. The preliminary results of our study suggest that the presence of an explanation makes the AI model more transparent but not necessarily more trustworthy.
更多查看译文
关键词
explainable AI,causality,risk prediction,natural language,transparency,trustworthiness
AI 理解论文
溯源树
样例
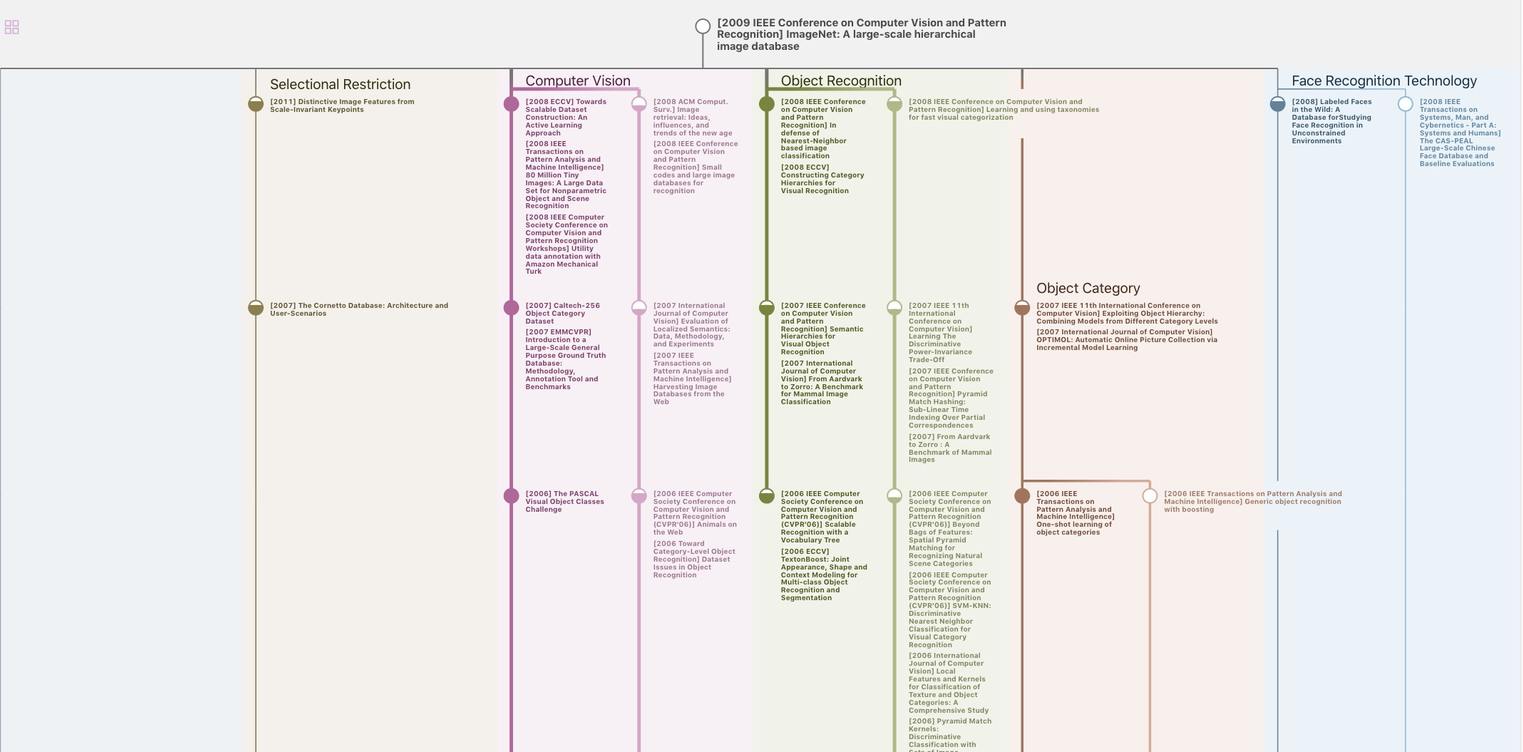
生成溯源树,研究论文发展脉络
Chat Paper
正在生成论文摘要