Enforcing Explainable Deep Few-Shot Learning to Analyze Plain Knee Radiographs: Data from the Osteoarthritis Initiative.
2023 IEEE 11th International Conference on Healthcare Informatics (ICHI)(2023)
摘要
The use of fast, accurate, and automatic knee radiography analysis is becoming increasingly important in orthopedics, and it is becoming more important in improving patient-specific diagnosis, prognosis, and treatment. Precise characterization of plain knee radiographs can greatly impact patient care, as they are usually used in preoperative and intraoperative planning. Rapid yet, deep learning medical image analysis has already shown success in a variety of knee image analysis tasks, ranging from knee joint area localization to joint space segmentation and measurement, with almost a human-like performance. However, there are several fundamental challenges that stop deep learning methods to obtain their full potential in a clinical setting such as orthopedics. These include the need for a large number of gold-standard, manually annotated training images and a lack of explainability and interpretability. To address these challenges, this study is the first to present an explainable deep few-shot learning model that can localize the knee joint area and segment the joint space in plain knee radiographs, using only a small number of manually annotated radiographs. The accuracy performance of the proposed method was thoroughly and experimentally evaluated using various image localization and segmentation measures, and it was compared to baseline models that utilized large-scale fully-annotated training datasets. The current deep few-shot learning methods achieved an average Intersection over Union (IoU) of 0.94 and a mean Average Precision @0.5 of 0.98, using 10-shot learning in the localization of the knee joint area, and an average IoU of 0.91 in the knee joint space segmentation using only 10 manually annotated radiographs.
更多查看译文
关键词
Few-shot learning,Knee joint space segmentation,Knee joint area localization,Artificial Intelligence
AI 理解论文
溯源树
样例
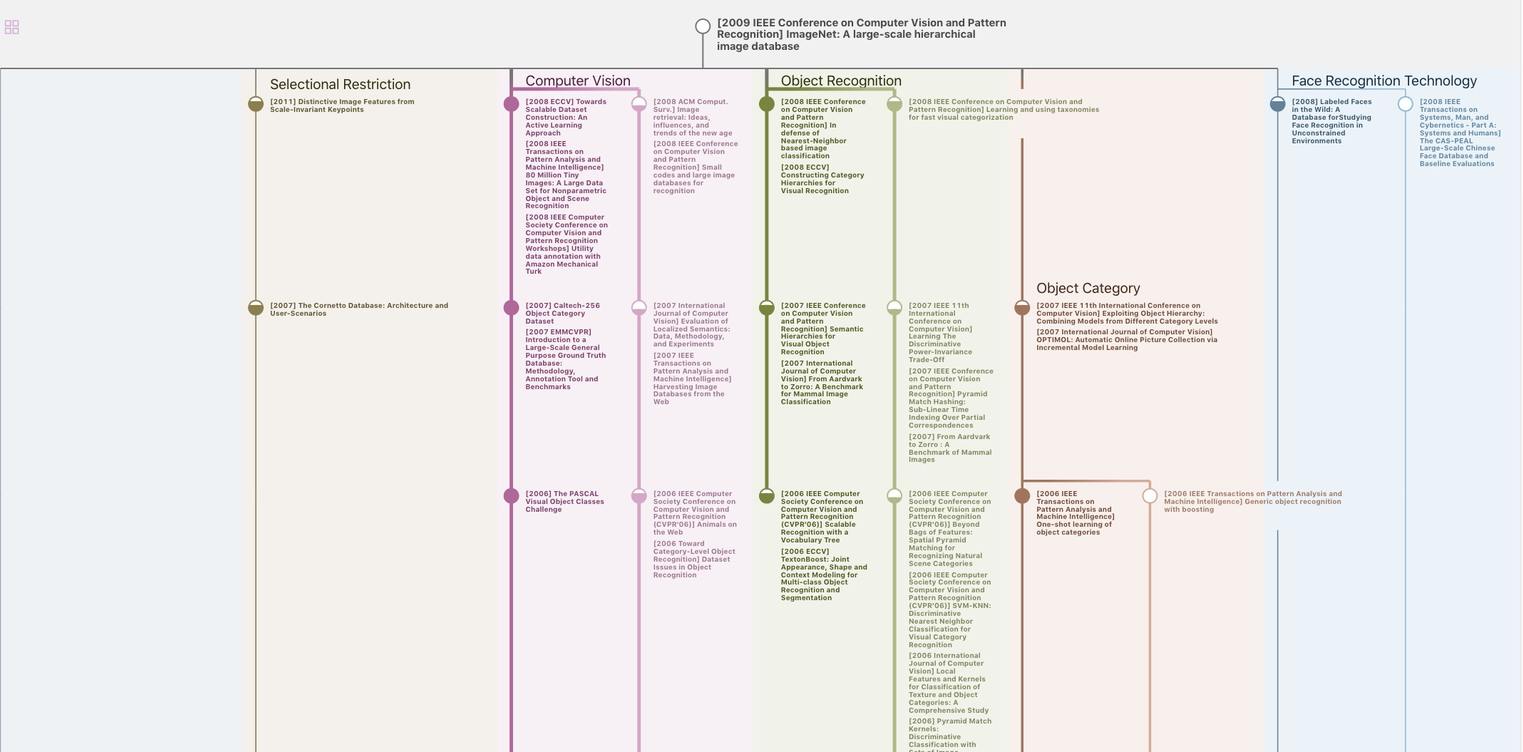
生成溯源树,研究论文发展脉络
Chat Paper
正在生成论文摘要