FPGA-aware automatic acceleration framework for vision transformer with mixed-scheme quantization
Proceedings of the 59th ACM/IEEE Design Automation Conference(2022)
摘要
Vision transformers (ViTs) are emerging with significantly improved accuracy in computer vision tasks. However, their complex architecture and enormous computation/storage demand impose urgent needs for new hardware accelerator design methodology. This work proposes an FPGA-aware automatic ViT acceleration framework based on the proposed mixed-scheme quantization. To the best of our knowledge, this is the first FPGA-based ViT acceleration framework exploring model quantization. Compared with state-of-the-art ViT quantization work (algorithmic approach only without hardware acceleration), our quantization achieves 0.31% to 1.25% higher Top-1 accuracy under the same bit-width. Compared with the 32-bit floating-point baseline FPGA accelerator, our accelerator achieves around 5.6× improvement on the frame rate (i.e., 56.4 FPS vs. 10.0 FPS) with 0.83% accuracy drop for DeiT-base.
更多查看译文
关键词
vision transformer,quantization,fpga-aware,mixed-scheme
AI 理解论文
溯源树
样例
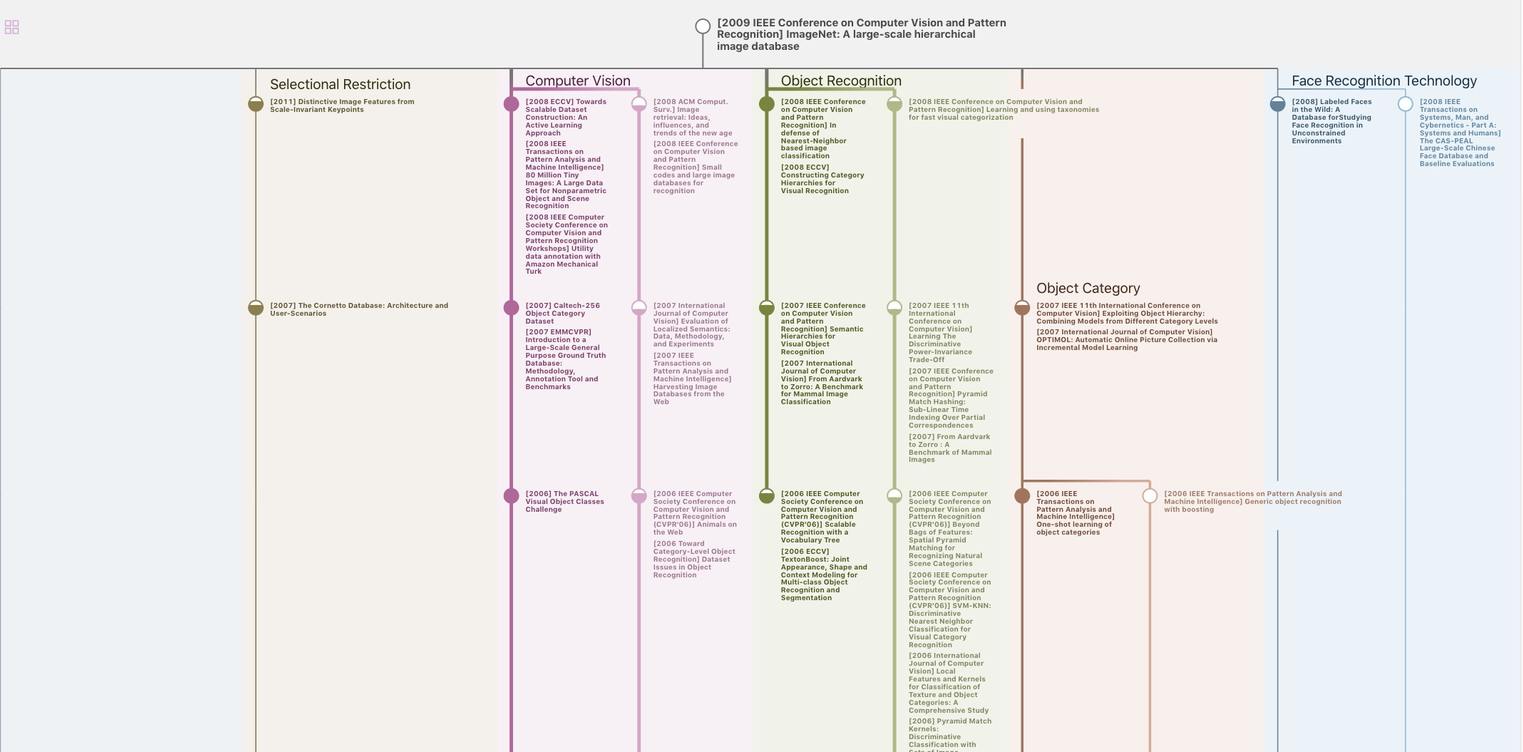
生成溯源树,研究论文发展脉络
Chat Paper
正在生成论文摘要