Causal Structure Learning from Imperfect Longitudinal Data in Healthcare.
2023 IEEE 11th International Conference on Healthcare Informatics (ICHI)(2023)
摘要
Causal Structure Discovery (CSD) is the task of learning the set of underlying causal relationships from observational data. Due to their computational scalability and flexibility, a recently developed class of CSD methods, NOTEARS, based on a formulation allowing for continuous optimization, is gaining popularity. However, this formulation can lead to incorrect edge orientations when little/no likelihood advantage is conferred upon any edge orientation, e.g., a → b → c versus c → b → a. In longitudinal data, like electronic health records (EHRs), temporal relationships are observable among many pairs of variables. Such temporal relationship is imperfect but still suggest an orientation since causal effects cannot travel backwards in time. Following this idea, we propose methods to incorporate precedence constraints into continuous optimization-based CSD methods. Experiments on both a synthetic and two real-world datasets validate the effectiveness of the proposed precedence constraints.
更多查看译文
关键词
Machine Learning,Causality,Healthcare
AI 理解论文
溯源树
样例
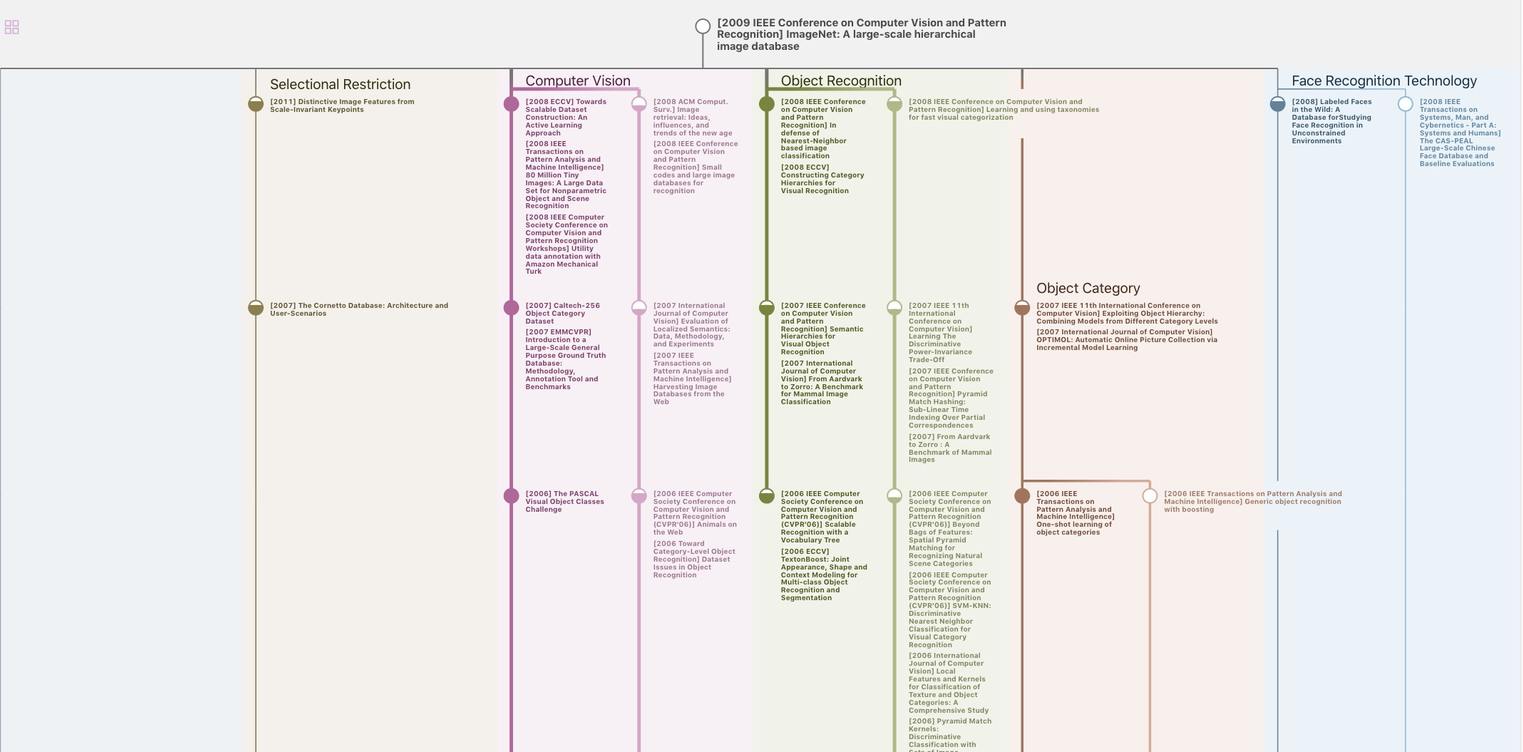
生成溯源树,研究论文发展脉络
Chat Paper
正在生成论文摘要