Conditional Generative Models for Simulation of EMG During Naturalistic Movements.
IEEE transactions on neural networks and learning systems(2024)
Abstract
Numerical models of electromyography (EMG) signals have provided a huge contribution to our fundamental understanding of human neurophysiology and remain a central pillar of motor neuroscience and the development of human-machine interfaces. However, while modern biophysical simulations based on finite element methods (FEMs) are highly accurate, they are extremely computationally expensive and thus are generally limited to modeling static systems such as isometrically contracting limbs. As a solution to this problem, we propose to use a conditional generative model to mimic the output of an advanced numerical model. To this end, we present BioMime, a conditional generative neural network trained adversarially to generate motor unit (MU) activation potential waveforms under a wide variety of volume conductor parameters. We demonstrate the ability of such a model to predictively interpolate between a much smaller number of numerical model's outputs with a high accuracy. Consequently, the computational load is dramatically reduced, which allows the rapid simulation of EMG signals during truly dynamic and naturalistic movements.
MoreTranslated text
Key words
Biophysical simulation,conditional generative model,electromyography,machine learning,motor system
AI Read Science
Must-Reading Tree
Example
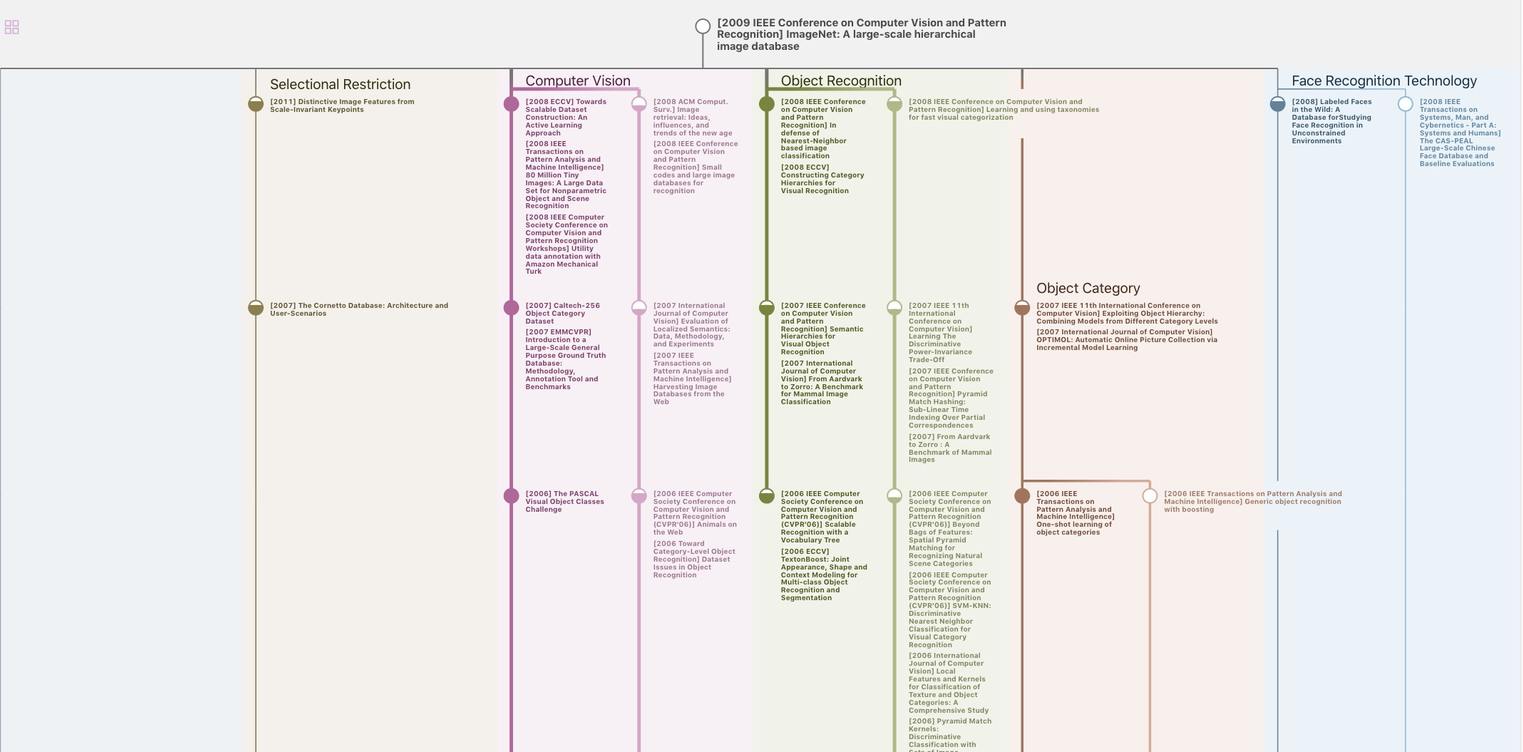
Generate MRT to find the research sequence of this paper
Chat Paper
Summary is being generated by the instructions you defined