Decision-Making Among Bounded Rational Agents
arXiv (Cornell University)(2022)
摘要
When robots share the same workspace with other intelligent agents (e.g., other robots or humans), they must be able to reason about the behaviors of their neighboring agents while accomplishing the designated tasks. In practice, frequently, agents do not exhibit absolutely rational behavior due to their limited computational resources. Thus, predicting the optimal agent behaviors is undesirable (because it demands prohibitive computational resources) and undesirable (because the prediction may be wrong). Motivated by this observation, we remove the assumption of perfectly rational agents and propose incorporating the concept of bounded rationality from an information-theoretic view into the game-theoretic framework. This allows the robots to reason other agents' sub-optimal behaviors and act accordingly under their computational constraints. Specifically, bounded rationality directly models the agent's information processing ability, which is represented as the KL-divergence between nominal and optimized stochastic policies, and the solution to the bounded-optimal policy can be obtained by an efficient importance sampling approach. Using both simulated and real-world experiments in multi-robot navigation tasks, we demonstrate that the resulting framework allows the robots to reason about different levels of rational behaviors of other agents and compute a reasonable strategy under its computational constraint.
更多查看译文
关键词
bounded rational agents,decision-making
AI 理解论文
溯源树
样例
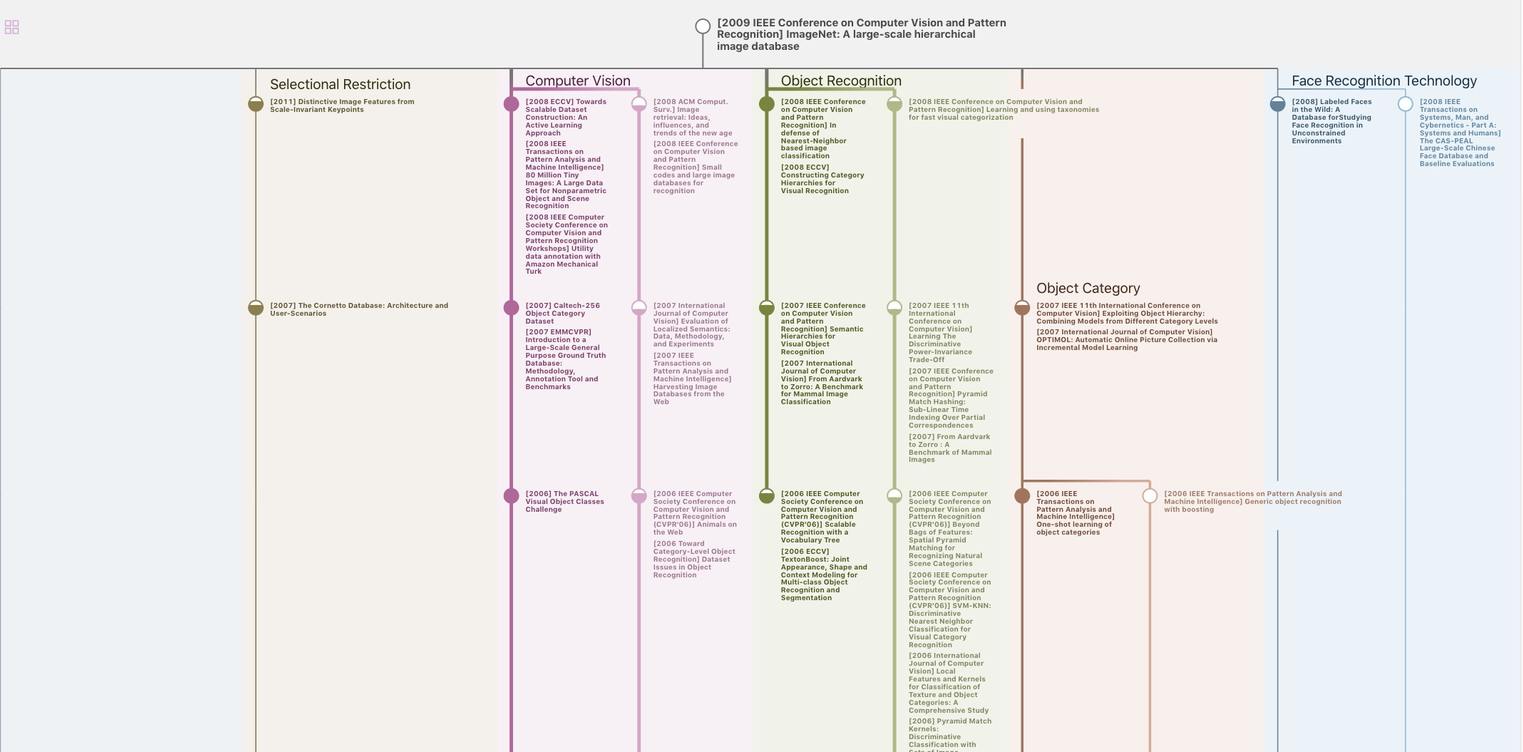
生成溯源树,研究论文发展脉络
Chat Paper
正在生成论文摘要