Transparent Shape from a Single View Polarization Image
2023 IEEE/CVF INTERNATIONAL CONFERENCE ON COMPUTER VISION (ICCV 2023)(2023)
摘要
This paper presents a learning-based method for transparent surface estimation from a single view polarization image. Existing shape from polarization(SfP) methods have the difficulty in estimating transparent shape since the inherent transmission interference heavily reduces the reliability of physics-based prior. To address this challenge, we propose the concept of physics-based prior confidence, which is inspired by the characteristic that the transmission component in the polarization image has more noise than reflection. The confidence is used to determine the contribution of the interfered physics-based prior. Then, we build a network(TransSfP) with multi-branch architecture to avoid the destruction of relationships between different hierarchical inputs. To train and test our method, we construct a dataset for transparent shape from polarization with paired polarization images and ground-truth normal maps. Extensive experiments and comparisons demonstrate the superior accuracy of our method. Our cdataset and code are publicly available at https://github.com/shaomq2187/TransSfP
更多查看译文
关键词
single view polarization image,shape
AI 理解论文
溯源树
样例
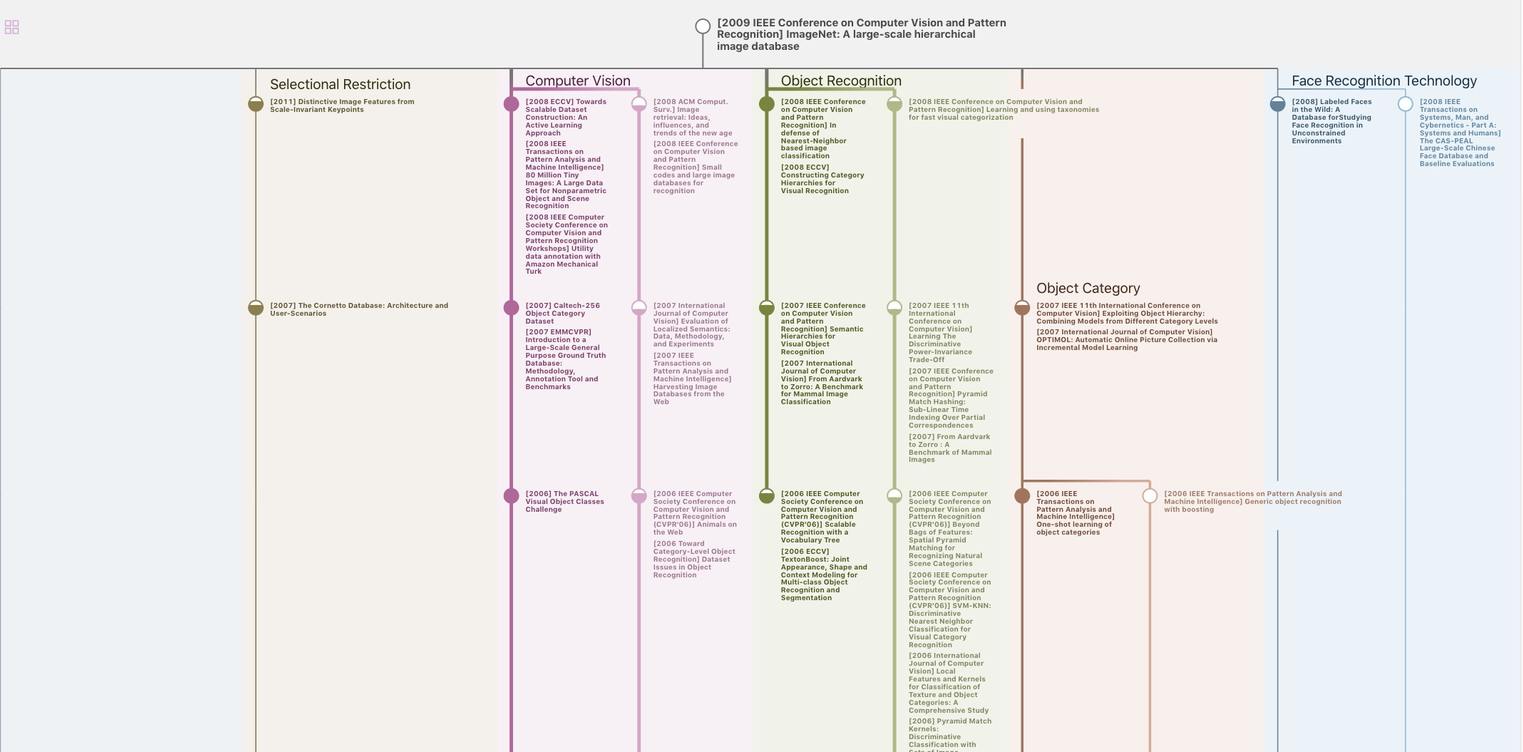
生成溯源树,研究论文发展脉络
Chat Paper
正在生成论文摘要