Hash-Based Function Call Graph Fusion Method for IoT Malware Detection
2023 26TH INTERNATIONAL SYMPOSIUM ON WIRELESS PERSONAL MULTIMEDIA COMMUNICATIONS, WPMC(2023)
摘要
The rapid growth of the Internet of Things (IoT) has brought convenience, efficiency, and significant risks, notably malware threats to IoT security. Enhancing security measures and adopting innovative detection strategies are crucial for sustainable IoT development. The Function Call Graph (FCG) is critical for classifying malicious programs. Still, reverse-engineered FCGs often have multiple functions with identical Opcode Sequences, reducing detection accuracy and potential false positives. Previous research on FCG-based malware detection has largely ignored this issue. To address this prevalent issue, the present study proposes a novel approach that leverages the distinctive characteristics of hash functions to enable one-to-one mapping and efficient searching. This method merges nodes within the FCG that share identical opcode sequences, resulting in significantly reduced training and preprocessing times while preserving the detection process's accuracy. Experimental results demonstrate a remarkable 36% reduction in training time, alongside an impressive 99.17% accuracy rate, thereby underscoring the efficacy and practicality of this proposed solution.
更多查看译文
关键词
IoT Malware,Malware Detector,Machine Learning,Deep Learning,Static Analysis,GNN
AI 理解论文
溯源树
样例
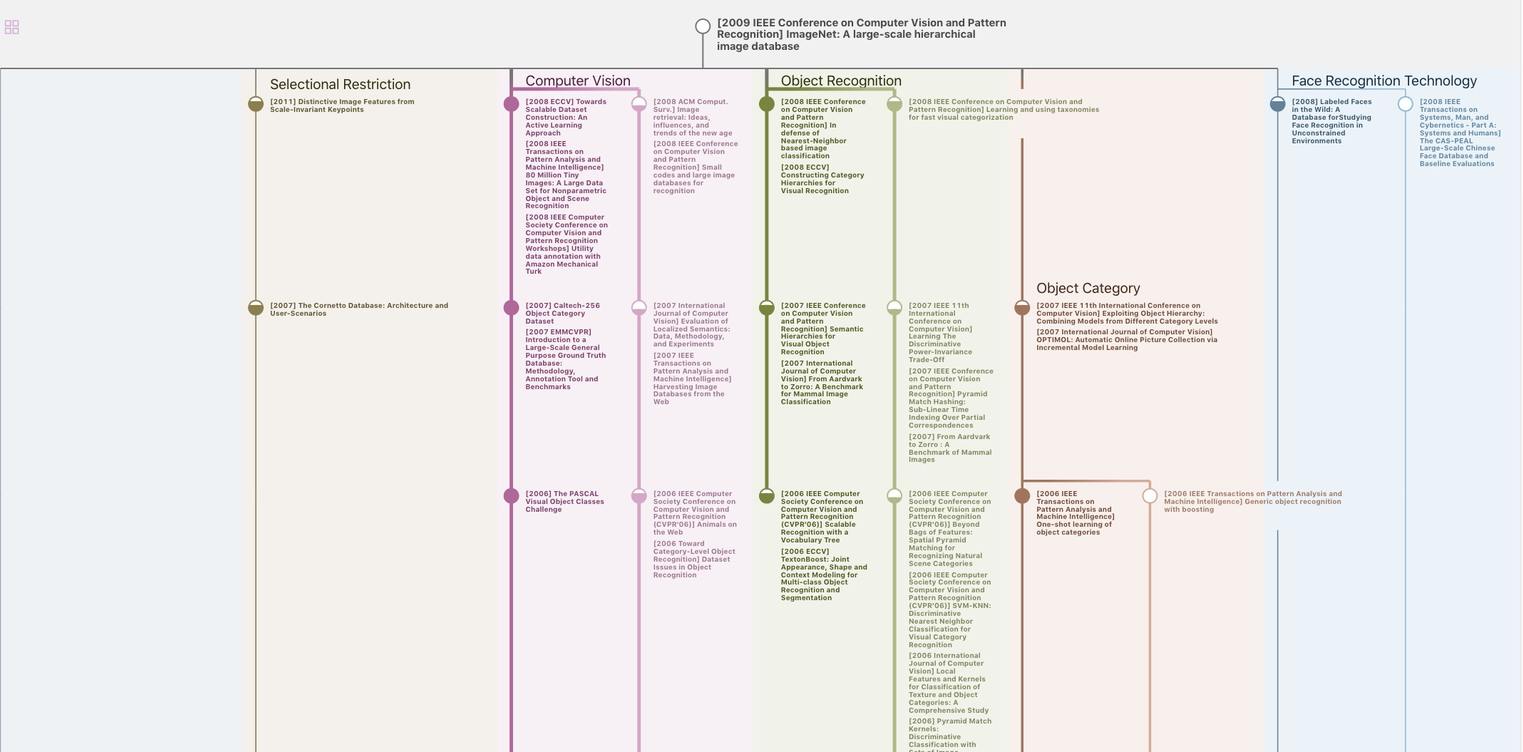
生成溯源树,研究论文发展脉络
Chat Paper
正在生成论文摘要