Self-Validated Ensemble Models for Design of Experiments
arXiv (Cornell University)(2021)
摘要
In the last twenty years, the prediction accuracy of machine learning models fit to observational data has improved dramatically. Many machine learning techniques require that the data be partitioned into at least two subsets; a training set for fitting models and a validation set for tuning models. Machine learning techniques requiring data partitioning have generally not been applied to designed experiments (DOEs), as the design structure and small run size limit the ability to withhold observations from the fitting algorithm. We introduce a newmodel-building algorithm, called self-validated ensemble models (SVEM), that emulates data partitioning by using the complete data simultaneously as both a training and a validation set. SVEM weights the two copies of the data differently under a weighting scheme based on the fractional-random-weight bootstrap (Xu et al., 2020). Similar to bagging (Breiman, 1994), this fractional-random-weight bootstrapping scheme is repeated many times and the final SVEM model is the sample average of the bootstrapped models. In this work, we investigate the performance of the SVEM algorithm with regression, Lasso, and the Dantzig Selector. However, the method is very general and can be applied in combination with most model selection and fitting algorithms. Through extensive simulations and a case study, we demonstrate that SVEM generates models with lower prediction error as compared to more traditional statistical approaches that are based on fitting a single model.
更多查看译文
关键词
models,experiments,self-validated
AI 理解论文
溯源树
样例
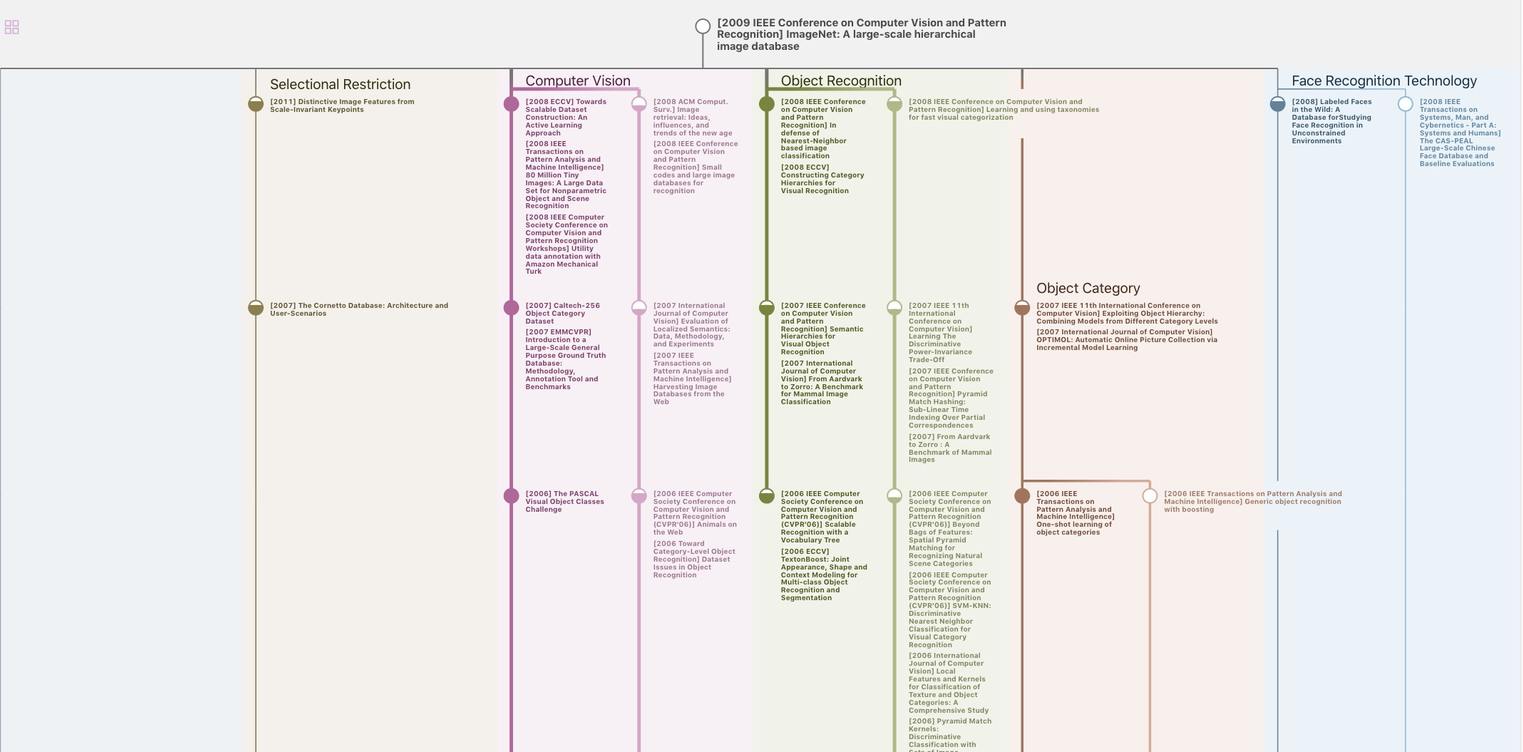
生成溯源树,研究论文发展脉络
Chat Paper
正在生成论文摘要