A contrastive rule for meta-learning
arXiv (Cornell University)(2021)
摘要
Meta-learning algorithms leverage regularities that are present on a set of tasks to speed up and improve the performance of a subsidiary learning process. Recent work on deep neural networks has shown that prior gradient-based learning of meta-parameters can greatly improve the efficiency of subsequent learning. Here, we present a biologically plausible meta-learning algorithm based on equilibrium propagation. Instead of explicitly differentiating the learning process, our contrastive meta-learning rule estimates meta-parameter gradients by executing the subsidiary process more than once. This avoids reversing the learning dynamics in time and computing second-order derivatives. In spite of this, and unlike previous first-order methods, our rule recovers an arbitrarily accurate meta-parameter update given enough compute. We establish theoretical bounds on its performance and present experiments on a set of standard benchmarks and neural network architectures.
更多查看译文
关键词
contrastive rule,meta-learning
AI 理解论文
溯源树
样例
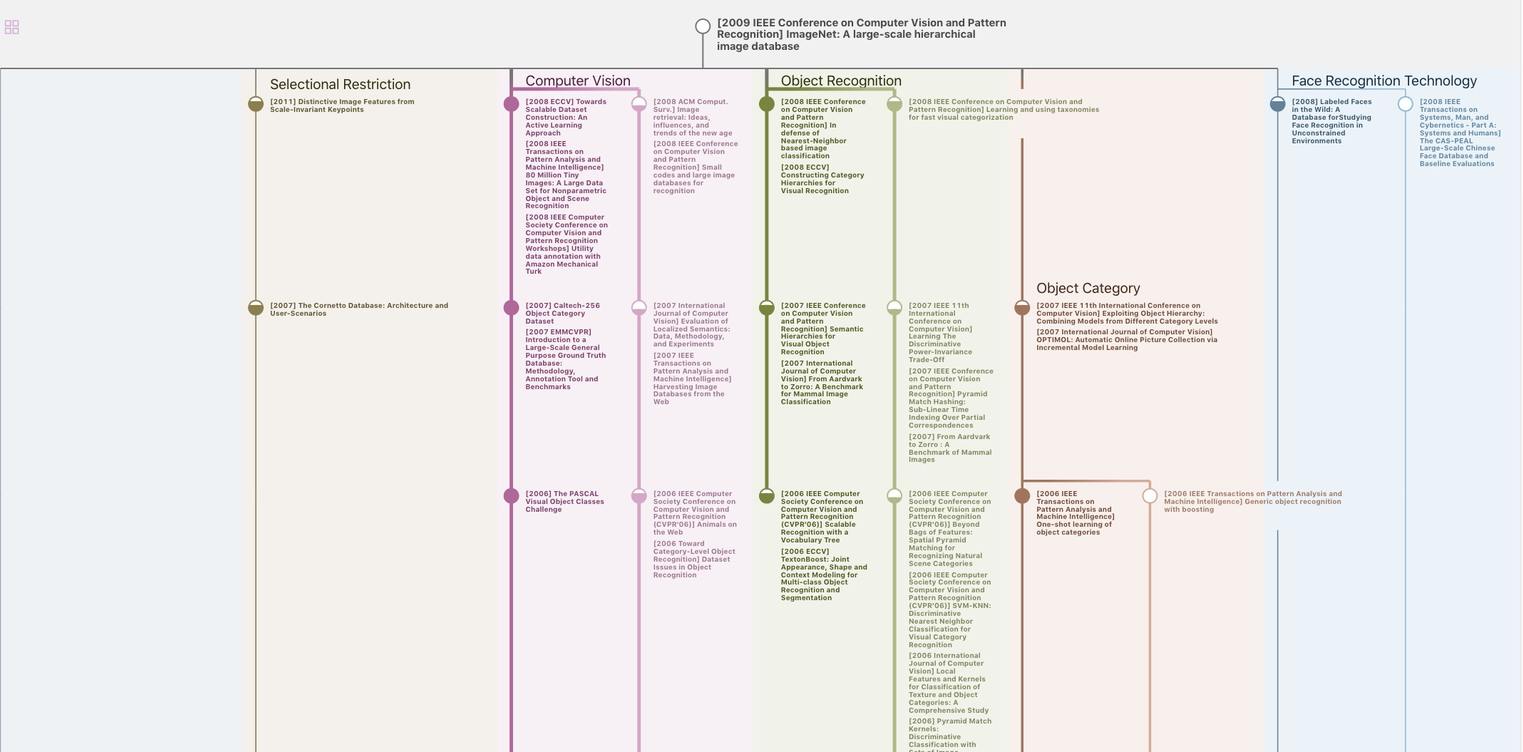
生成溯源树,研究论文发展脉络
Chat Paper
正在生成论文摘要