Reinforcement Learning for Robot Navigation with Adaptive Forward Simulation Time (AFST) in a Semi-Markov Model
2023 IEEE/RSJ INTERNATIONAL CONFERENCE ON INTELLIGENT ROBOTS AND SYSTEMS, IROS(2023)
摘要
Deep reinforcement learning (DRL) algorithms have proven effective in robot navigation, especially in unknown environments, by directly mapping perception inputs into robot control commands. However, most existing methods ignore the local minimum problem in navigation and thereby cannot handle complex unknown environments. In this paper, we propose the first DRL-based navigation method modeled by a semi-Markov decision process (SMDP) with continuous action space, named Adaptive Forward Simulation Time (AFST), to overcome this problem. Specifically, we reduce the dimensions of the action space and improve the distributed proximal policy optimization (DPPO) algorithm for the specified SMDP problem by modifying its GAE to better estimate the policy gradient in SMDPs. Experiments in various unknown environments demonstrate the effectiveness of AFST.
更多查看译文
关键词
robot navigation,reinforcement learning,adaptive forward
AI 理解论文
溯源树
样例
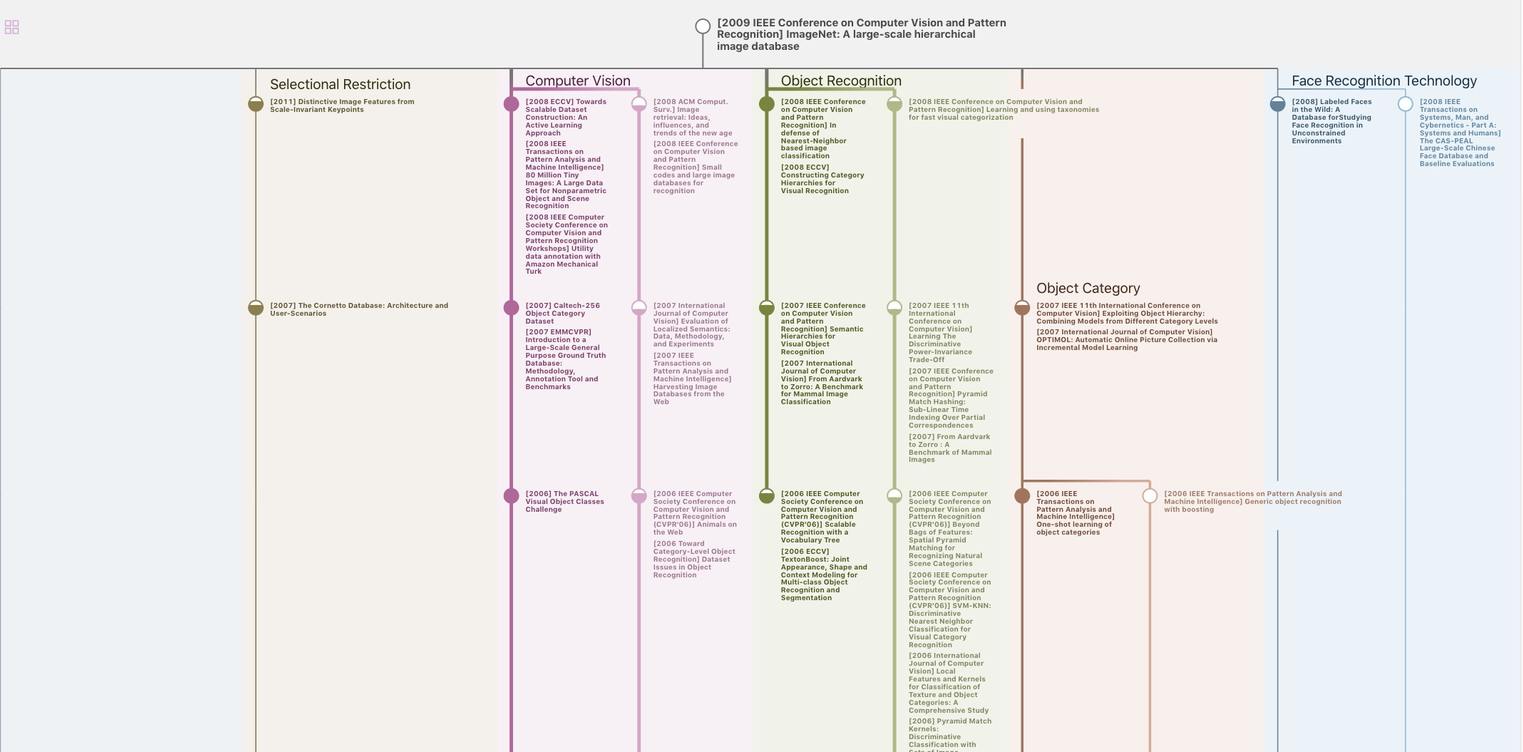
生成溯源树,研究论文发展脉络
Chat Paper
正在生成论文摘要