Unsupervised Out-of-Domain Detection via Pre-trained Transformers
arXiv (Cornell University)(2021)
摘要
Deployed real-world machine learning applications are often subject to uncontrolled and even potentially malicious inputs. Those out-of-domain inputs can lead to unpredictable outputs and sometimes catastrophic safety issues. Prior studies on out-of-domain detection require in-domain task labels and are limited to supervised classification scenarios. Our work tackles the problem of detecting out-of-domain samples with only unsupervised in-domain data. We utilize the latent representations of pre-trained transformers and propose a simple yet effective method to transform features across all layers to construct out-of-domain detectors efficiently. Two domain-specific fine-tuning approaches are further proposed to boost detection accuracy. Our empirical evaluations of related methods on two datasets validate that our method greatly improves out-of-domain detection ability in a more general scenario.
更多查看译文
关键词
detection,unsupervised,out-of-domain,pre-trained
AI 理解论文
溯源树
样例
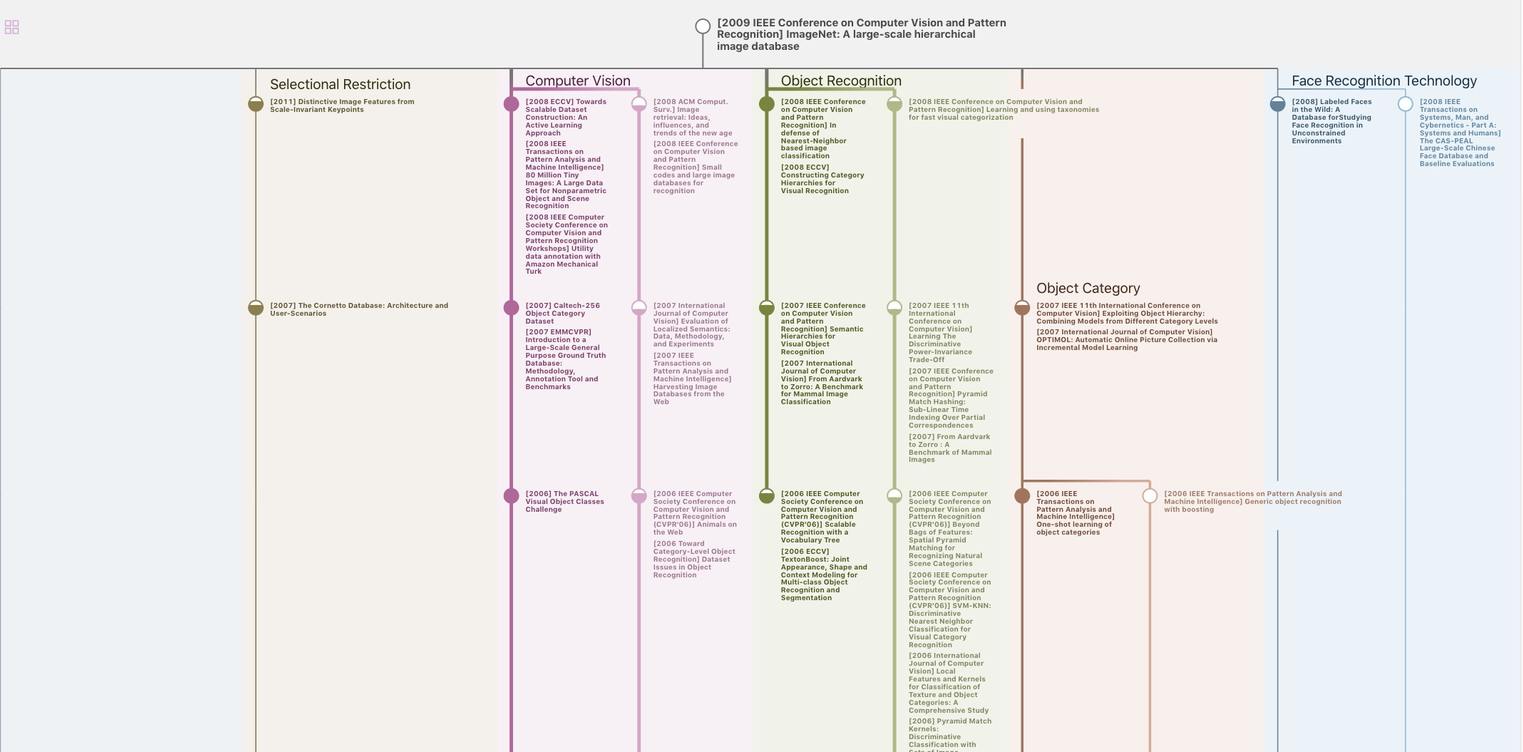
生成溯源树,研究论文发展脉络
Chat Paper
正在生成论文摘要