A low-sample-count, high-precision Pareto front adaptive sampling algorithm based on multi-criteria and Voronoi
Soft Computing(2023)
摘要
In this paper, a Pareto front (PF)-based sampling algorithm, PF-Voronoi sampling method, is proposed to solve computationally intensive multi-objective problems of medium size. The Voronoi diagram is introduced to classify the region containing PF prediction points into Pareto front cells (PFCs). Valid PFCs are screened according to the maximum crowding criterion (MCC), Maximum leave-one-out error criterion (MLEC), and maximum mean mean-square-error (MSE) criterion (MMMSEC). Sampling points are selected among the valid PFCs based on the Euclidean distance. The PF-Voronoi sampling method is applied to the coupled Kriging and NSGA-II models, and its validity is verified on the ZDT mathematical cases. The results show that the MCC criterion helps to improve the distribution diversity of PF. The MLEC criterion and the MMMSEC criterion reduce the number of training samples by 38.9
更多查看译文
关键词
adaptive,algorithm,low-sample-count,high-precision,multi-criteria
AI 理解论文
溯源树
样例
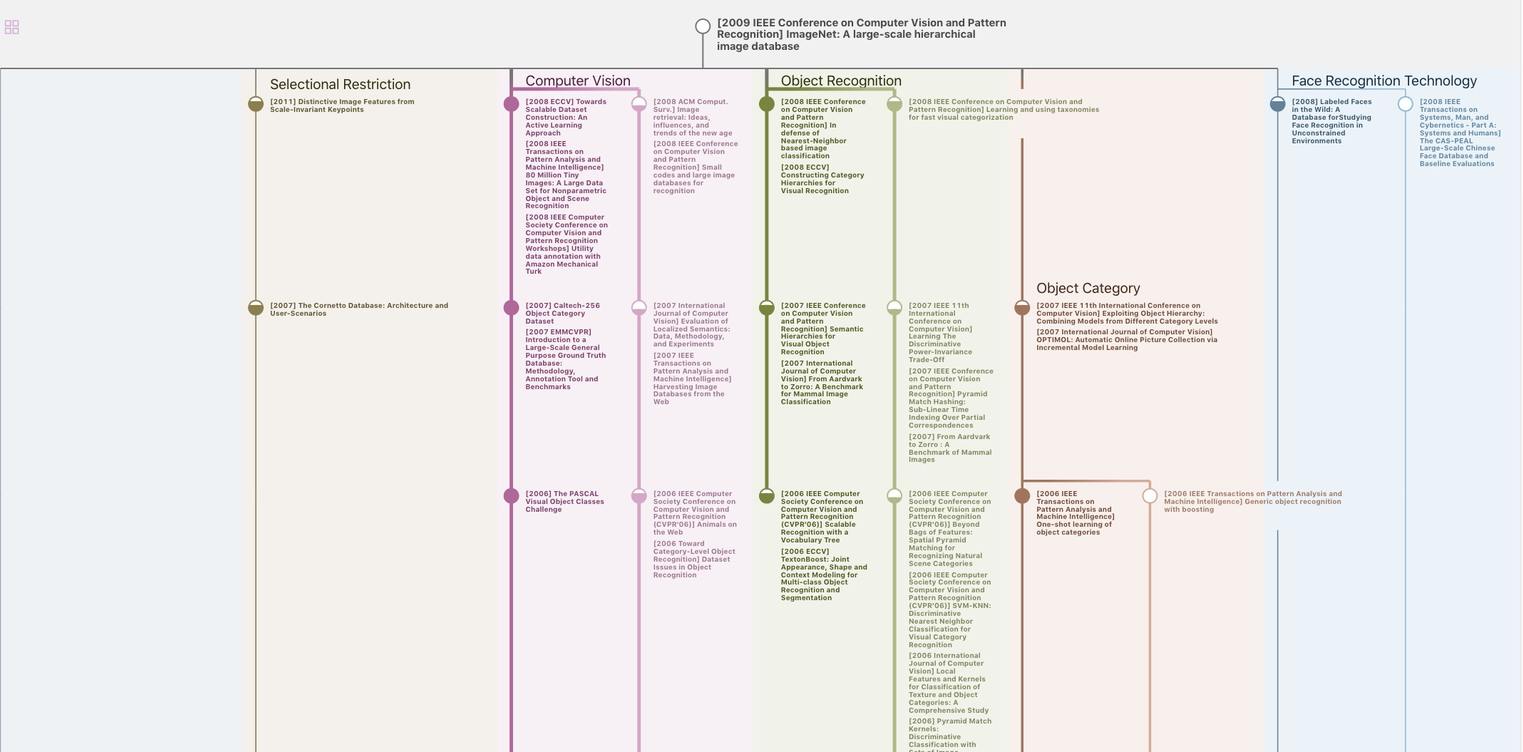
生成溯源树,研究论文发展脉络
Chat Paper
正在生成论文摘要